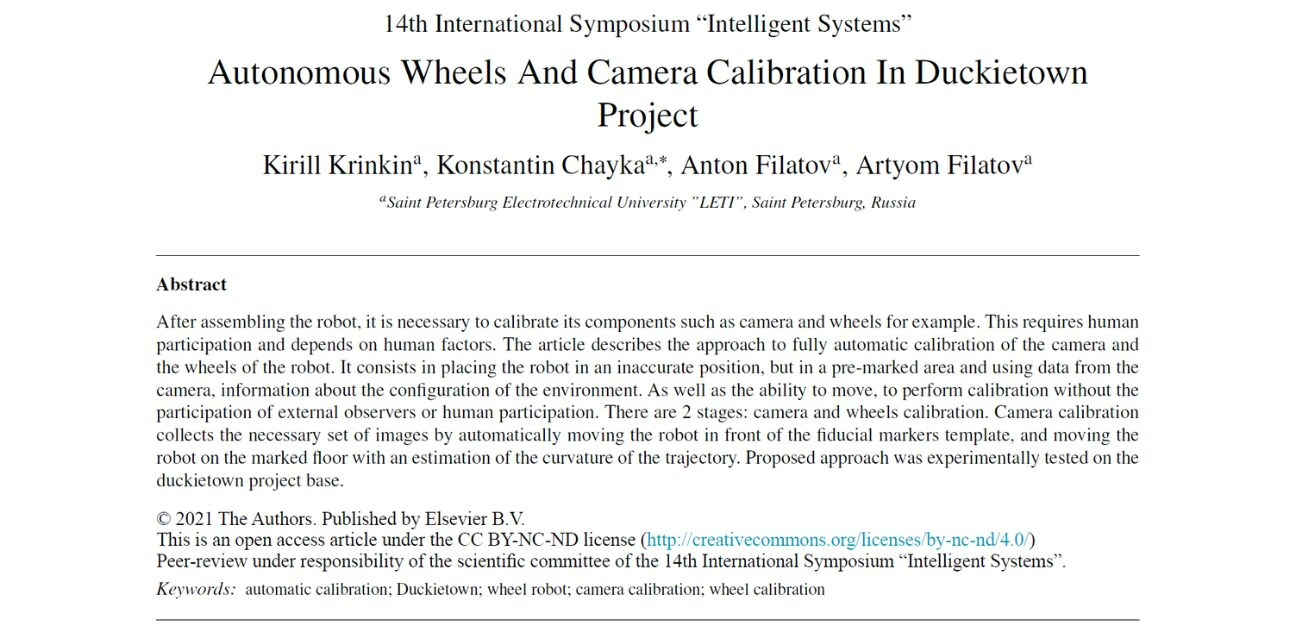
Category: Research
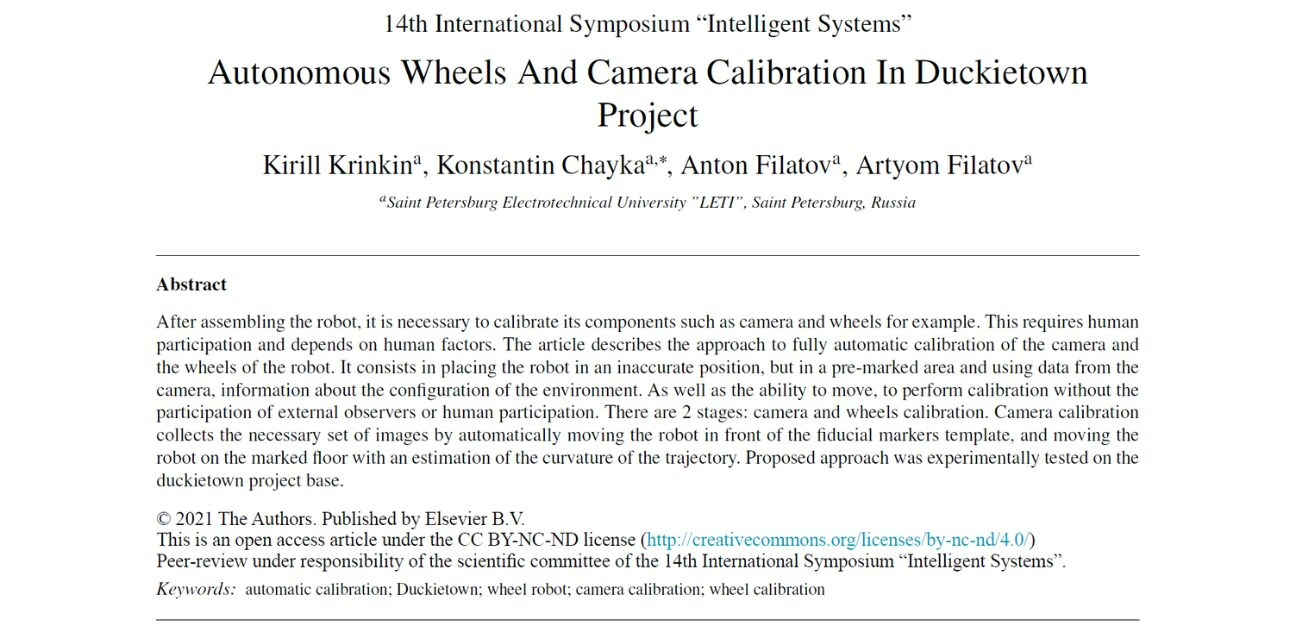
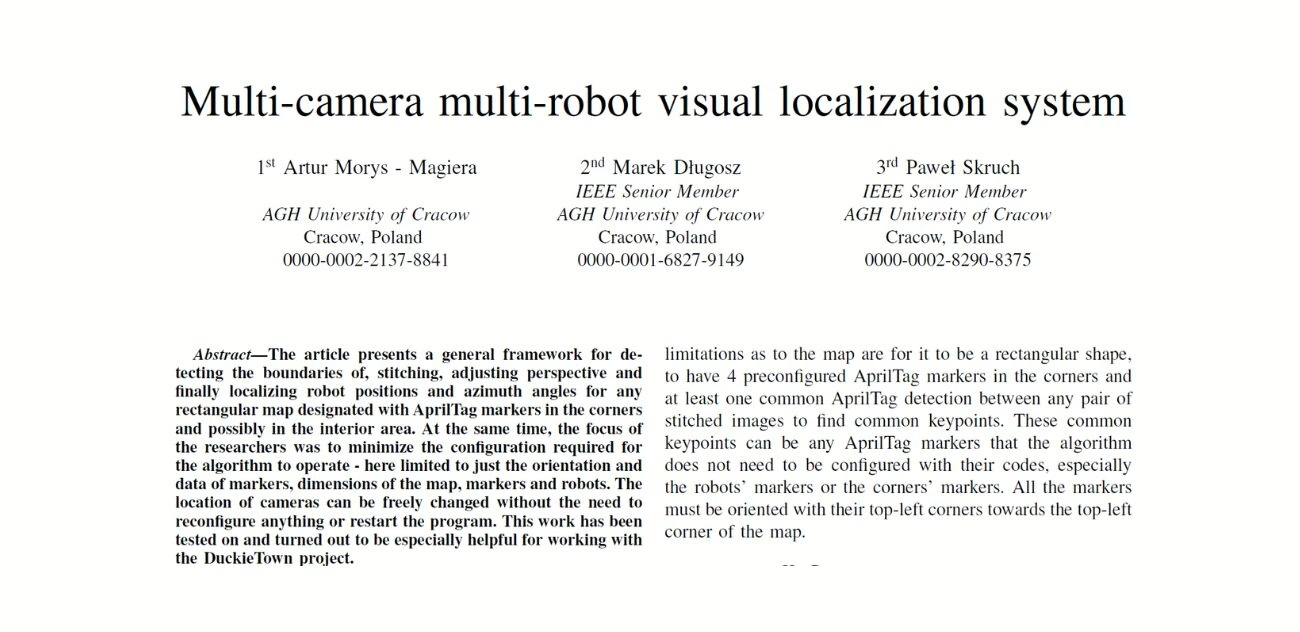
Visual localization using multi-camera multi-robot system
General Information
- Title: Multi-camera multi-robot visual localization system
- Authors: Artur Morys Magiera, Marek Długosz, Paweł Skruch.
- Institution: AGH University of Cracow, Poland
- Citation: A. M. Magiera, M. Długosz and P. Skruch, "Multi-camera multi-robot visual localization system," 2024 28th International Conference on Methods and Models in Automation and Robotics (MMAR) , Poland, 2024, pp. 375-380, doi: 10.1109/MMAR62187.2024.10680813.
Visual localization using multi-camera multi-robot system
Visual robot localization is a crucial problem in robotics: how to estimate the agents’ position using vision.
A common approach to solving it is through Simultaneous Localization and Mapping (SLAM) algorithms, using onboard sensors to map and estimate robot positions.
This work introduces a new algorithm for robot localization using AprilTag fiducial markers. It works on a rectangular map with four corner tags, requiring minimal configuration and offering flexibility in camera positions.
Unlike prior methods, this algorithm automatically stitches images from cameras, regardless of angle, and converts them into a top-down view for robot localization.
The approach promises flexibility, making adapting to dynamic camera setups easier without reconfiguration.
This solution offers automated robot localization with minimal setup, leveraging computer vision and AprilTags for more efficient mapping. The only constraint is the rectangular shape of the map and properly oriented corner markers, making it an ideal fit for scalable, adaptive robot environments.
Learn about robot autonomy, including perception, localization, and SLAM, starting from the link below!
Abstract
In the author’s words:
The article presents a general framework for detecting the boundaries of, stitching, adjusting perspective and finally localizing robot positions and azimuth angles for any rectangular map designated with AprilTag markers in the corners and possibly in the interior area.
At the same time, the focus of the researchers was to minimize the configuration required for the algorithm to operate – here limited to just the orientation and data of markers, dimensions of the map, markers and robots.
