General Information
- Towards Autonomous Driving with Small-Scale Cars: A Survey of Recent Development
- Dianzhao Li, Paul Auerbach, Ostap Okhrin
- Technische Universität Dresden, Germany
Towards Autonomous Driving with Small-Scale Cars: A Survey of Recent Development
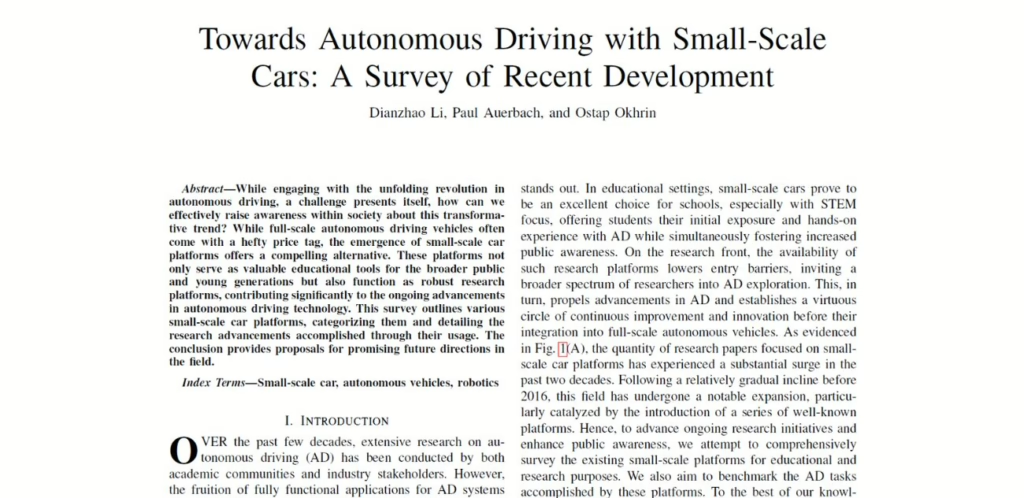
“Towards Autonomous Driving with Small-Scale Cars: A Survey of Recent Development by Dianzhao Li, Paul Auerbach, and Ostap Okhrin is a review that highlights the rapid development of the industry and the important contributions of small-scale car platforms to robot autonomy research.
This survey is a valuable resource for anyone looking to get their bearings in the landscape of autonomous driving research.
We are glad see Duckietown – not only included on the list – but identified as one of the platforms that started a marked increase in the trend of yearly published papers.
The mission of Duckietown, since we started at as a class at MIT, is to democratize access to the science and technology of robot autonomy. Part of how we intended to achieve this mission was to streamline the way autonomous behaviors for non-trivial robots were developed, tested and deployed in the real world.
From 2018-2021 we ran several editions of the AI Driving Olympics (AI-DO): an international competition to benchmark the state of the art of embodied AI for safety-critical applications. It was a great experience – not only because it led to the development of the Challenges infrastructure, the Autolab infrastructure, and many agent baselines that catalyze further developments that are now available to the broader community, but even because it was the first time physical robots were brought the world’s leading scientific conference in Machine Learning (NeurIPS: the Neural Information Processing Systems conference – known as NIPS the first time AI-DO was launched).
All this infrastructure development and testing might have been instrumental in making R&D in autonomous mobile robotics more efficient. Practitioners in the field know-how doing R&D is particularly difficult because final outcomes are the result of the tuple (robot) x (environment) x (task) – so not standardizing everything other than the specific feature under development (i.e., not following the ceteris paribus principle) often leads to apples and pair comparisons, i.e., bad science, which hampers the overall progress of the field.
We are happy to see Duckietown recognized as a contributor to facilitating the making of good science in the field. We beleive that even better and more science will come in the next years, as the students being educated with the Duckietown system start their professional journeys in academia or the workforce.
We are excited to see what the future of robot autonomy will look like, and we will continue doing our best by providing tools, workflows, and comprehensive resources to facilitate the professional development of the next generations of scientists, engineers, and practicioners in the field!
To learn more about Duckietown teaching resources follow the link below.
Starting around 2016, with the introduction of Duckietown, BARC, and Autorally, there was a significant increase in research papers.
