General Information
- Title: Learning to Drive with Reinforcement Learning and Variational Autoencoders
- Authors: Bryon Kucharski
- Institution: University of Massachusetts Amherst, United States
- Citation: Kucharski, B., Learning to Drive with Reinforcement Learning and Variational Autoencoders.
Variational Autoencoder for autonomous driving in Duckietown
This project explored using reinforcement learning (RL) and Variational Autoencoder (VAE) to train an autonomous agent for lane following in the Duckietown Gym simulator. VAEs were used to encode high-dimensional raw images into a low-dimensional latent space, reducing the complexity of the input for the RL algorithm (Deep Deterministic Policy Gradient, DDPG). The goal was to evaluate if this dimensionality reduction improved training efficiency and agent performance.
The agent successfully learned to follow straight lanes using both raw images and VAE-encoded representations. However, training with raw images performed similarly to VAEs, likely because the task was simple and had limited variability in road configurations.
The agent also displayed discrete control behaviors, such as extreme steering, in a task requiring continuous actions. These issues were attributed to the network architecture and limited reward function design.
While the VAE reduced training time slightly, it did not significantly improve performance. The project highlighted the complexity of RL applications, emphasizing the need for robust reward functions and network designs.
Highlights - Variational Autoencoder and RL for Duckietown Lane Following