The location of cameras can be freely changed without the need to reconfigure anything or restart the program. This work has been tested on and turned out to be especially helpful for working with the Duckietown project.
Highlights - Visual localization using multi-camera multi-robot system
Here is a visual tour of the work of the authors. For more details, check out the full paper.
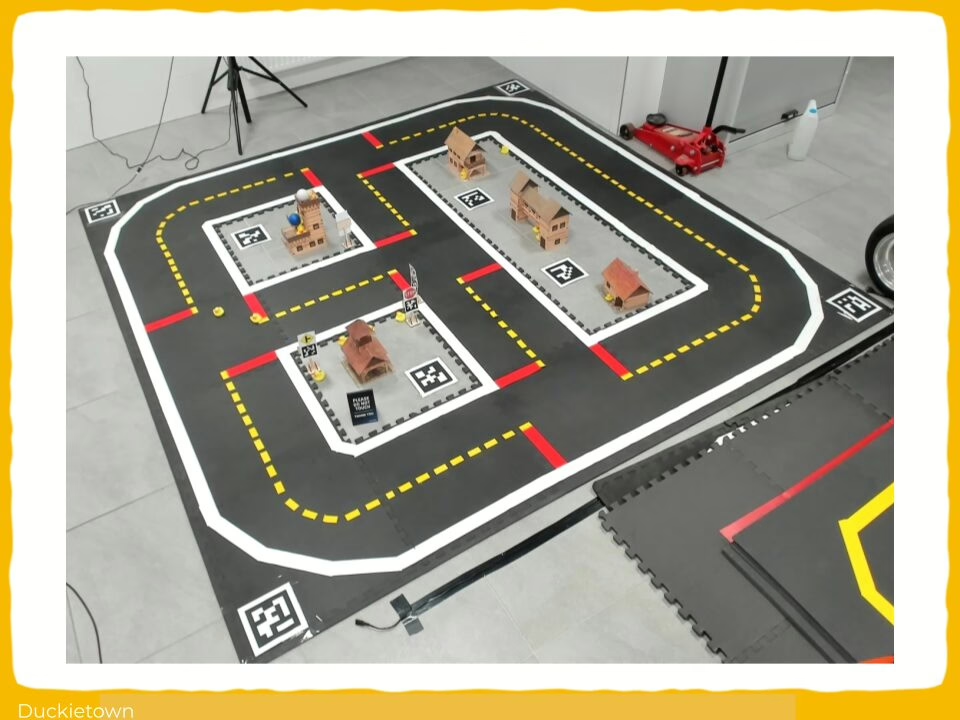
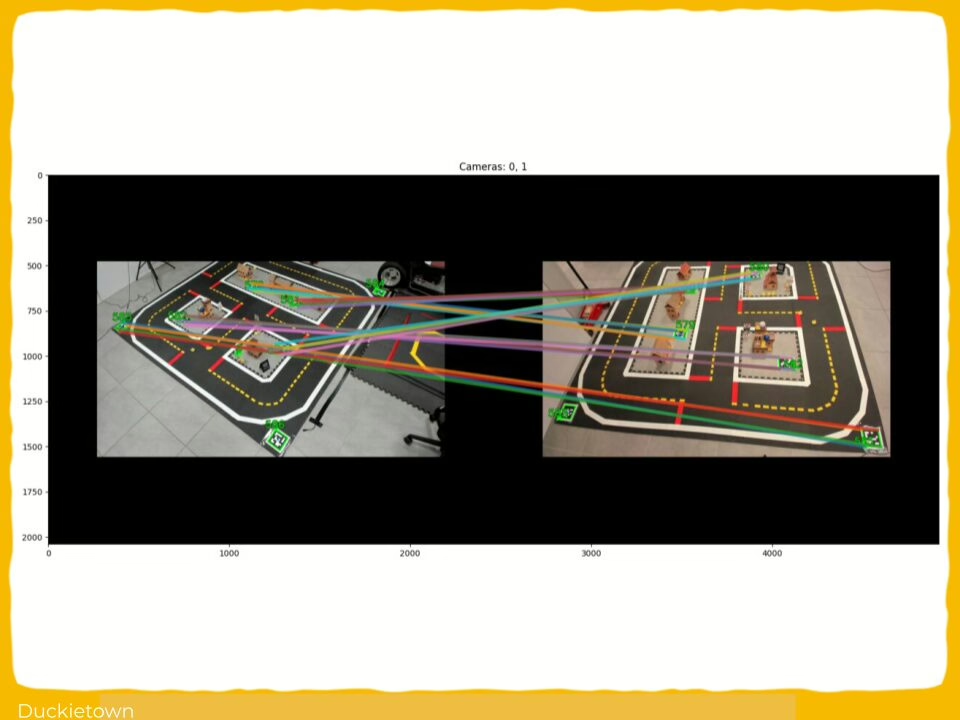
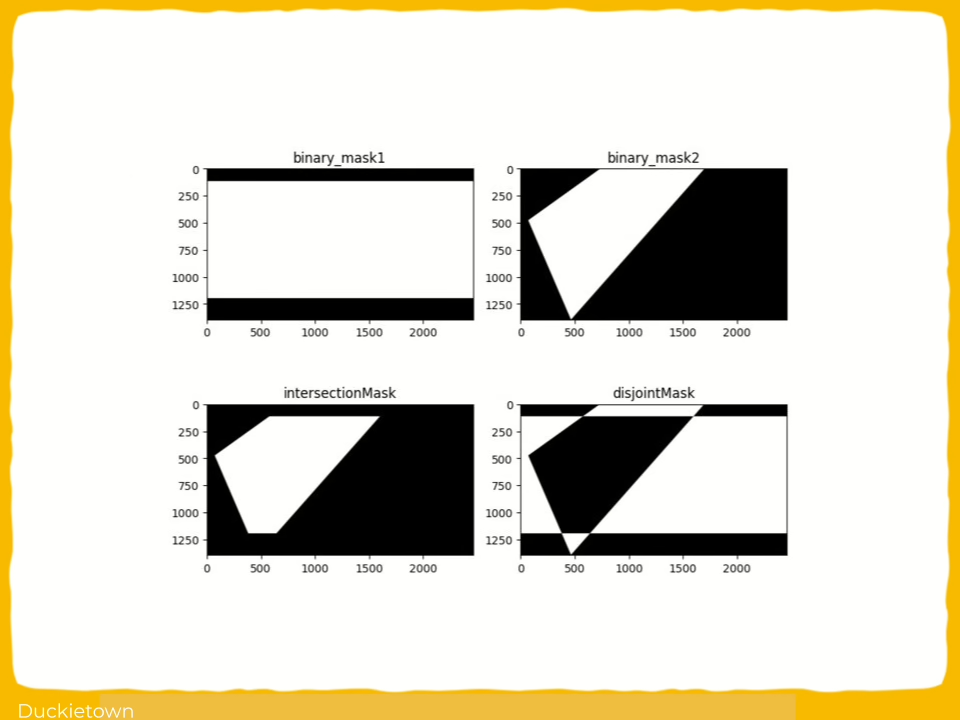
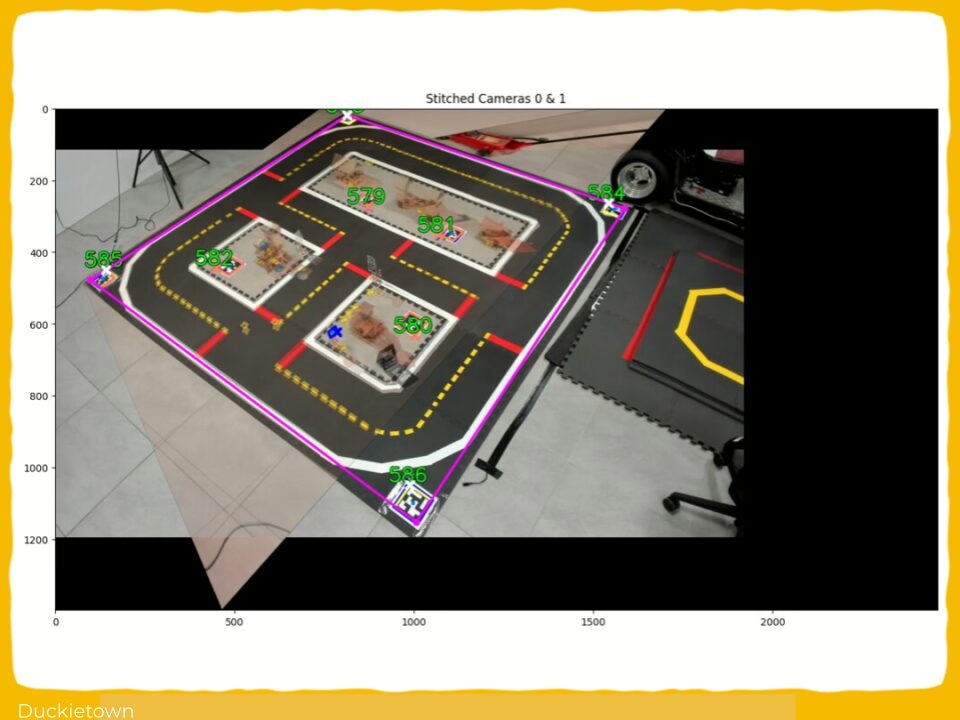
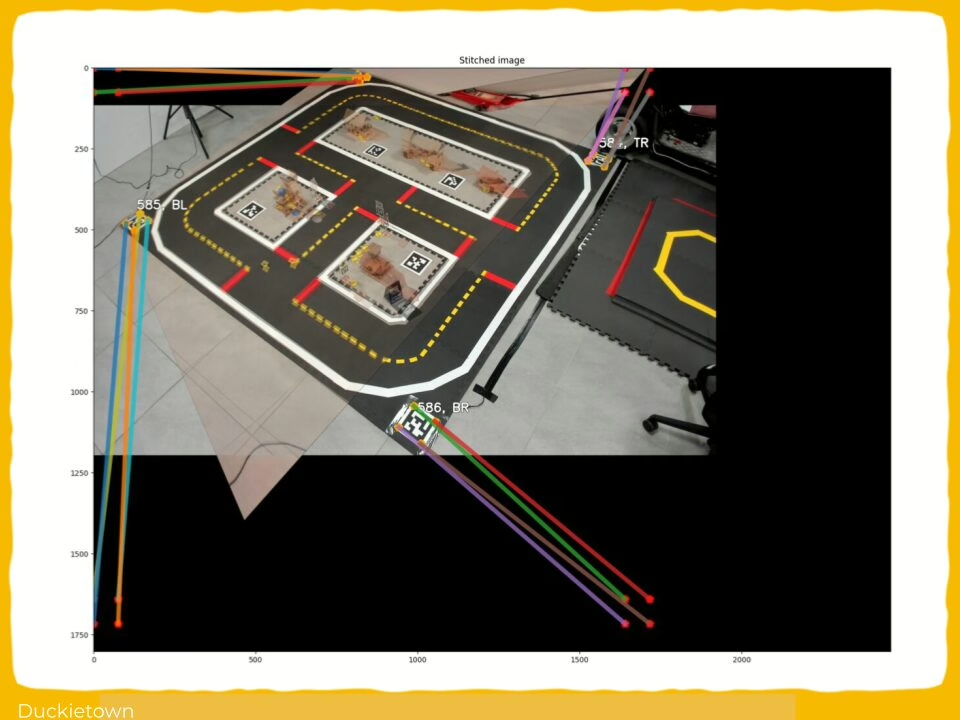
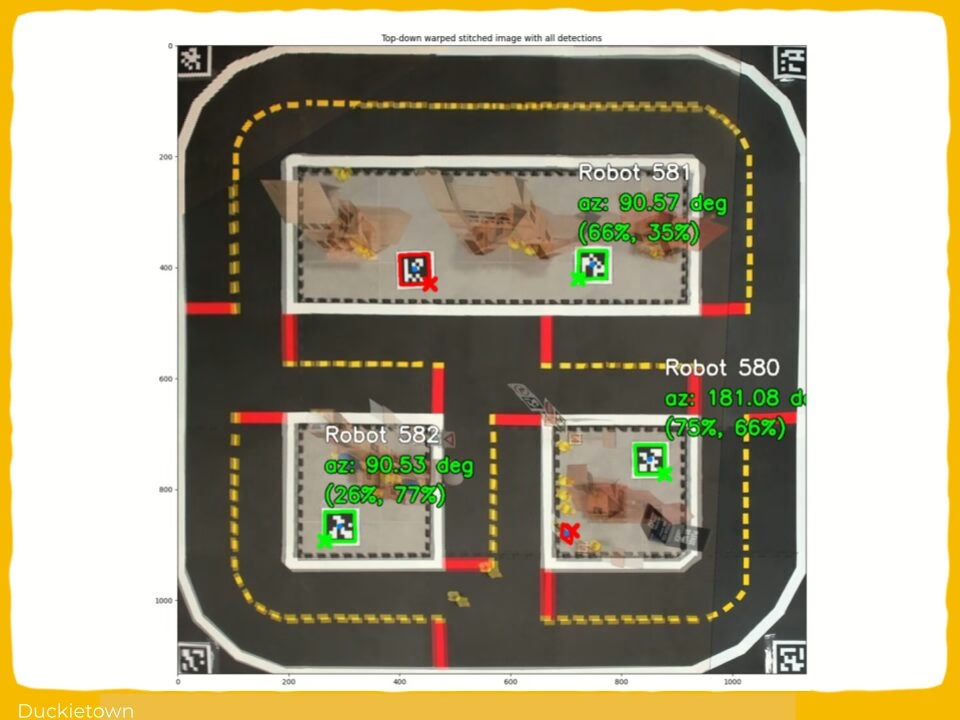
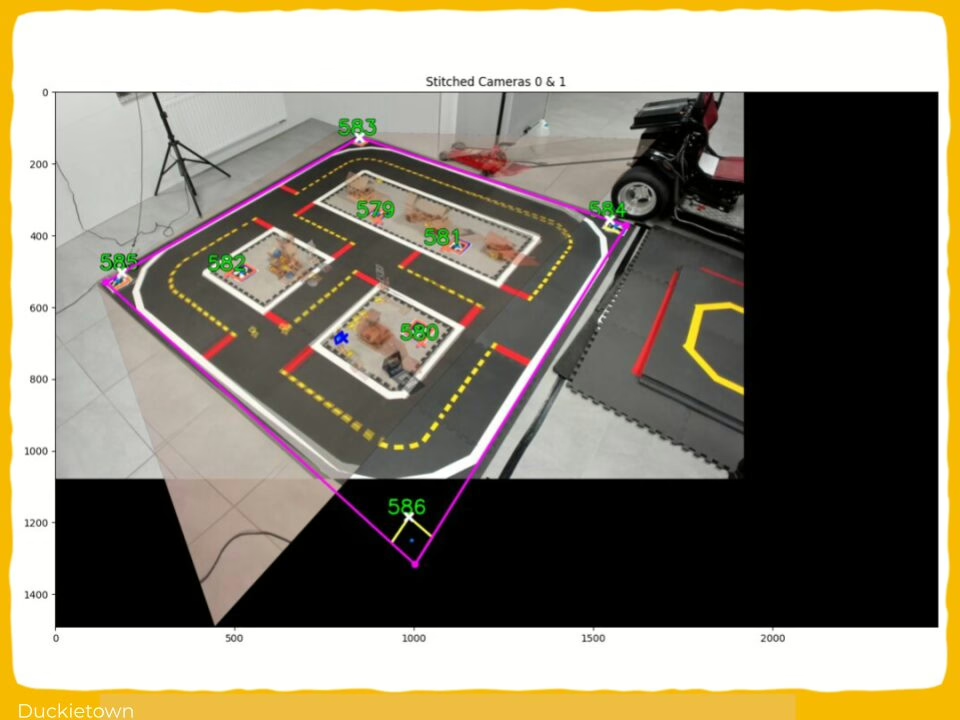
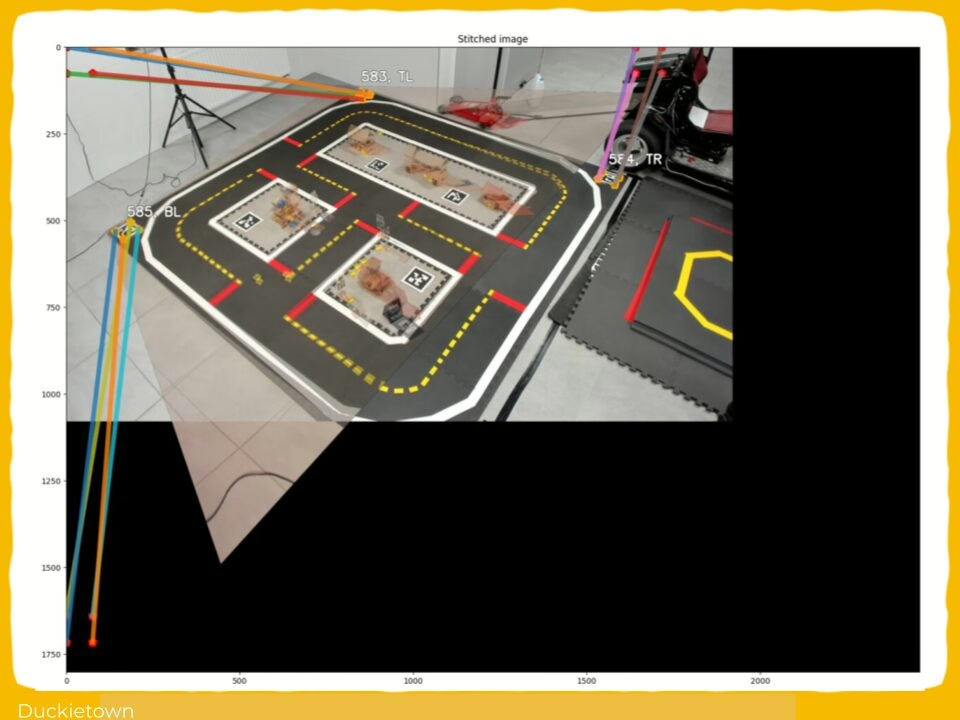
