General Information
- Title: Embedded Out-of-Distribution Detection on an Autonomous Robot Platform
- Authors: Michael Yuhas, Yeli Feng, Daniel Jun Xian Ng, Zahra Rahiminasab, Arvind Easwaran
- Institution: Nanyang Technological University, Singapore
- Citation: Yuhas, M., Feng, Y., Ng, D.J.X., Rahiminasab, Z. and Easwaran, A., 2021, May. Embedded out-of-distribution detection on an autonomous robot platform. In Proceedings of the Workshop on Design Automation for CPS and IoT (pp. 13-18).
Embedded Out-of-Distribution Detection in Duckietown
The project “embedded out-of-distribution detection (OOD) Detection on an Autonomous Robot Platform” focuses on safety in Duckietown by implementing real-time OOD detection on the Duckiebots. The concept involves using a machine learning-based OOD detector, specifically a β-Variational Autoencoder (β-VAE), to identify test inputs that deviate from the training data’s distribution. Such inputs can lead to unreliable behavior in machine learning systems, critical for safety in autonomous platforms like the Duckiebot.
Key aspects of the project include:
- Integration: The β-VAE OOD detector is integrated with the Duckiebot’s ROS-based architecture, alongside lane-following and motor control modules.
- Emergency Braking: An emergency braking mechanism halts the Duckiebot when OOD inputs are detected, ensuring safety during operation.
- Evaluation: Performance was evaluated in scenarios where the Duckiebot navigated a track and avoided obstacles. The system achieved an 87.5% success rate in emergency stops.
This work demonstrates a method to mitigate safety risks in autonomous robotics. By providing a framework for OOD detection on low-cost platforms, the project contributes to the broader applicability of safe machine learning in cyber-physical systems.
Highlights - Embedded Out-of-Distribution Detection in Duckietown
Here is a visual tour of the work of the authors. For all the details, check out the full paper.
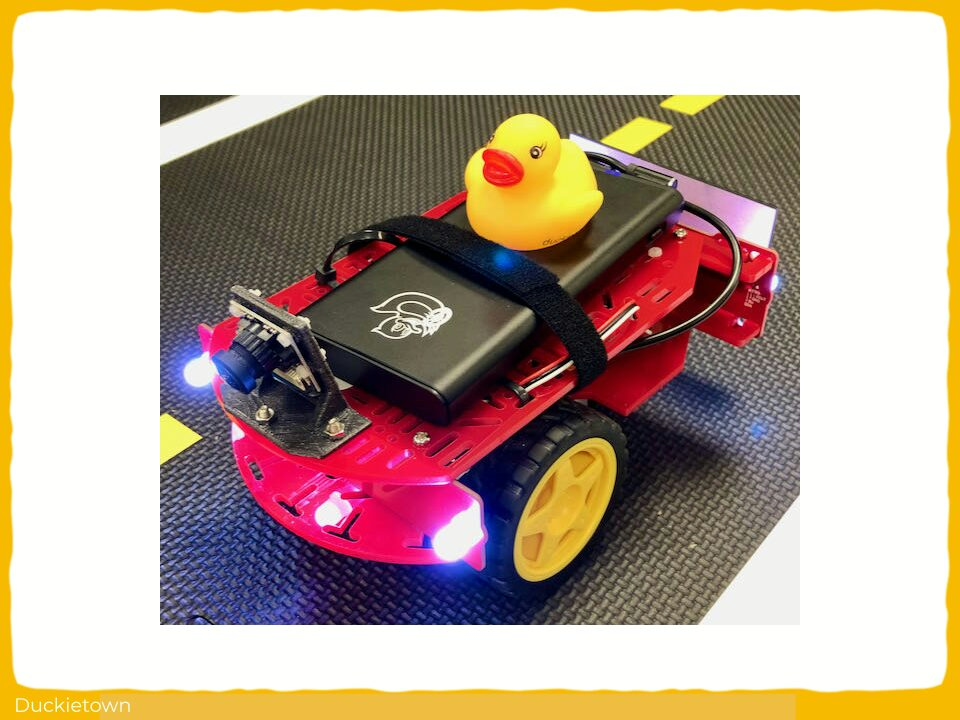
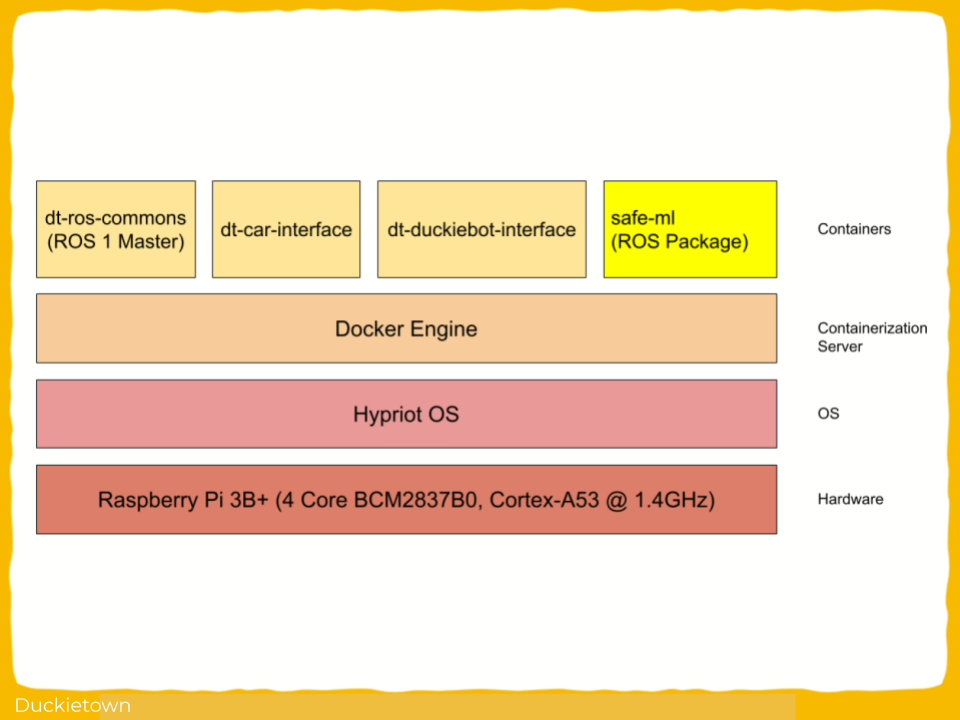
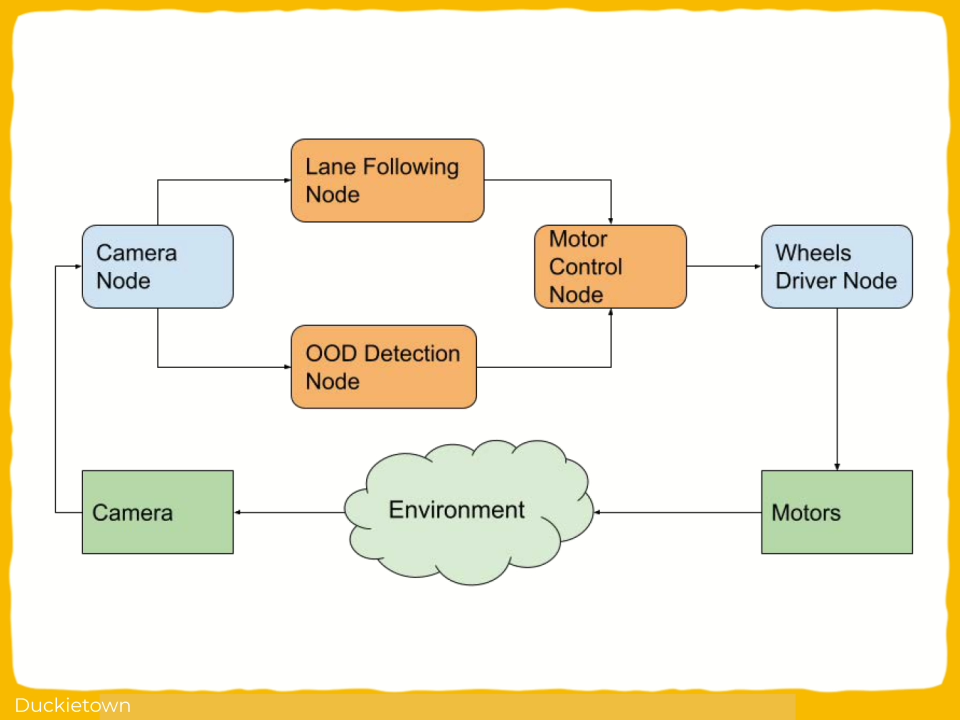