Here is a visual tour of the work of the authors. For all the details, check out the full paper.
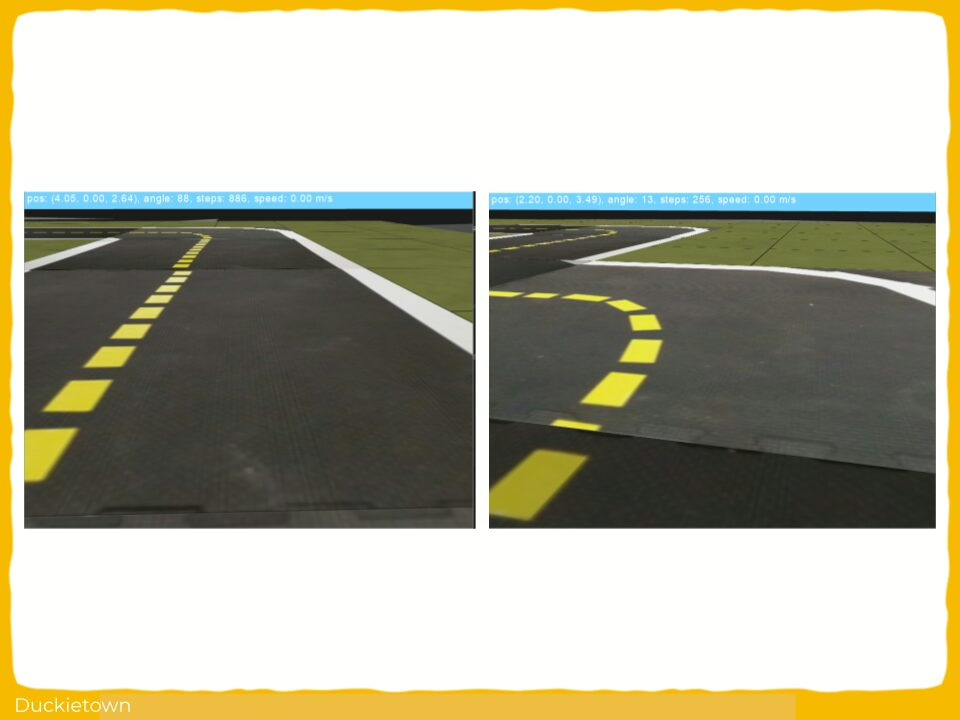
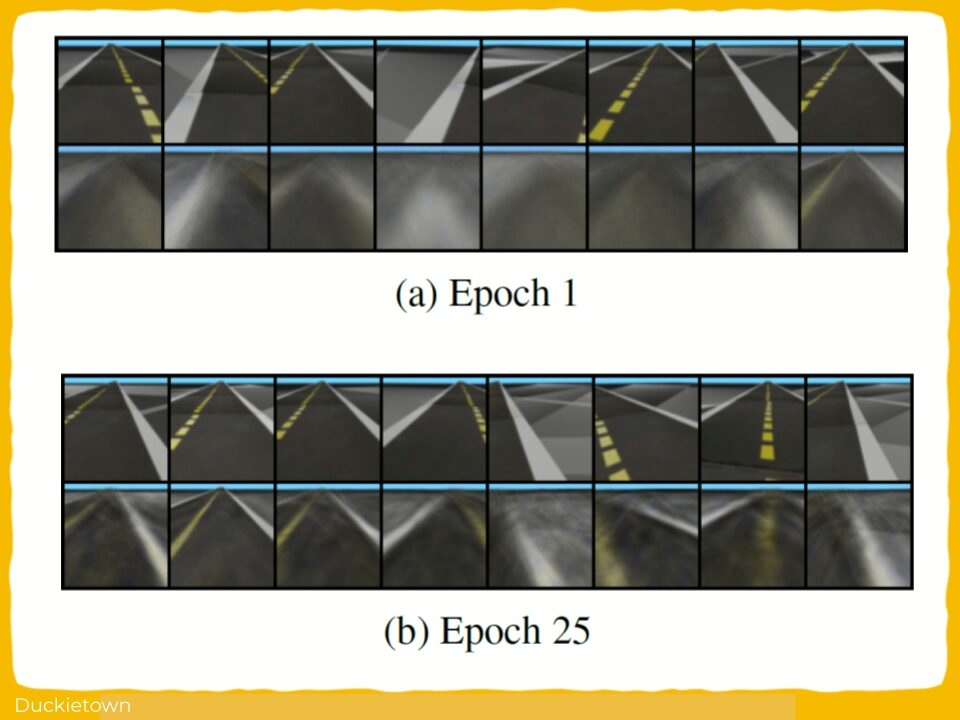
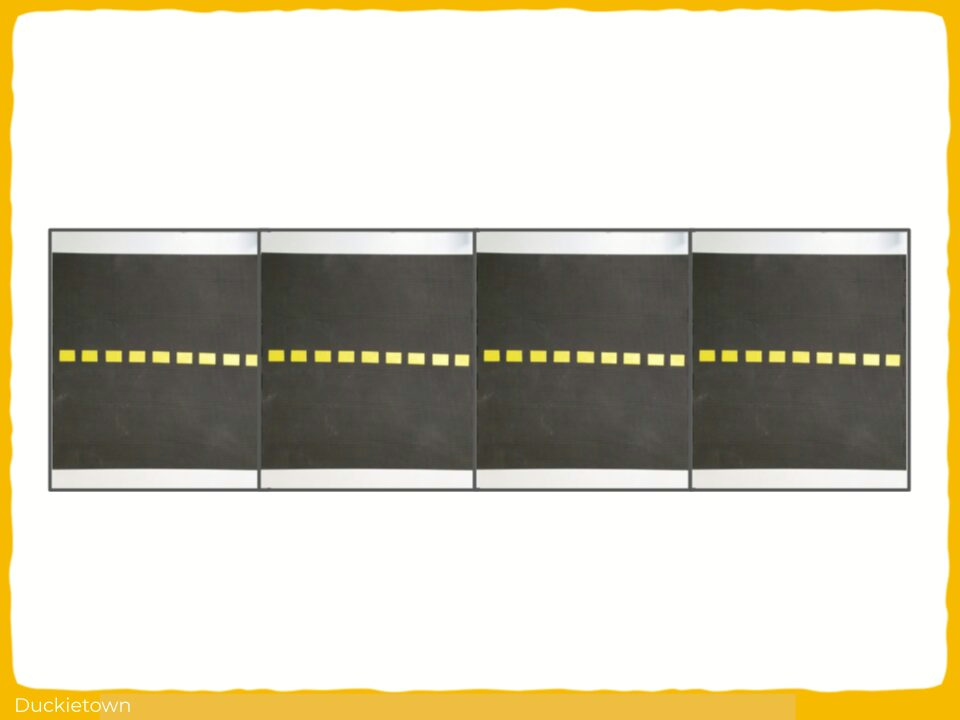
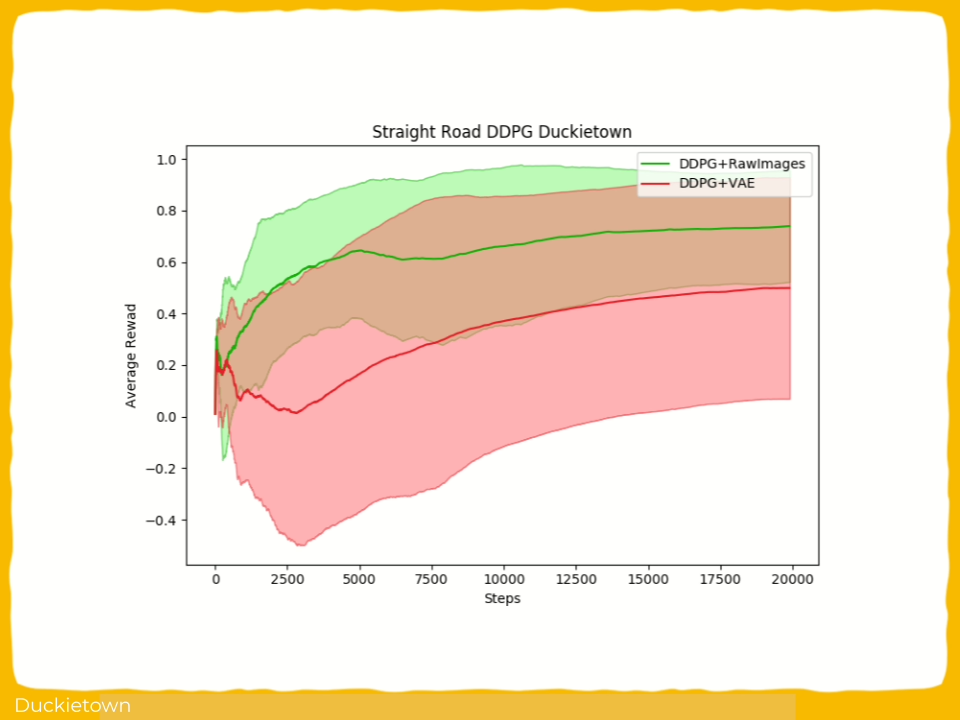
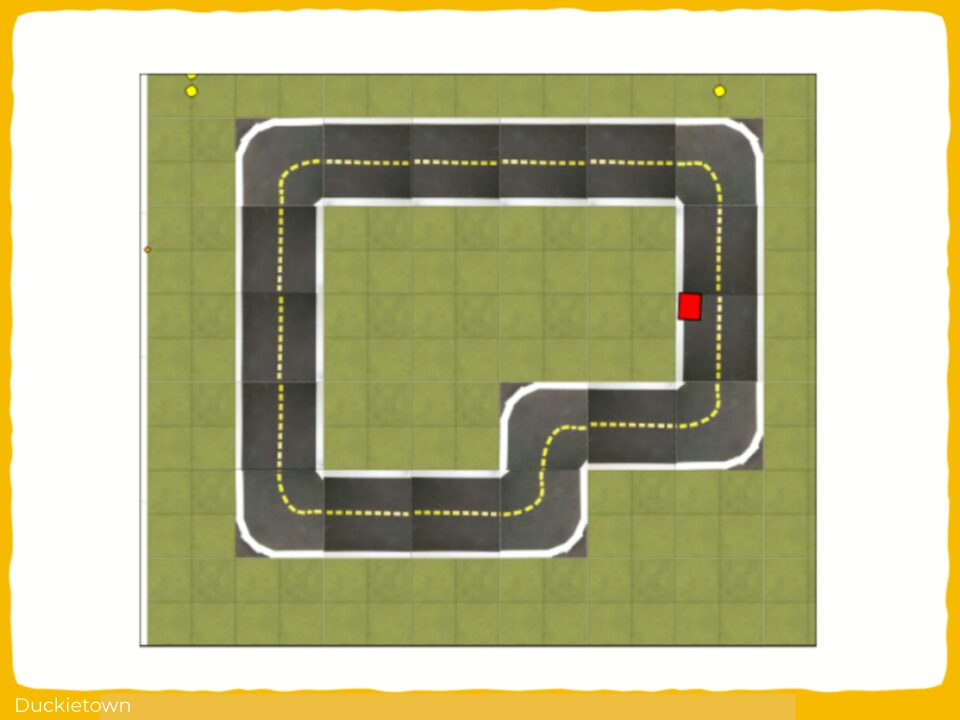
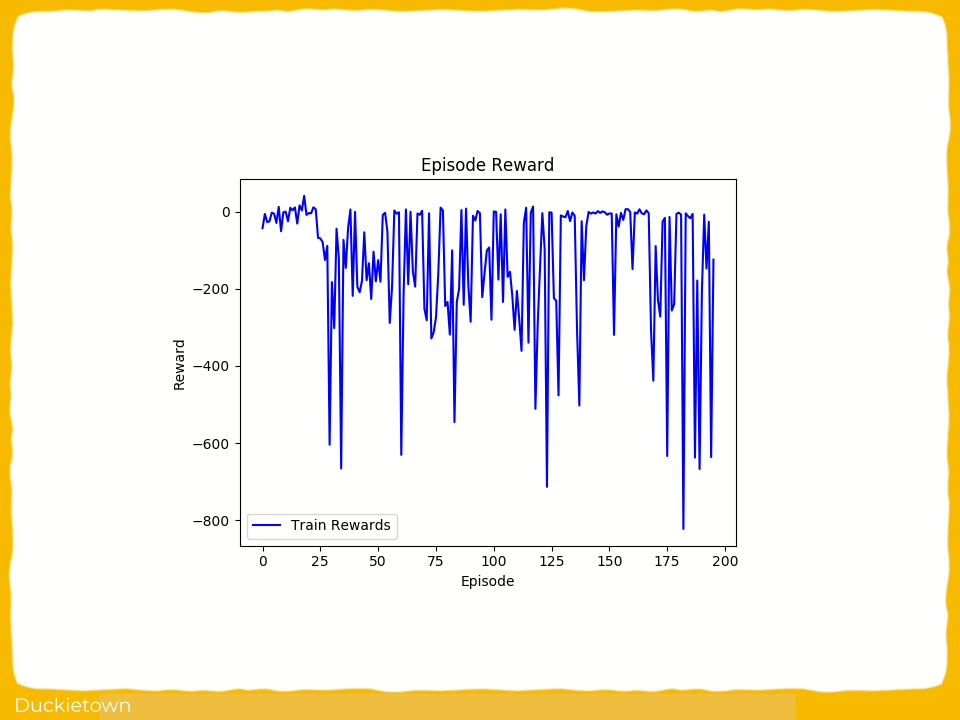
Abstract
In the author’s words:
The use of deep reinforcement learning (RL) for following the center of a lane has been studied for this project. Lane following with RL is a push towards general artificial intelligence (AI) which eliminates the use for hand crafted rules, features, and sensors.
A project called Duckietown has created the Artificial Intelligence Driving Olympics, which aims to promote AI education and embodied AI tasks. The AIDO team has released an open-sourced simulator which was used as an environment for this study. This approach uses the Deep Deterministic Policy Gradient (DDPG) with raw images as input to learn a policy for driving in the middle of a lane for two experiments. A comparison was also done with using an encoded version of the state as input using a Variational Autoencoder (VAE) on one experiment.
A variety of reward functions were tested to achieve the desired behavior of the agent. The agent was able to learn how to drive in a straight line, but was unable to learn how to drive on curves. It was shown that the VAE did not perform better than the raw image variant for driving in the straight line for these experiments. Further exploration of reward functions should be considered for optimal results and other improvements are suggested in the concluding statements.