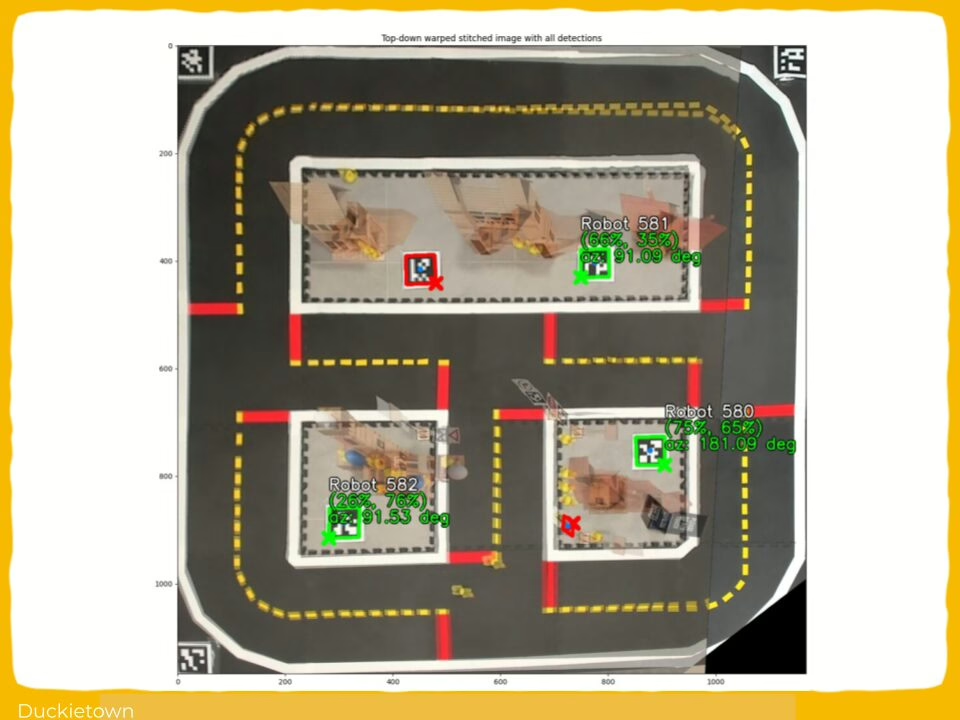
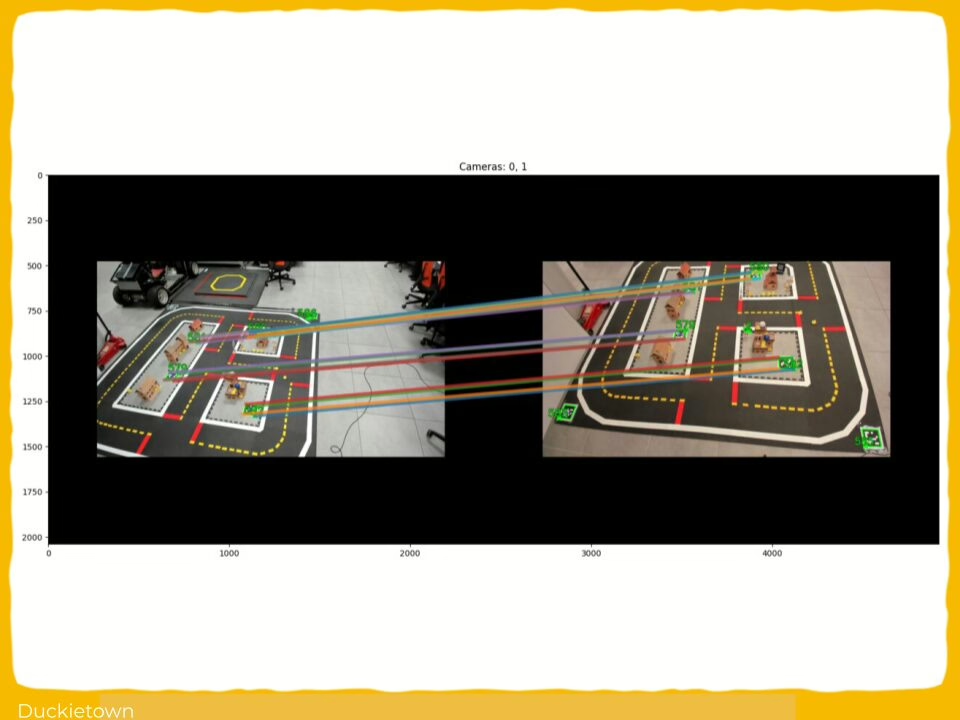
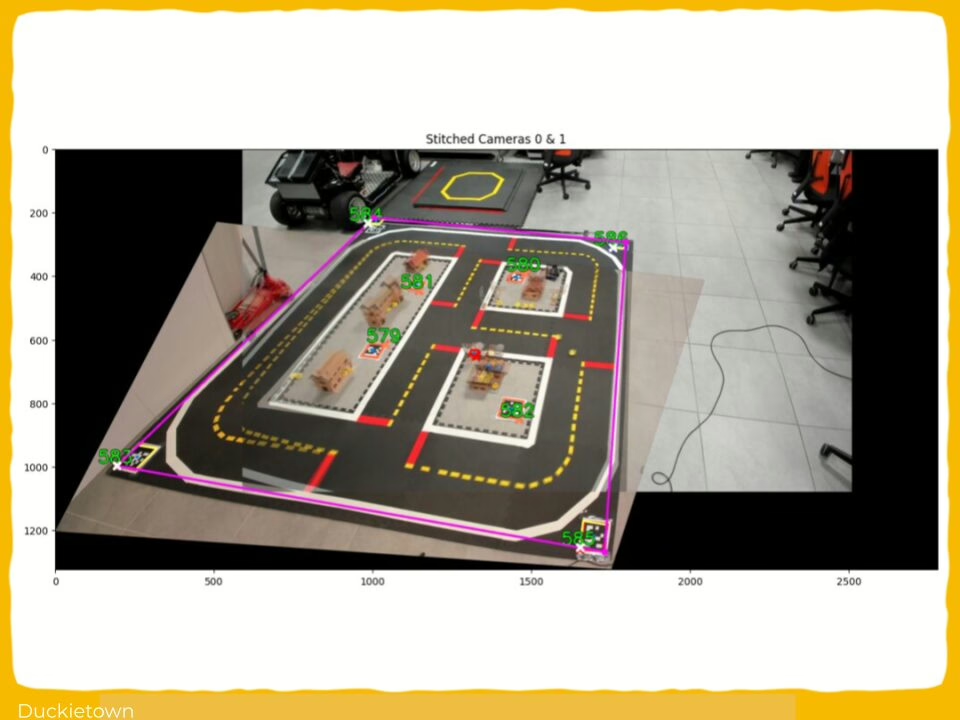
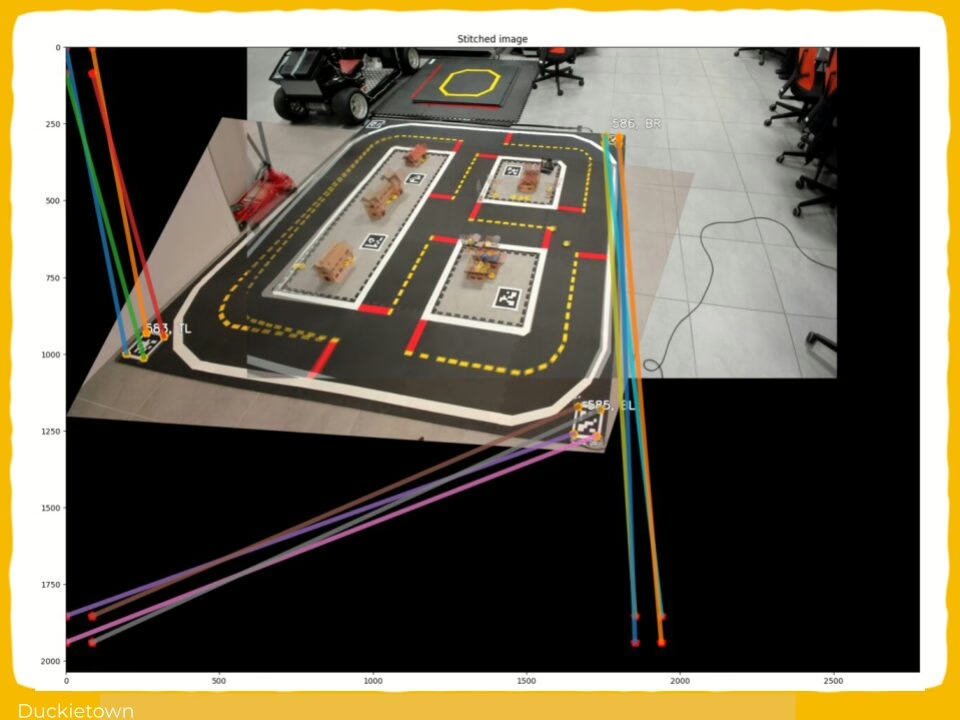
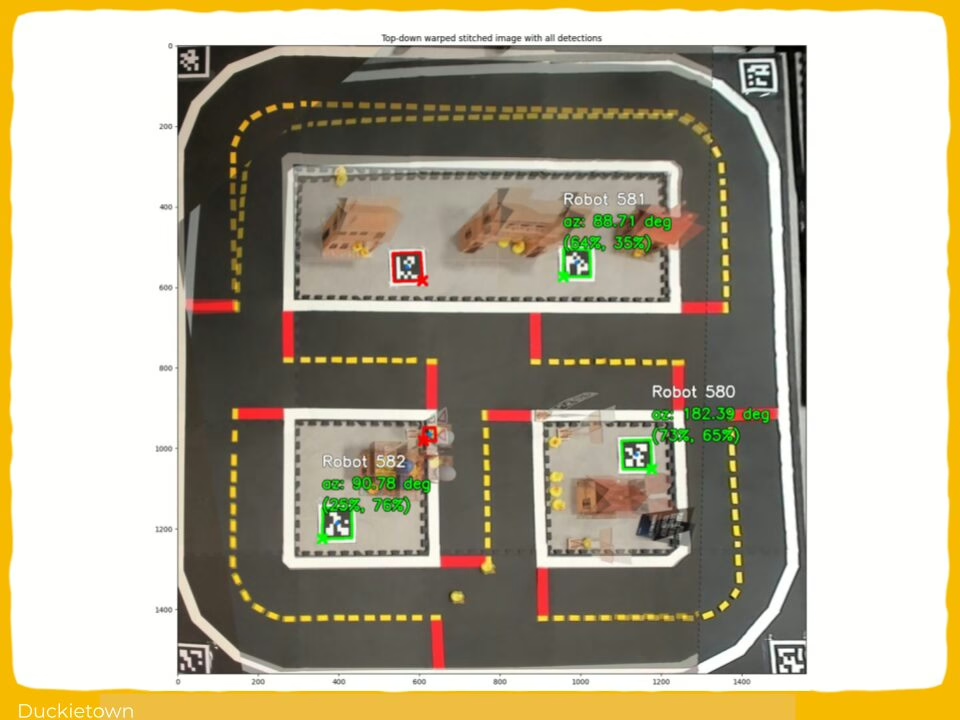
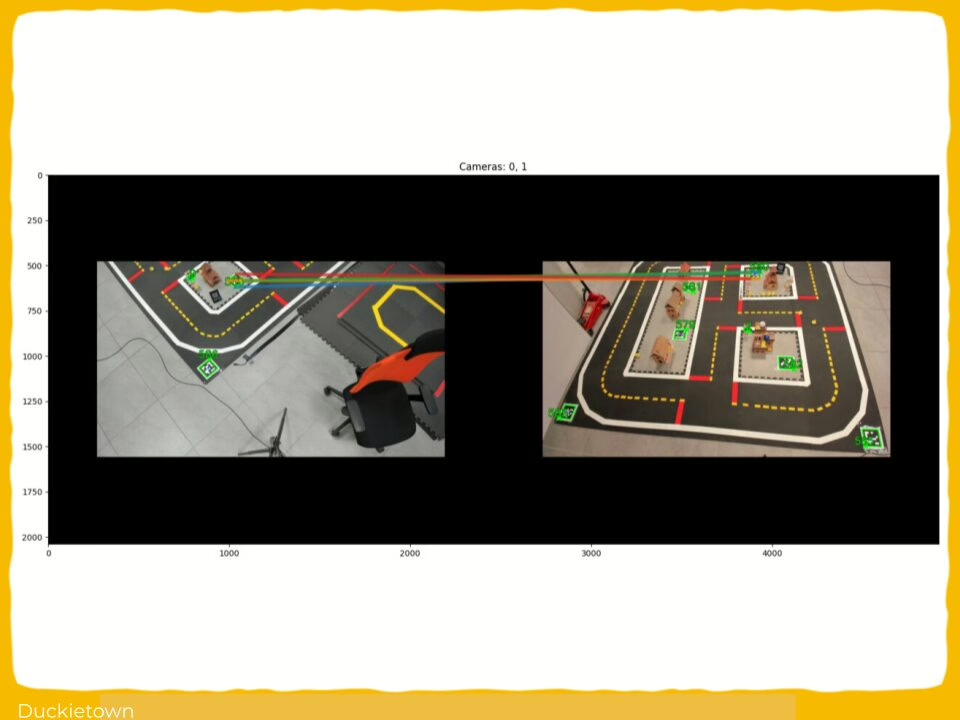
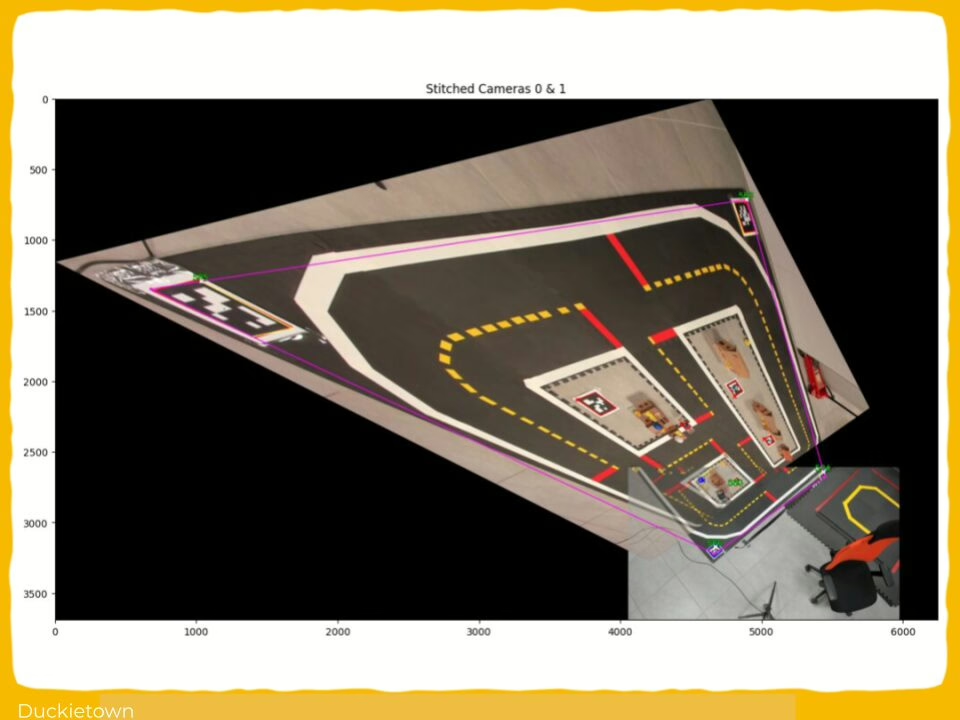
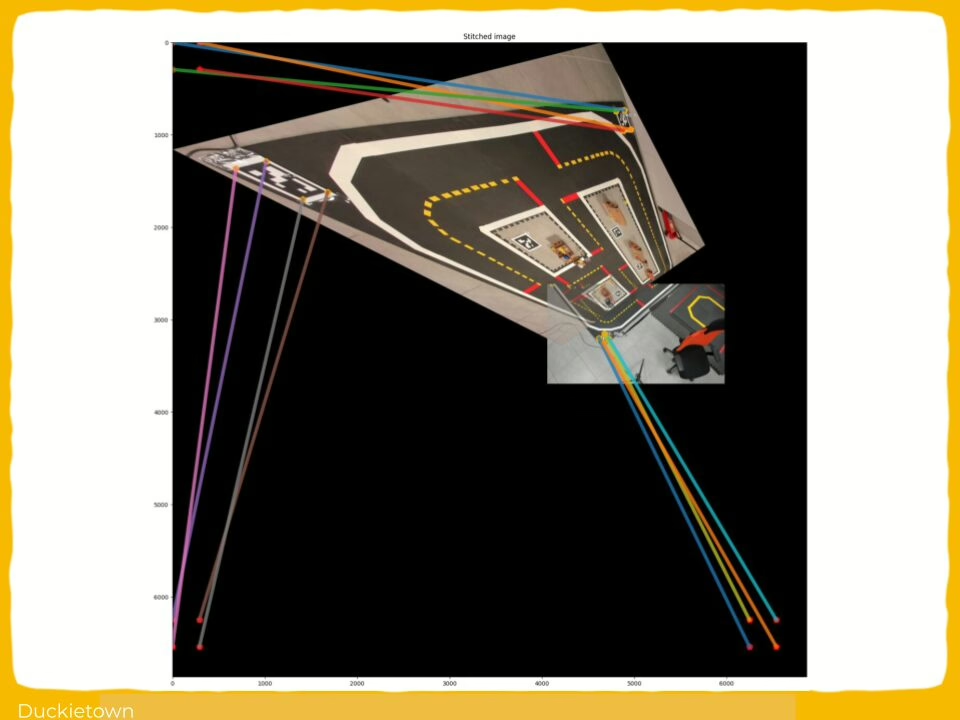
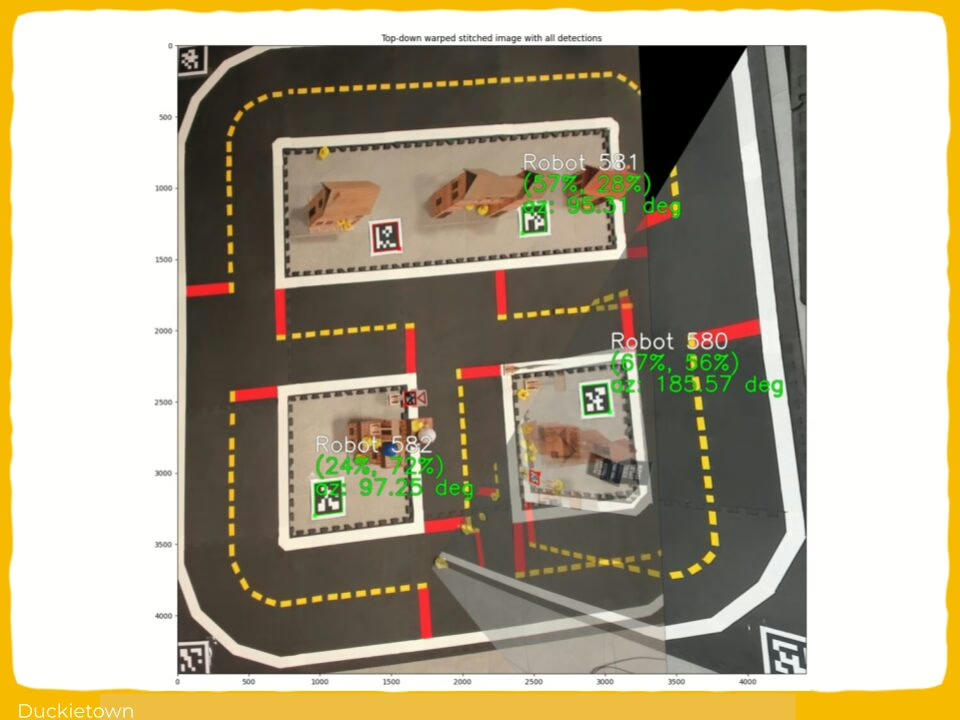
Conclusion - Visual localization using multi-camera multi-robot system
Here are the conclusions from the authors of this paper:
“The primary contribution and aim of this work is to provide a universal framework for stitching views of the same map from multiple cameras that can be freely moved and laid out around the map, with minimal required configuration.
The requirements for placement of codes are also loose: only the orientation with respect to the map frame is constrained and configuration of corner codes is required, as well as the lower limit of visible common markers on two images to be processed is 1, with no need for any corner markers to be present in both images at the same time.
The algorithms efficiency, however, depends on the quality of the homography matrices used in it, which implies that the more detections and corner detections, the better the result. It happens that the stitched / extrapolated coordinates may be off ’ground truth’ in some cases, or even stitching might fail, resulting in malformed output.
The authors provided experiments on two cameras, yet the algorithm may be run sequentially with images from more cameras. The algorithm may be improved in the future by applying more sophisticated methods of aggregating values of multiple detections of a given robot, such as a weighted combination of the position based on the quality of each detection.”
Project Authors
Marek Długosz is a graduate and faculty member of the Faculty of Electrical Engineering, Automatics, Computer Science and Biomedical Engineering at the AGH University of Science and Technology in Krakow, Poland.
Paweł Skruch is a Professor of the AGH University of Science and Technology, Poland.
