Ozgur Erkent: robotic rescue operations with Duckietown
Ankara, Turkey, January 2025: Prof. Ozgur Erkent shares how Duckietown is shaping robotics education at Hacettepe University. From hands-on learning in his Introduction to Robotics course, to real-world applications in rescue operations, he explains why he believes Duckietown is an invaluable tool for students exploring autonomous systems.
Bringing hands-on robotics to the classroom
At Hacettepe University, Professor Ozgur Erkent is using Duckietown in his curriculum and providing students with hands-on learning experiences that bridge theory and real-world applications.
Good morning and welcome! Could you introduce yourself and your work?
My name is Ozgur Erkent and I am an Assistant Professor at Hacettepe University’s Computer Engineering Department. I have been here for nearly three years, focusing on mobile robots and autonomous vehicles. My work involves both teaching and research in these areas.
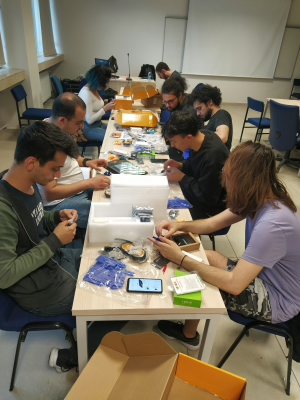
How did you first discover Duckietown?
What course do you use Duckietown for, and what does it involve?
I also wrote a project funded by NVIDIA through the “Bridge To Turkiye Fund”, that focuses on rescue robotics. After the devastating earthquake in Turkey two years ago, NVIDIA launched an initiative to support research aimed at disaster response. With NVIDIA as the sponsor, we were able to purchase the Duckiebots, Duckiedrones and related tools for the Robotics Lab course. I proposed a project that leverages Duckietown kits to train students in SLAM (Simultaneous Localization and Mapping), sensor integration, and autonomous navigation—key skills for robotics applications in search and rescue operations. Through this project, students may gain hands-on experience in developing robotic systems that could one day assist in real-world disaster relief efforts.
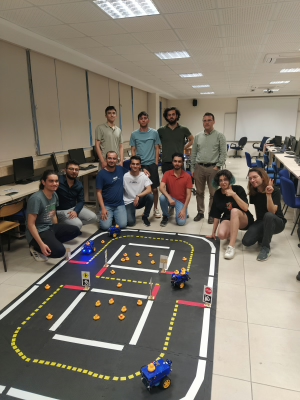
Robotics is more than just algorithms; it’s about solving real-world challenges. Duckietown helps students bridge that gap in a meaningful way.
Ozgur Erkent
How have students reacted to working with Duckietown?
Many students come from a software background, so working with real hardware is a new challenge. Some find it difficult at first, but those who enjoy hands-on work really thrive. They even help their peers with assembly and troubleshooting. It’s a valuable learning experience. If I were to design something for undergraduate students learning robotics, it would probably look a lot like Duckietown. I think it would be a great addition, as it would help students get hands-on experience with the basics of robotics.
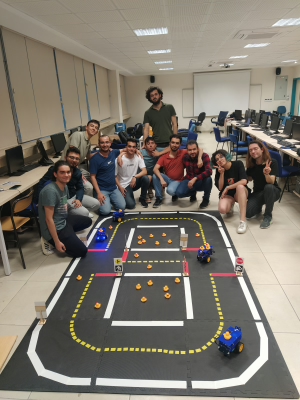
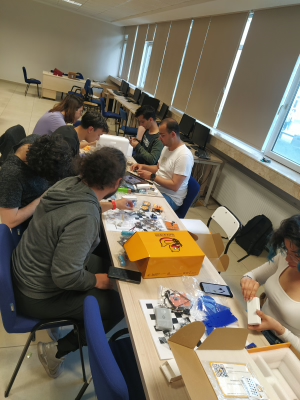
If I were to design something for undergraduate students learning robotics, it would probably look a lot like Duckietown. I think it would be a great addition, as it would help students get hands-on experience with the basics of robotics.
Ozgur Erkent
Besides Duckiebots, are you using any other tools?
Yes, I have also introduced Duckiedrones, which are especially popular in Turkey. The national foundation supports drone projects, and students are eager to explore them. Several groups are already working on Duckiedrone-based initiatives.
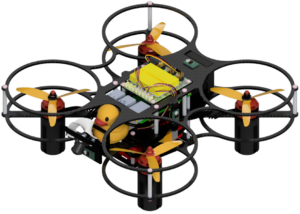
What do you think about the Duckietown community and support?
Any final thoughts?
Learn more about Duckietown
Duckietown enables state-of-the-art robotics and AI learning experiences.
It is designed to help teach, learn, and do research: from exploring the fundamentals of computer science and automation to pushing the boundaries of human knowledge.
Tell us your story
Are you an instructor, learner, researcher or professional with a Duckietown story to tell?
Reach out to us!