Dianzhao Li, Paul Auerbach, Ostap Okhrin
Abstract
We report the abstract of the authors’ work:
“While engaging with the unfolding revolution in autonomous driving, a challenge presents itself, how can we effectively raise awareness within society about this transformative trend? While full-scale autonomous driving vehicles often come with a hefty price tag, the emergence of small-scale car platforms offers a compelling alternative.
These platforms not only serve as valuable educational tools for the broader public and young generations but also function as robust research platforms, contributing significantly to the ongoing advancements in autonomous driving technology.
This survey outlines various small-scale car platforms, categorizing them and detailing the research advancements accomplished through their usage. The conclusion provides proposals for promising future directions in the field.”
Towards Autonomous Driving with Small-Scale Cars: A Survey of Recent Development
Here is a visual tour of the work. For more details, check out the full paper.
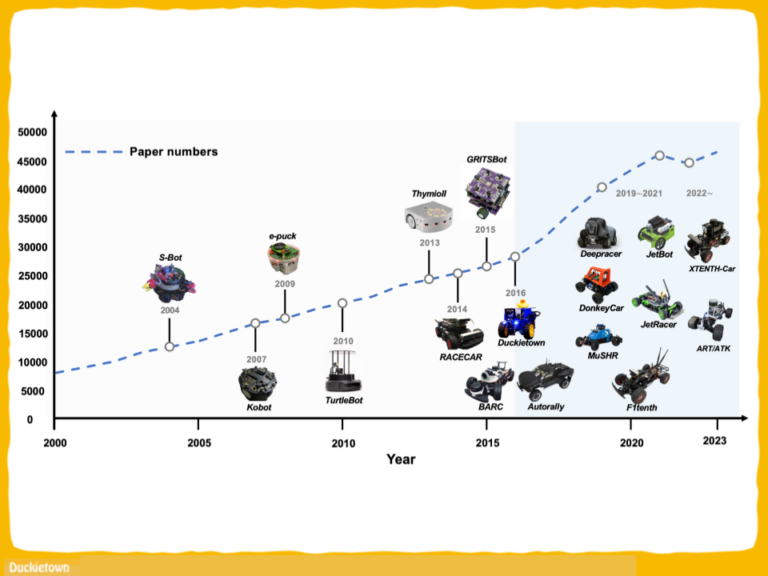
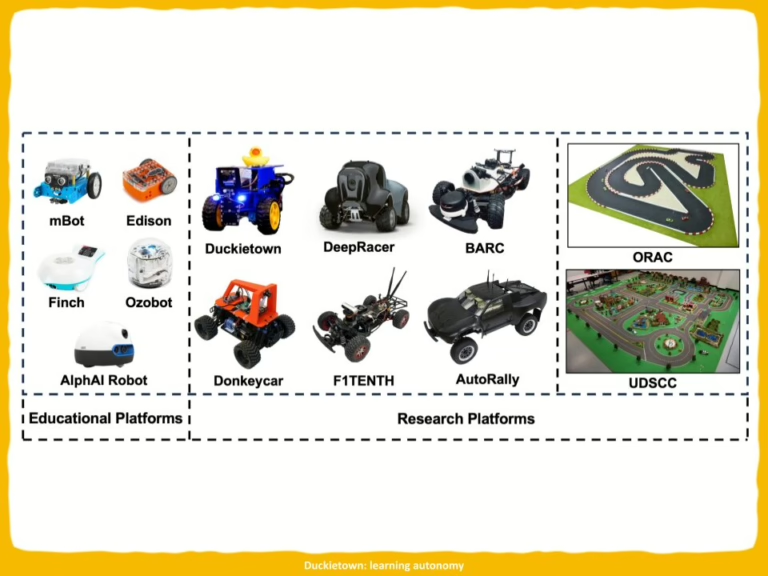
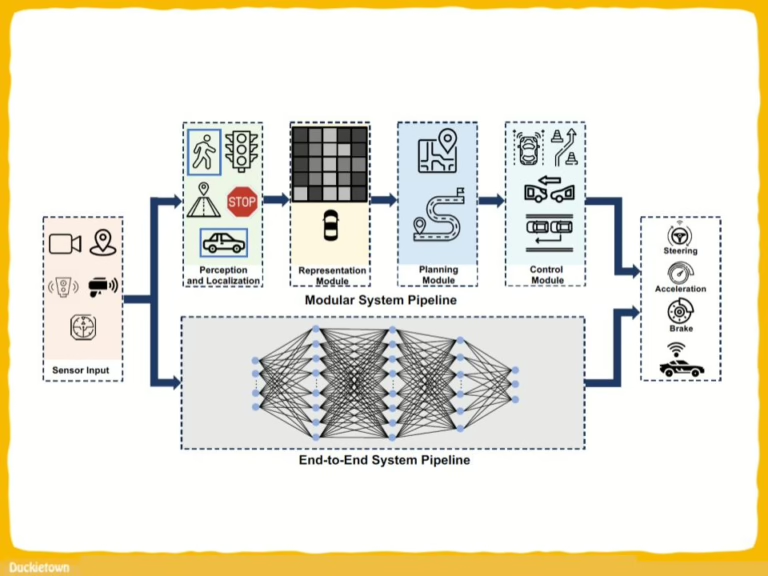
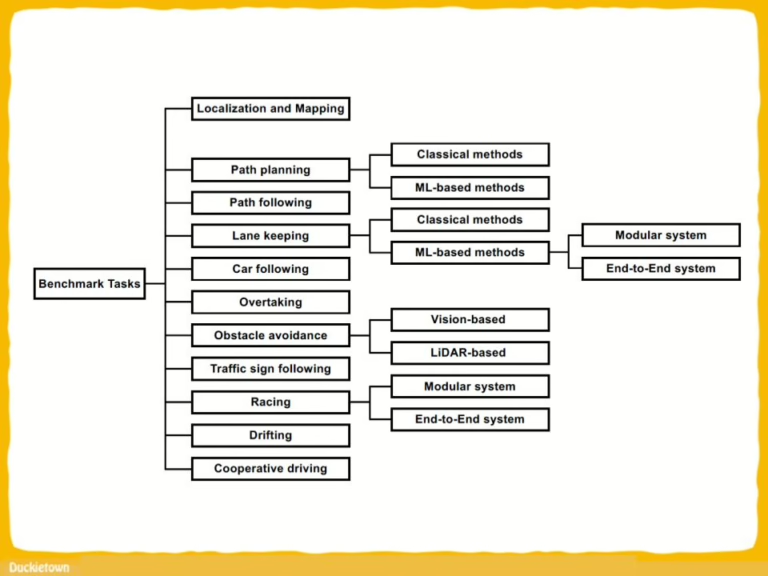
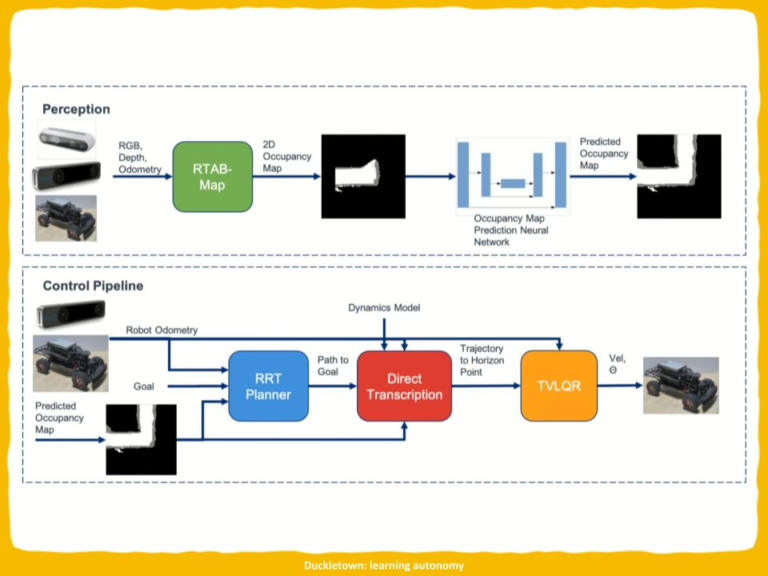
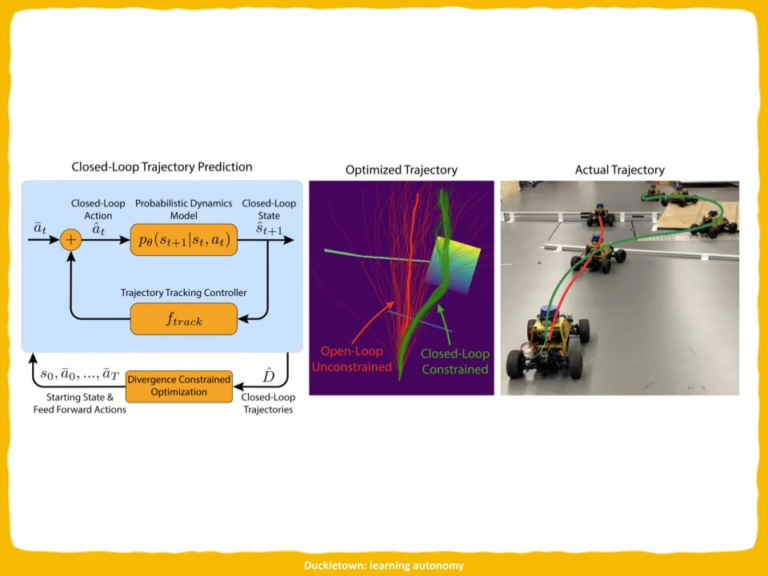
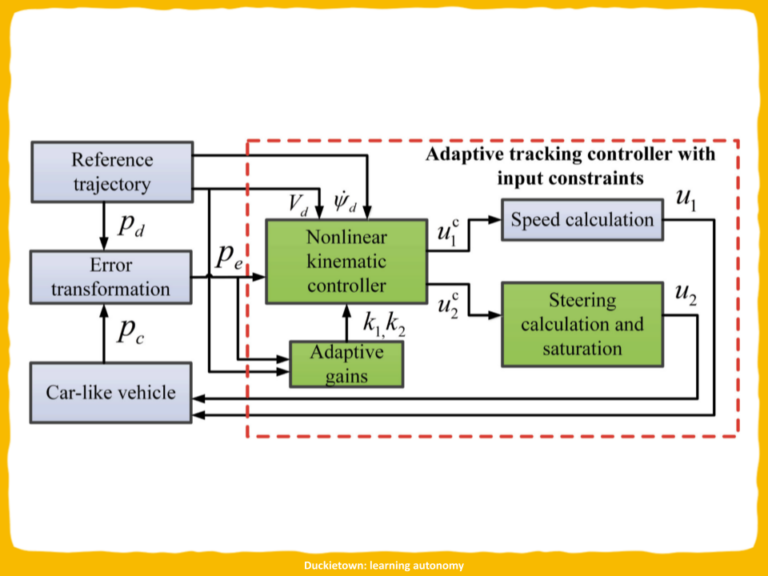
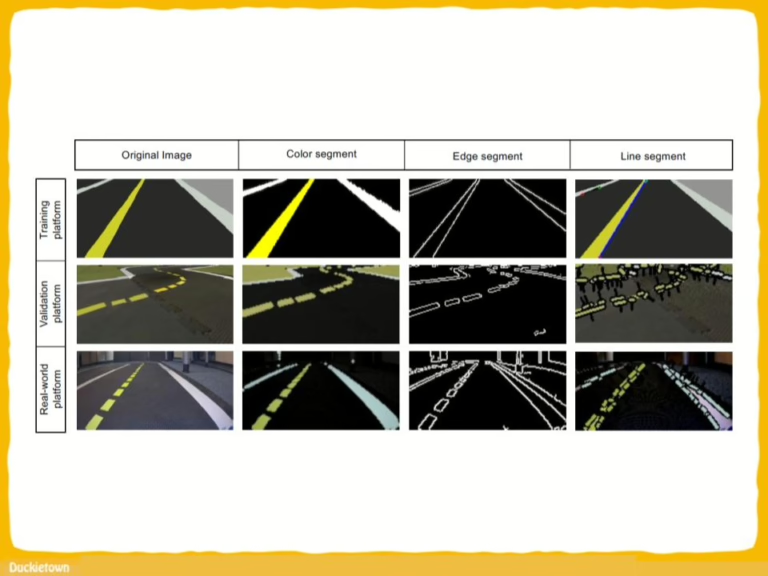
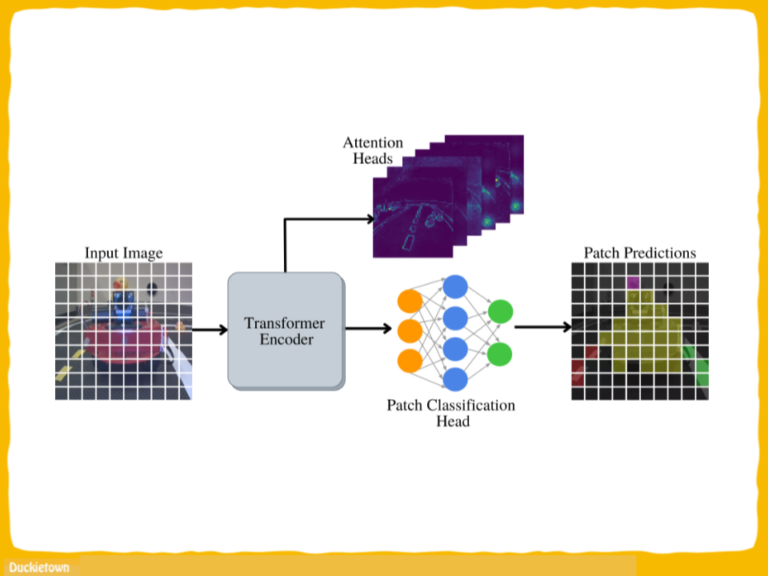
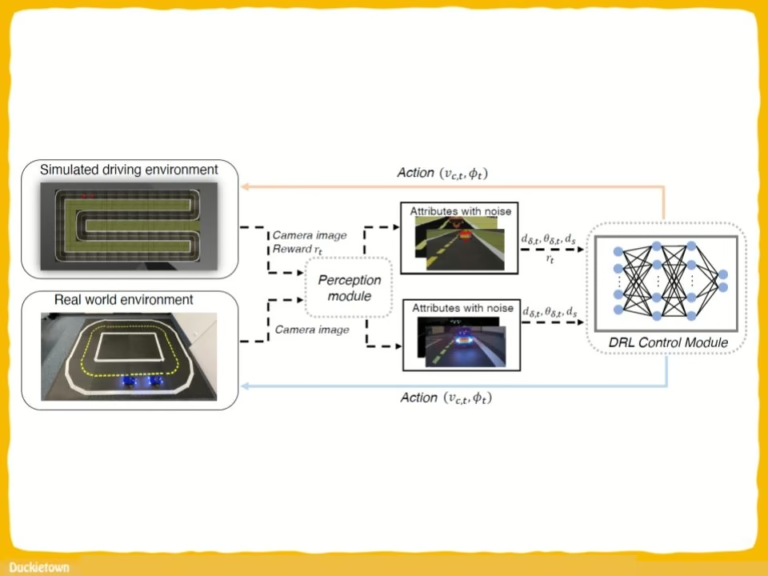
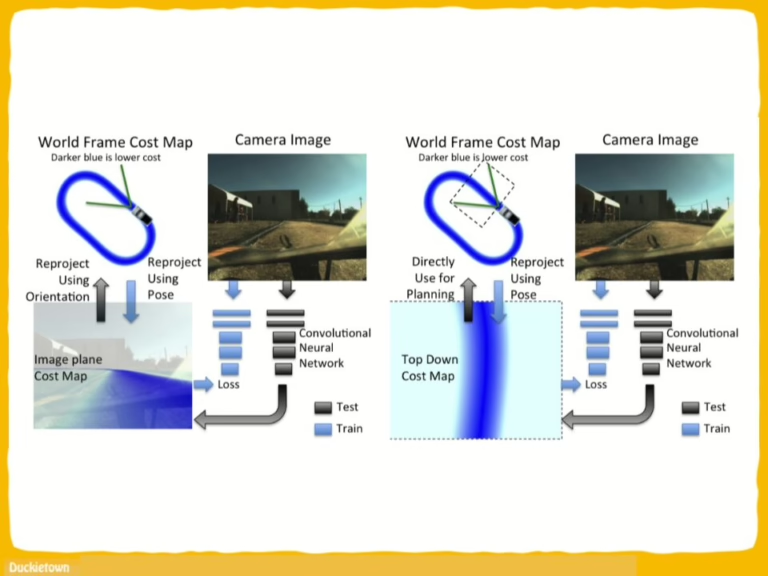
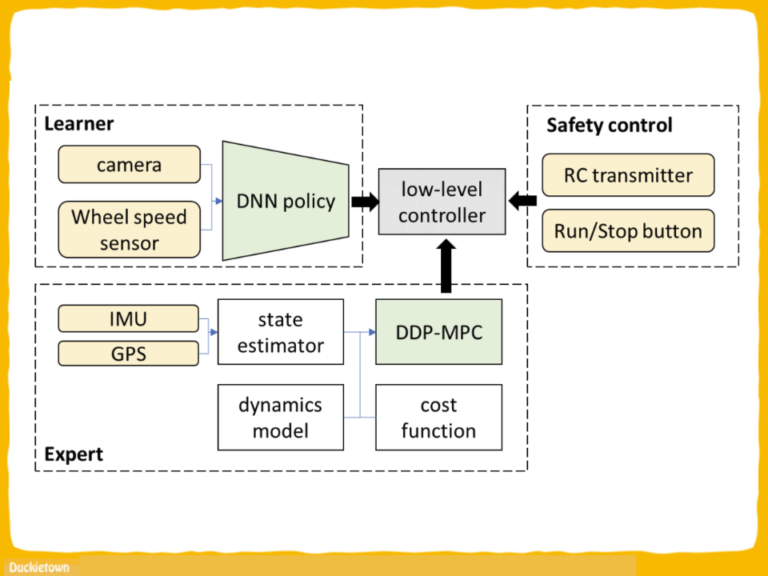
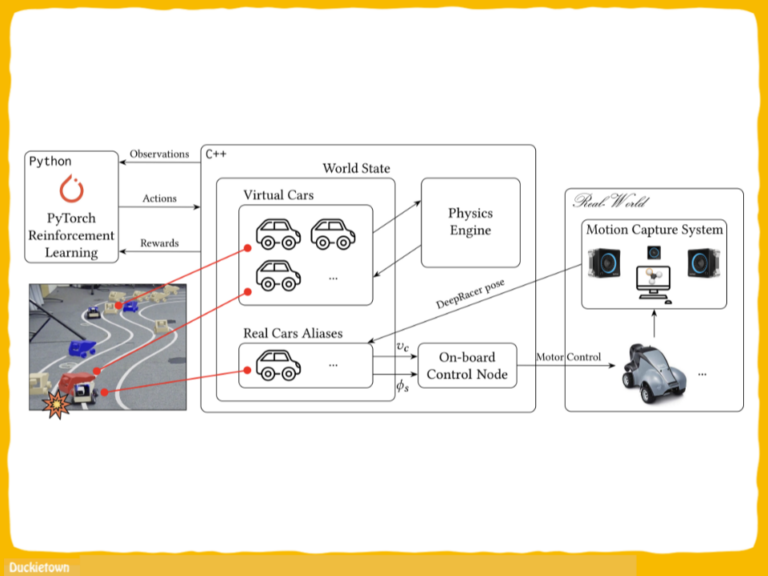
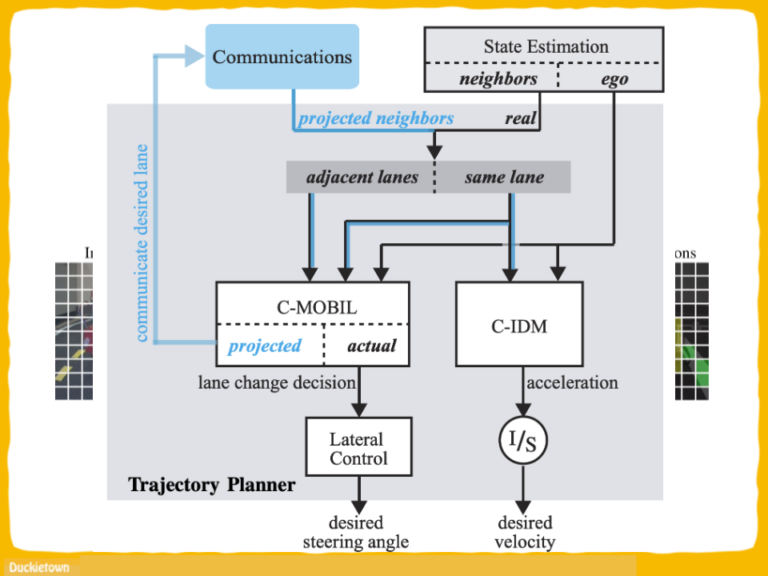
Summary and conclusion
Here is what the authors learned from this survey:
“In this paper, we offer an overview of the current state-of-the- art developments in small-scale autonomous cars. Through a detailed exploration of both past and ongoing research in this domain, we illuminate the promising trajectory for the advancement of