Learn more
Duckietown is a platform for creating and disseminating robotics and AI learning experiences.
It is modular, customizable and state-of-the-art, and designed to teach, learn, and do research. From exploring the fundamentals of computer science and automation to pushing the boundaries of knowledge, Duckietown evolves with the skills of the user.
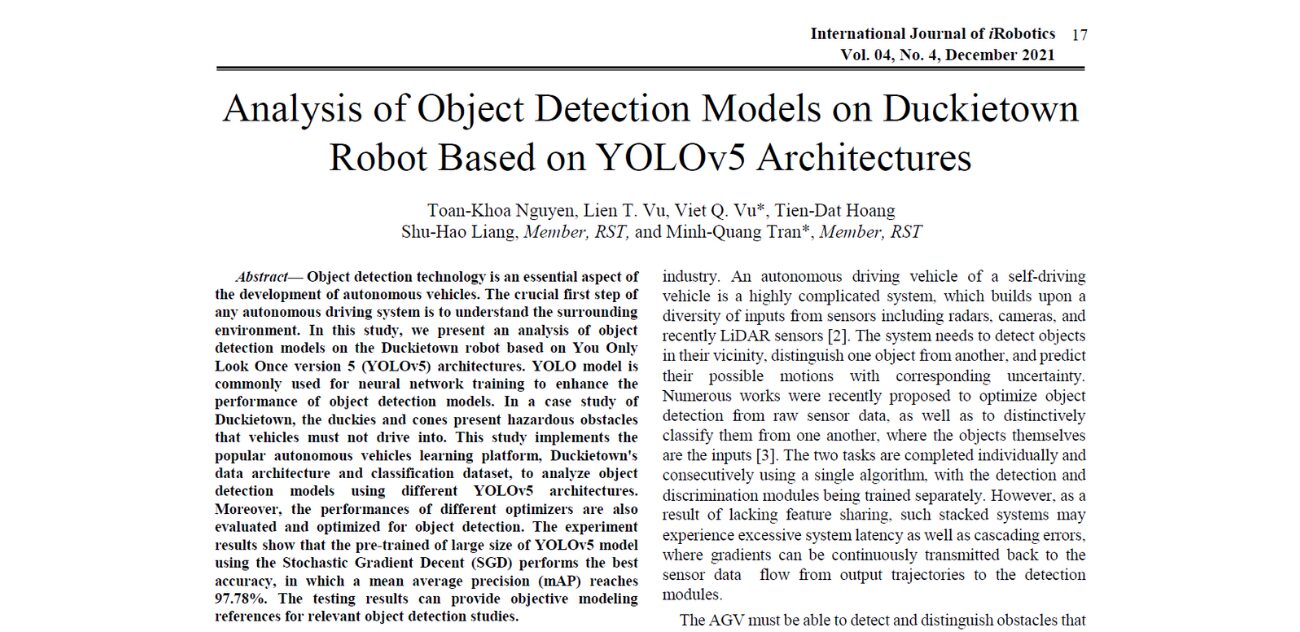
Object Detection on Duckiebots Using YOLOv5 Models
General Information
- Analysis of Object Detection Models on Duckietown Robot Based on YOLOv5 Architectures
- Toan-Khoa Nguyen, Lien T. Vu, Viet Q. Vu, TiShu-Hao Liang, en-Dat Hoang, Minh-Quang Tran.
- National Taiwan University of Science and Technology, Taiwan.
- Nguyen, T.K., Vu, L.T., Vu, V.Q., Hoang, T.D., Liang, S.H. and Tran, M.Q., 2021. Analysis of object detection models on duckietown robot based on yolov5 architectures. International Journal of iRobotics, 4(4), pp.17-22.
Object Detection on Duckiebots Using YOLOv5 Models
Obstacle detection is about having autonomous vehicles perceive their surroundings, identify objects, and determine if they might conflict with the accomplishment of the robot’s task, e.g., navigating to reach a goal position.
Amongst the many applications of AI, object detection from images is arguably the one that experienced the most performance enhancement compared to “traditional approaches” such as color or blob detection.
Images are, from the point of view of a machine, nothing but (several) “tables” of numbers, where each number represents the intensity of light, at that location, across a channel (e.g., R, G, B for colored images).
Giving meaning to a cluster of numbers is not as easy as, for a human, it would be to identify a potential obstacle on the path. Machine learning-driven approaches have quickly outperformed traditional computer vision approaches at this task, strong of the abundant and cheap data for training made available by datasets and general imagery on the internet.
Various approaches (networks) for object detection have rapidly succeded in outperforming each other, and YOLO models particularly for their balance of computational efficiency and detection accuracy.
Learn about robot autonomy, and the difference between traditional and machine learning approaches, from the links below!
Abstract
In the author’s words:
Object detection technology is an essential aspect of the development of autonomous vehicles. The crucial first step of any autonomous driving system is to understand the surrounding environment.
In this study, we present an analysis of object detection models on the Duckietown robot based on You Only Look Once version 5 (YOLOv5) architectures. YOLO model is commonly used for neural network training to enhance the performance of object detection models.
In a case study of Duckietown, the duckies and cones present hazardous obstacles that vehicles must not drive into. This study implements the popular autonomous vehicles learning platform, Duckietown’s data architecture and classification dataset, to analyze object detection models using different YOLOv5 architectures. Moreover, the performances of different optimizers are also evaluated and optimized for object detection.
The experiment results show that the pre-trained of large size of YOLOv5 model using the Stochastic Gradient Decent (SGD) performs the best accuracy, in which a mean average precision (mAP) reaches 97.78%. The testing results can provide objective modeling references for relevant object detection studies.
Highlights - Object Detection on Duckiebots Using YOLOv5 Models
Here is a visual tour of the work of the authors. For more details, check out the full paper.
Conclusion - Object Detection on Duckiebots Using YOLOv5 Models
Here are the conclusions from the authors of this paper:
“This paper presents an analysis of object detection models on the Duckietown robot based on YOLOv5 architectures. The YOLOv5 model has been successfully used to recognize the duckies and cones on the Duckietown. Moreover, the performances of different YOLOv5 architectures are analyzed and compared.
The results indicate that using the pre-trained model of YOLOv5 architecture with the SGD optimizer can provide excellent accuracy for object detection. The higher accuracy can also be obtained even with the medium size of the YOLOv5 model that enables to accelerate the computation of the system.
Furthermore, once the object detection model is optimized, it is integrated into the ROS in the Duckietown robot. In future works, it is potential to investigate the YOLOv5 with Layer-wise Adaptive Moments Based (LAMB) optimizer instead of SGD, applying repeated augmentation with Binary Cross-Entropy (BCE), and using domain adaptation technique.”
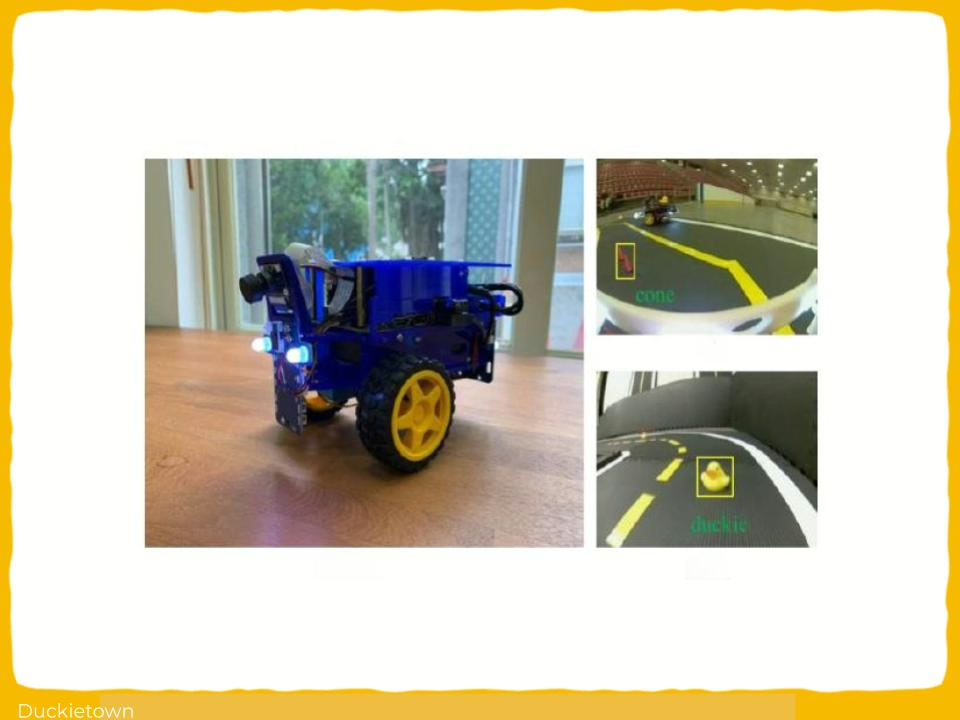
Project Authors
Toan-Khoa Nguyen is currently working as an AI engineer at FPT Software AI Center, Vietnam.
Lien T. Vu is with the Faculty of Mechanical Engineering and Mechatronics, Phenikaa University, Vietnam.
Viet Q. Vu is with the Faculty of International Training, Thai Nguyen University of Technology, Vietnam.
Tien-Dat Hoang is with the Faculty of International Training, Thai Nguyen University of Technology, Vietnam.
Shu-Hao Liang is with the Center for Cyber-Physical System Innovation, National Taiwan University of Science and Technology, Taiwan.
Minh-Quang Tran is with the Industry 4.0 Implementation Center, Center for Cyber-Physical System Innovation, National Taiwan University of Science and Technology, Taiwan and also with the Department of Mechanical Engineering, Thai Nguyen University of Technology, Vietnam.
Learn more
Duckietown is a platform for creating and disseminating robotics and AI learning experiences.
It is modular, customizable and state-of-the-art, and designed to teach, learn, and do research. From exploring the fundamentals of computer science and automation to pushing the boundaries of knowledge, Duckietown evolves with the skills of the user.