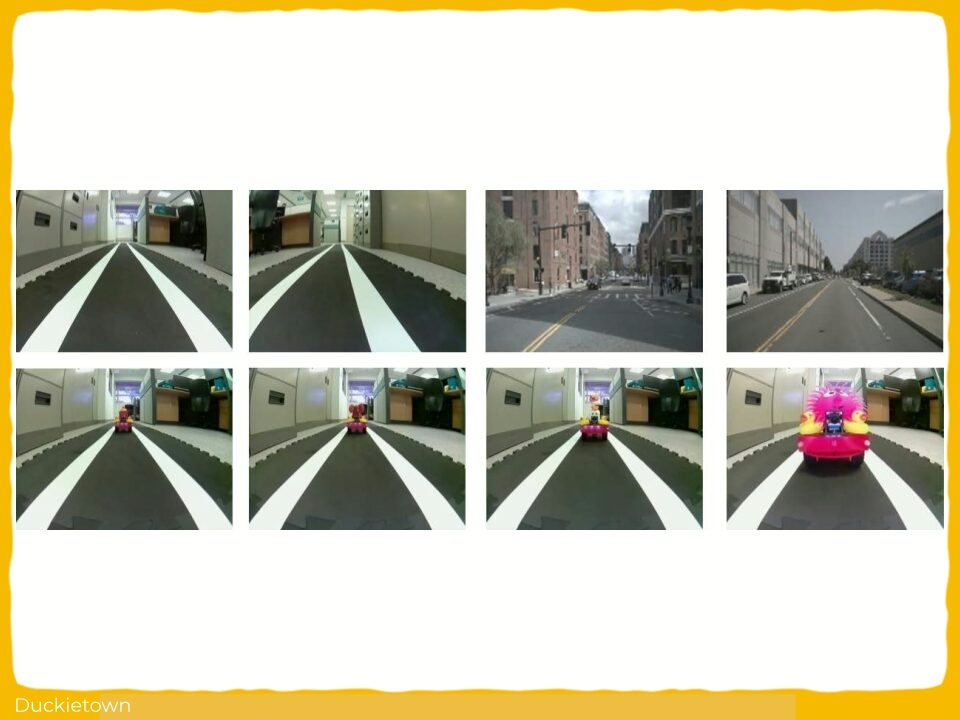
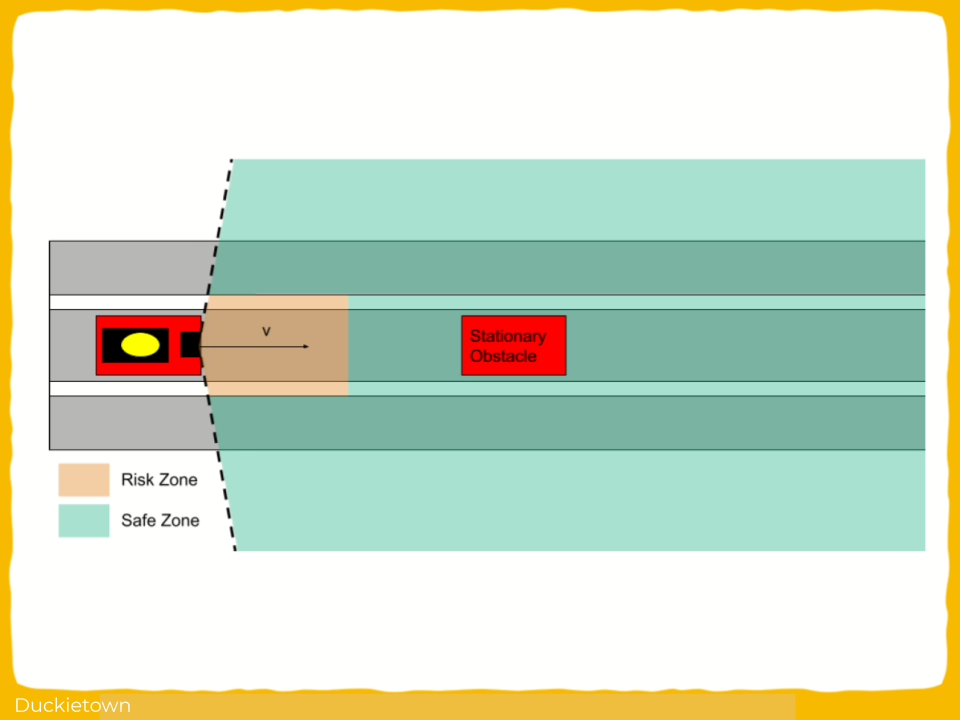
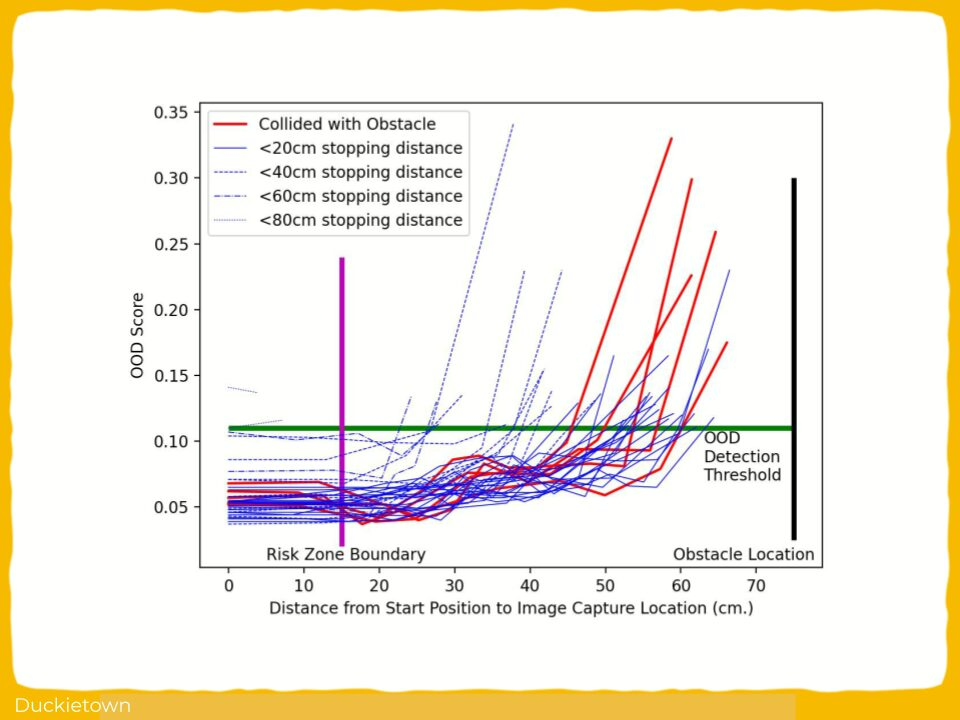
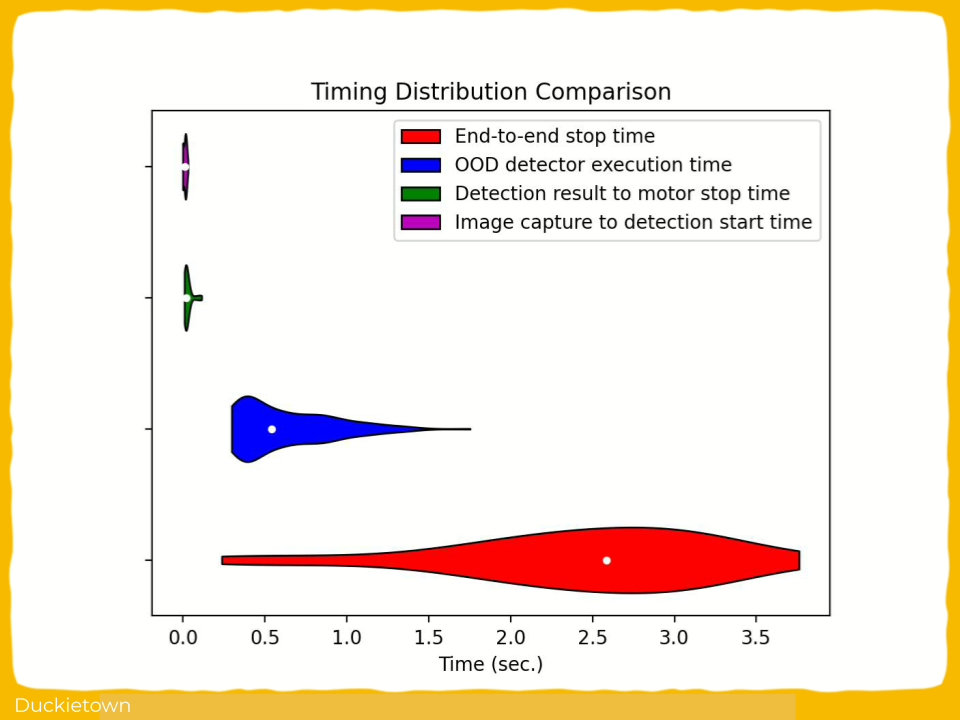
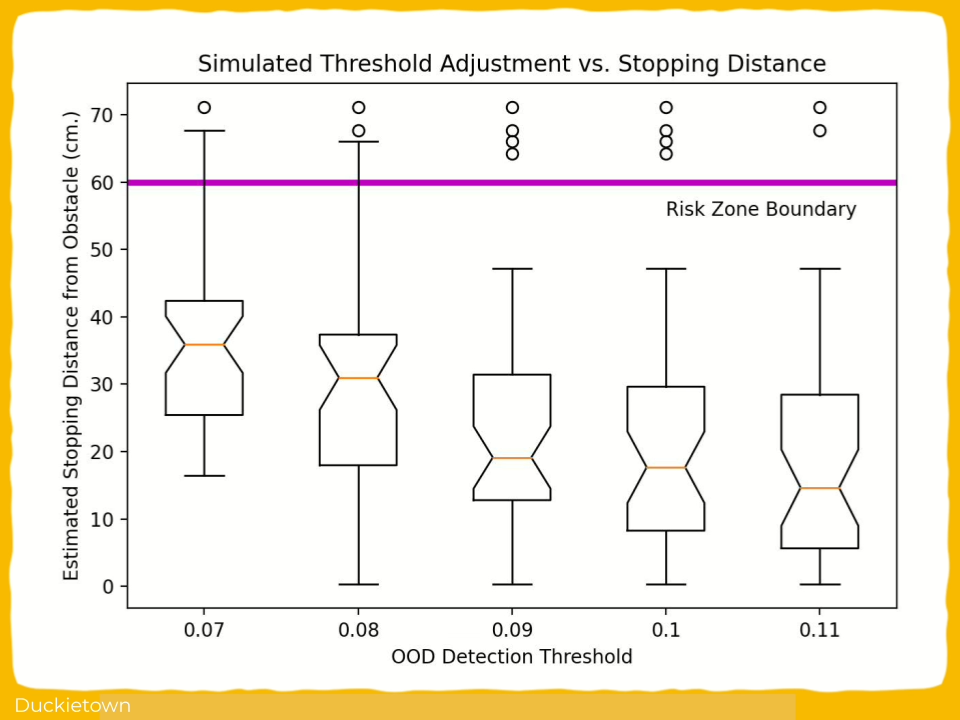
Abstract
In the author’s words:
Machine learning (ML) is actively finding its way into modern cyber-physical systems (CPS), many of which are safety-critical real-time systems. It is well known that ML outputs are not reliable when testing data are novel with regards to model training and validation data, i.e., out-of-distribution (OOD) test data. We implement an unsupervised deep neural network-based OOD detector on a real-time embedded autonomous Duckiebot and evaluate detection performance. Our OOD detector produces a success rate of 87.5% for emergency stopping a Duckiebot on a braking test bed we designed. We also provide case analysis on computing resource challenges specific to the Robot Operating System (ROS) middleware on the Duckiebot.
Conclusion - Embedded Out-of-Distribution Detection in Duckietown
Here are the conclusions from the author of this paper:
“We successfully demonstrated that the đť›˝-VAE OOD detection algorithm could run on an embedded platform and provides a safety check on the control of an autonomous robot. We also showed that performance is dependent on real-time performance of the embedded system, particularly the OOD detector execution time. Lastly, we showed that there is a trade-off involved in choosing an OOD detection threshold; a smaller threshold value increases the average stopping distance from an obstacle, but leads to an increase in false positives.
This work also generates new questions that we hope to investigate in the future. The system architecture demonstrated in this paper was not utilizing a real-time OS and did not take advantage of technologies such as GPUs or TPUs, which are now becoming common on embedded systems. There is still much work that can be done to optimize process scheduling and resource utilization while maintaining the goal of using low-cost, off-the-shelf hardware and open-source software. Understanding what quality of service can be provided by a system with these constraints and whether it suffices for reliable operations of OOD detection algorithms is an ongoing research theme.
From the OOD detection perspective, we would like to run additional OOD detection algorithms on the same architecture and compare performance in terms of accuracy and computational efficiency. We would also like to develop a more comprehensive set of test scenarios to serve as a benchmark for OOD detection on embedded systems. These should include dynamic as well as static obstacles, operation in various environments and lighting conditions, and OOD scenarios that occur while the robot is performing more complex tasks like navigating corners, intersections, or merging with other traffic.
Demonstrating OOD detection on the Duckietown platform opens the door for more embedded applications of OOD detectors. This will serve to better evaluate their usefulness as a tool to enhance the safety of ML systems deployed as part of critical CPS.”
Did this work spark your curiosity?
The authors followed up with additional research on this very topic:
Other works using variational autoencoders with Duckietown:
Project Authors
Michael Yuhas is currenly working as a Research Assistant and pursuing his PhD at the Nanyang Technological University, Singapore.
Yeli Feng is currenly working as a Lead Data Scientist at Amplify Health, Singapore.
Daniel Jun Xian Ng is currenly working as a Mobile Robot Software Engineer at the Hyundai Motor Group Innovation Center Singapore (HMGICS), Singapore.
Zahra Rahiminasab is currenly working as a Postdoctoral Researcher at Aalto University, Finland.
Arvind Easwaran is currenly working as an Associate Professor at the Nanyang Technological University, Singapore.
Learn more
Duckietown is a platform for creating and disseminating robotics and AI learning experiences.
It is modular, customizable and state-of-the-art, and designed to teach, learn, and do research. From exploring the fundamentals of computer science and automation to pushing the boundaries of knowledge, Duckietown evolves with the skills of the user.