autonomous driving technology with small-scale cars. We initially enumerate the presently predominant small-scale car platforms widely employed in academic and educational domains and present the configuration specifics of each platform. Similar to their full-size counterparts, the deployment of hyper-realistic simulation environments is imperative for training, validating, and testing autonomous systems before real-world implementation. To this end, we show the commonly employed universal simulators and platform-specific simulators.
Furthermore, we provide a detailed summary and categorization of tasks accomplished by small-scale cars, encompassing localization and mapping, path planning and following, lane-keeping, car following, overtaking, racing, obstacle avoidance, and more. Within each benchmarked task, we classify the literature into distinct categories: end-toend systems versus modular systems and traditional methods 20 versus ML-based methods. This classification facilitates a nuanced understanding of the diverse approaches adopted in the field. The collective achievements of small-scale cars are thus showcased through this systematic categorization. Since this paper aims to provide a holistic review and guide, we also outline the commonly utilized in various well-known platforms. This information serves as a valuable resource, enabling readers to leverage our survey as a guide for constructing their own platforms or making informed decisions when considering commercial options within the community.
We additionally present future trends concerning small-scale car platforms, focusing on different primary aspects. Firstly, enhancing accessibility across a broad spectrum of enthusiasts: from elementary students and colleagues to researchers, demands the implementation of a comprehensive learning pipeline with diverse entry levels for the platform. Next, to complete the whole ecosystem of the platform, a powerful car body, varying weather