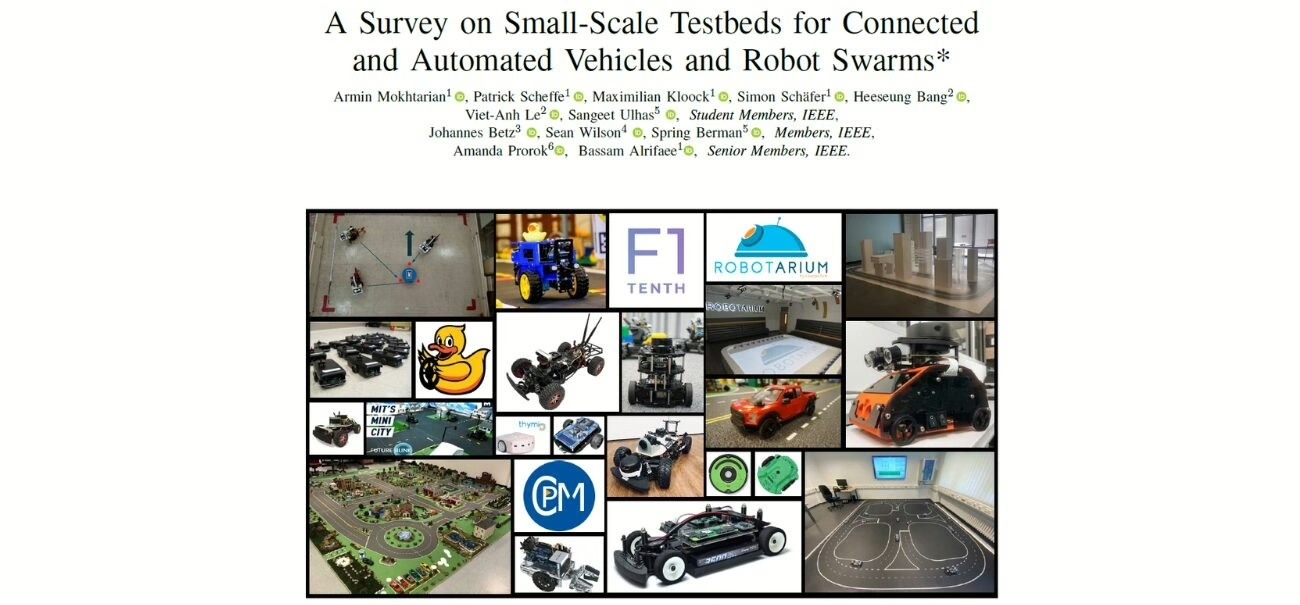
Survey on Testbeds for Vehicle Autonomy & Robot Swarms
General Information
- A Survey on Small-Scale Testbeds for Connected and Automated Vehicles and Robot Swarms
- Armin Mokhtarian, Jianye Xu, Patrick Scheffe, Maximilian Kloock, Simon Schäfer, Heeseung Bang, Viet-Anh Le, Sangeet Ulhas, Johannes Betz, Sean Wilson, Spring Berman, Liam Paull, Amanda Prorok, Bassam Alrifaee
- RWTH Aachen University, Germany
- Mokhtarian, Armin & Scheffe, Patrick & Kloock, Maximilian & Schäfer, Simon & Bang, Heeseung & Le, Viet-Anh & Sankaramangalam Ulhas, Sangeet & Betz, Johannes & Wilson, Sean & Berman, Spring & Prorok, Amanda & Alrifaee, Bassam. (2024). A Survey on Small-Scale Testbeds for Connected and Automated Vehicles and Robot Swarms. 10.13140/RG.2.2.16176.74248/1.
Survey on Testbeds for Vehicle Autonomy & Robot Swarms
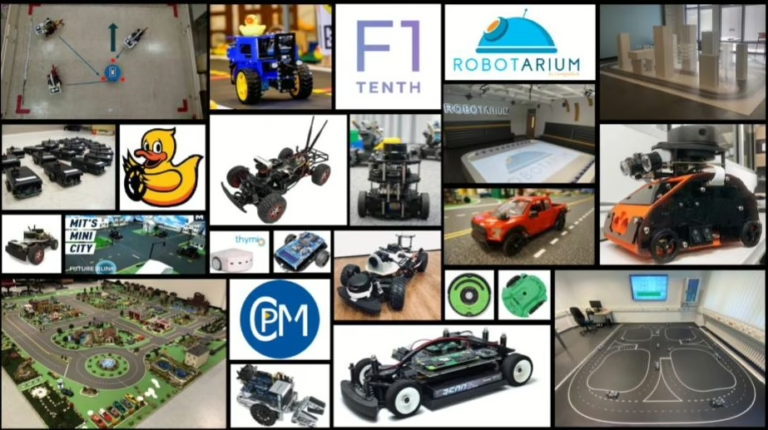
“A Survey on Small-Scale Testbeds for Connected and Automated Vehicles and Robot Swarms“ by Armin Mokhtarian et al. offers a comparison of current small-scale testbeds for Connected and Automated Vehicles (CAVs), Vehicle Autonomy and Robot Swarms (RS).
As mentioned in , small-scale autonomous vehicle testbeds are paving the way to faster and more meaningful research and development in vehicle autonomy, embodied AI, and AI robotics as a whole.
Although small-scale, often made of off-the-shelf components and relatively low-cost, these platforms provide the opportunity for deep insights into specific scientific and technological challenges of autonomy.
Duckietown, in particular, is highlighted for its modular, miniature-scale smart-city environment, which facilitates the study of autonomous vehicle localization and traffic management through onboard sensors.