Conclusion - Variational Autoencoder and RL for Duckietown Lane Following
Here are the conclusions from the author of this paper:
“After the completion of this project, I have gained insight on how difficult it is to get RL applications to work well. Most of my time was spent trying to tune the reward function. I have a list of improvements that are suggested as future work.
- Different network architectures – I used fully connected networks for all the architectures. I would think CNN architectures may be better at creating features for state representations.
- Tuning Networks – Since most of my time was spent on the reward exploration, I did not change any parameters at all. I followed the paper in the original DDPG paper [4]. A hyperparameter search may prove to be beneficial to find parameters that work best for my problem instead of all the problems in the paper.
- More training images for VAE
- Different Algorithm – Maybe an algorithm like PPO may be able to learn a better policy?
- Linear Function Approximation – Deep reinforcement learning has proven to be difficult to tune and work well. Maybe I could receive similar or better results using a different function approximator than a neural network. Wayve explains the use of prioritized experience replay [7], which is a method to improve on randomly sampled tuples of experiences during RL training and is based on sorting the tuples. This may improve performance of both of my algorithms.
- Exploring different Ornstein-Uhlenbeck process parameters to encourage, discourage more/less exploration
- Other dimensionality reducing methods instead of VAE. Maybe something like PCA?
As for the AIDO competition, I have made the decision not to submit this work. It became apparent to me as I progressed through the project how difficult it is to get a perfectly working model using reinforcement learning. If I was to continue with this work for the submission, I think I would rather go towards the track of imitation learning. While this would introduce a wide range of new problems, I think intuitively it moves more sense to ”show” the robot how it should drive on the road rather having it learn from scratch. I even think classical control methods may work better or just as good as any machine learning based algorithm. Although I will not submit to this competition, I am glad I got to express two interests of mine in reinforcement learning and variational autoencoders.
The supplementary documents for this report include the training set for the VAE, a video of experiment 1 working properly for both DDPG+Raw and DDPG+VAE, and a video of experiment 2 not working properly. The code has been posted to GitHub (Click for link).”
Project Authors
Bryon Kucharski is currently working as a Lead Data Scientist at Gartner, United States.
Learn more
Duckietown is a platform for creating and disseminating robotics and AI learning experiences.
It is modular, customizable and state-of-the-art, and designed to teach, learn, and do research. From exploring the fundamentals of computer science and automation to pushing the boundaries of knowledge, Duckietown evolves with the skills of the user.