conditions, and communications issues should be addressed in a smart city setup. These trends are anticipated to shape the trajectory of the field, contributing significantly to advancements in real-world autonomous driving research.
While we have aimed to achieve maximum comprehensiveness, the expansive nature of this topic makes it challenging to encompass all noteworthy works. Nonetheless, by illustrating the current state of small-scale cars, we hope to offer a distinctive perspective to the community, which would generate more discussions and ideas leading to a brighter future of autonomous driving with small-scale cars.”
Project Authors
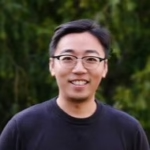
Dianzhao Li is a research assistant at the Technische Universität Dresden, Dresden, Germany.
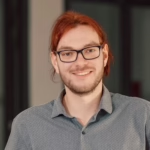
Paul Auerbach is with Barkhausen Institut gGmbH, Dresden, Germany
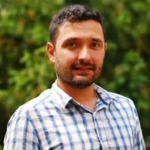
Ostap Okhrin is Chair of Statistics and Econometrics at the Institute of Economics and Transport, School of Transportation, Technische Universitat Dresden in Germany.
Learn more
Duckietown is a platform for creating and disseminating robotics and AI learning experiences.
It is modular, customizable and state-of-the-art, and designed to teach, learn, and do research. From exploring the fundamentals of computer science and automation to pushing the boundaries of knowledge, Duckietown evolves with the skills of the user.
End-to-end Deep RL (DRL) systems: in autonomous driving environments that rely on visual input for vehicle control face potential security risks, including:
- State Adversarial Perturbations: Subtle alterations to visual input that mislead the DRL agent, causing incorrect decision-making.
- Reward Tampering: Manipulation of the reward signal to misguide the learning process, leading the agent to adopt unsafe or inefficient policies.
These vulnerabilities can compromise the safety and reliability of self-driving vehicles.