Learn about robot autonomy, traditional robotics autonomy architectures, agent training, sim2real, navigation, and other topics with Duckietown, starting from the link below!
Abstract
Connected and Automated Vehicles (CAVs) and Robot Swarms (RS) have the potential to transform the transportation and manufacturing sectors into safer, more efficient, sustainable systems.
However, extensive testing and validation of their algorithms are required. Small-scale testbeds offer a cost-effective and controlled environment for testing algorithms, bridging the gap between full-scale experiments and simulations. This paper provides a structured overview of characteristics of testbeds based on the sense-plan-act paradigm, enabling the classification of existing testbeds.
Its aim is to present a comprehensive survey of various testbeds and their capabilities. We investigated 17 testbeds and present our results on the public webpage www.cpm-remote.de/testbeds.
Furthermore, this paper examines seven testbeds in detail to demonstrate how the identified characteristics can be used for classification purposes.
Highlights - Survey on Testbeds for Vehicle Autonomy & Robot Swarms
Here is a visual tour of the authors’ work. For more details, check out the full paper or the corresponding up-to-date project website.
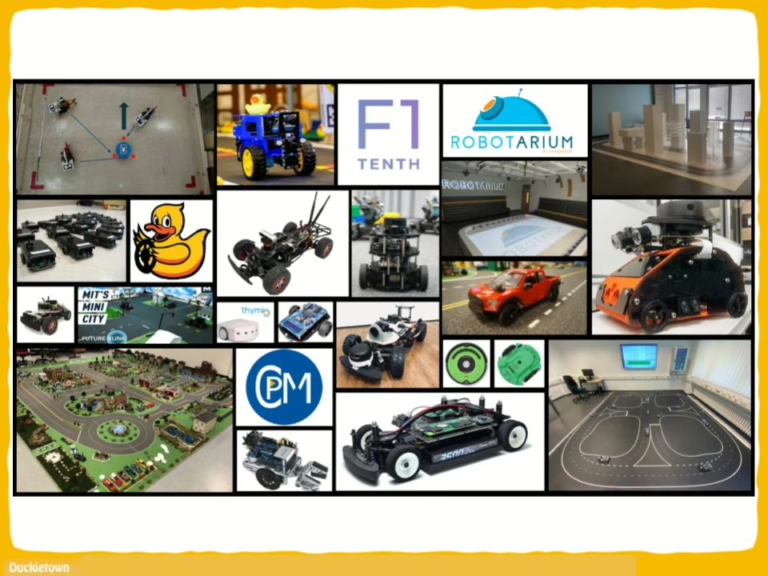
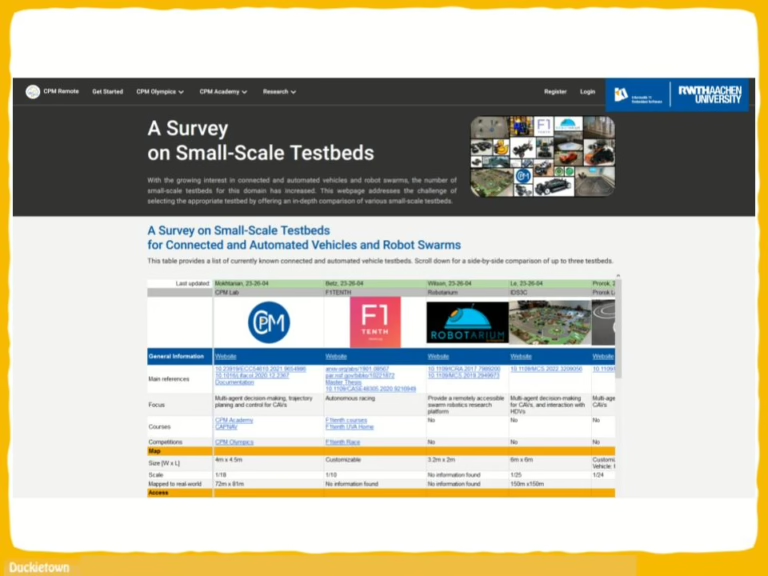
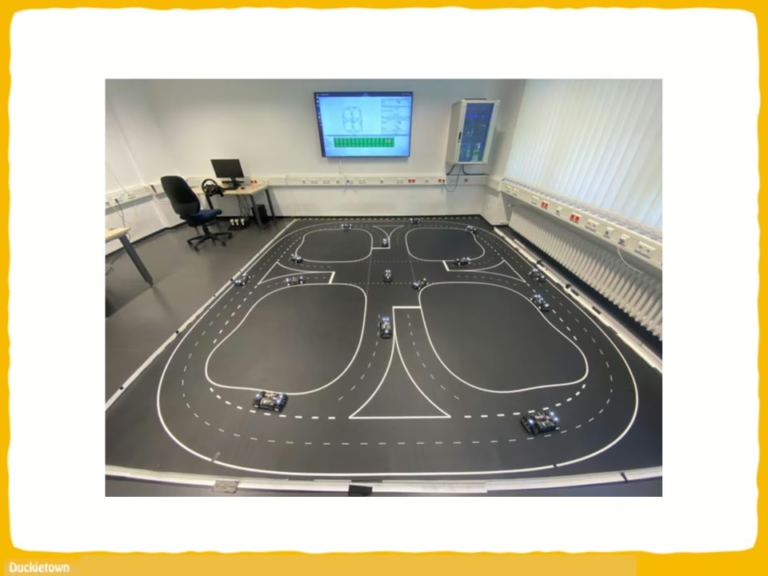
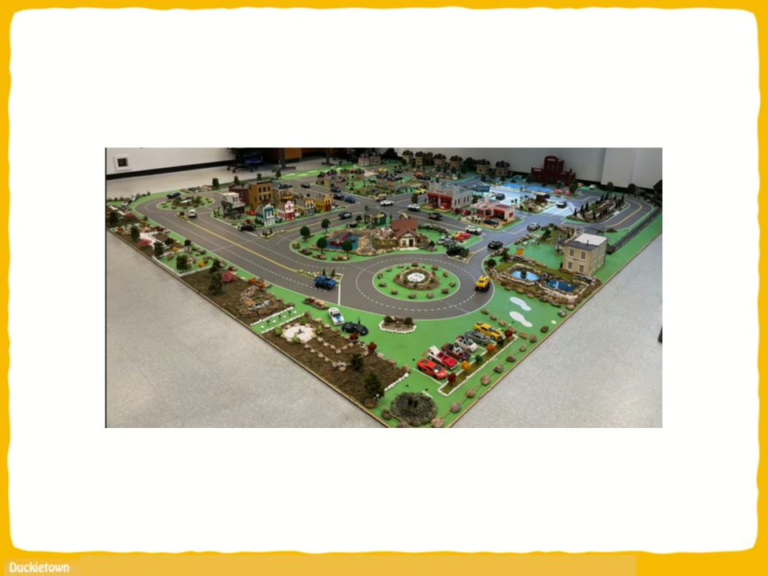
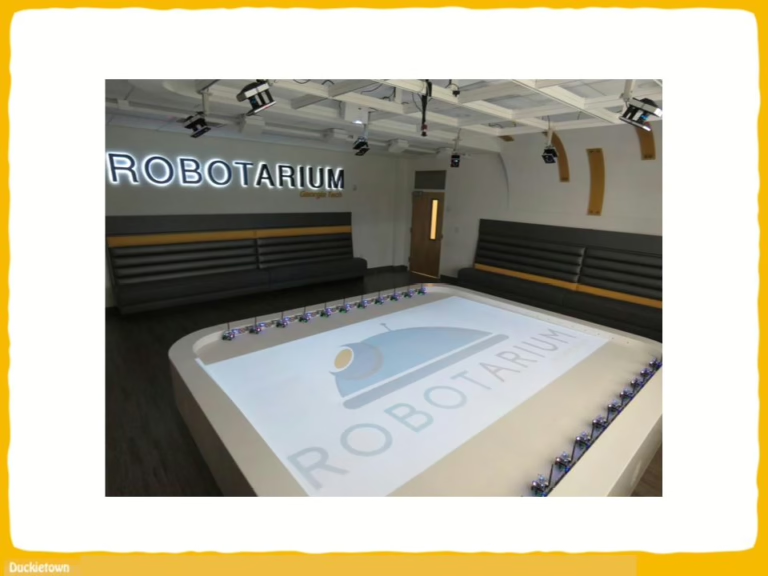
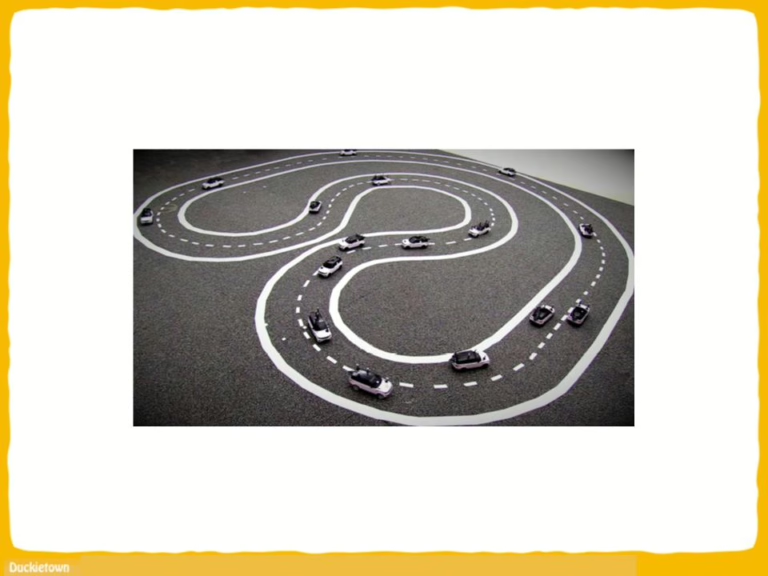
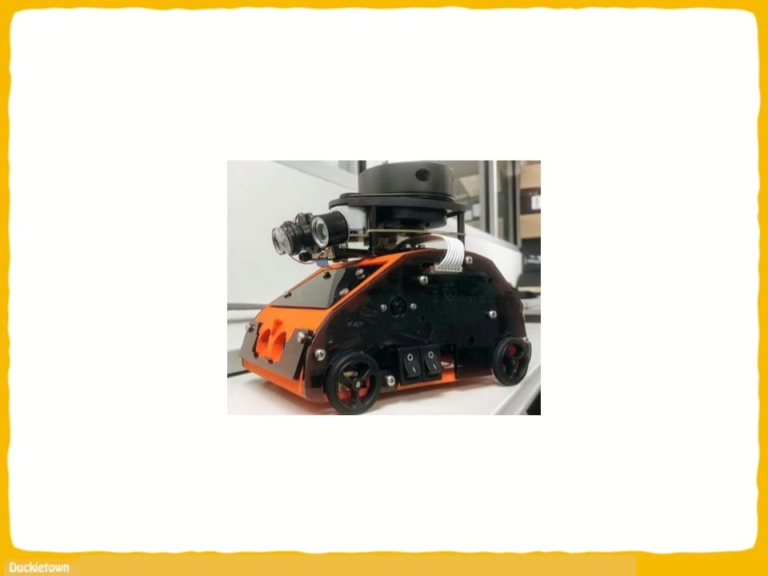
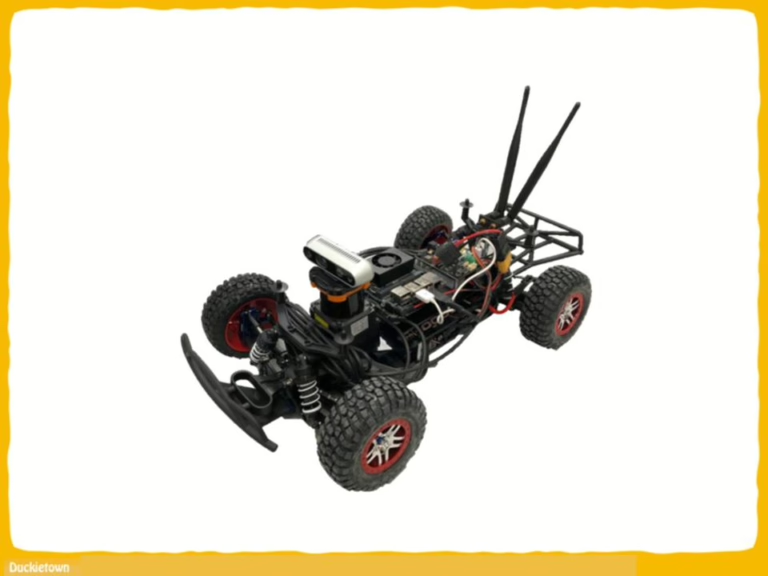
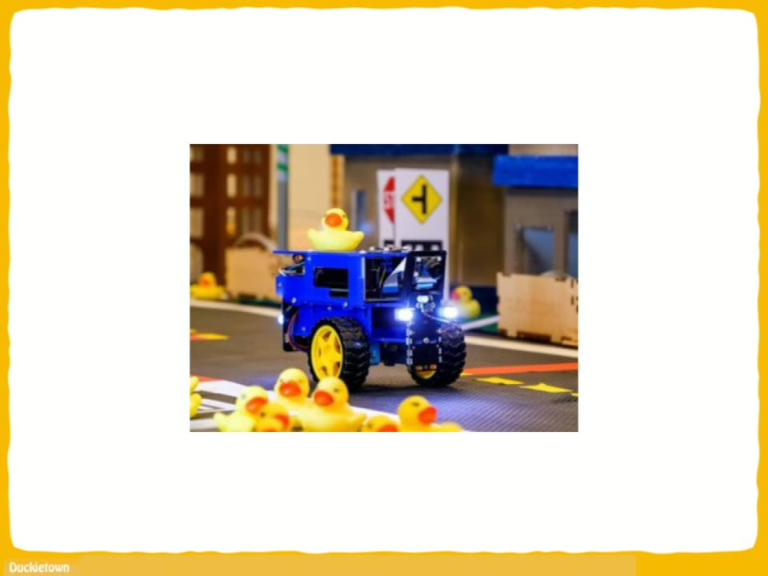
Conclusion - Survey on Testbeds for Vehicle Autonomy & Robot Swarms
Here are the conclusions from the authors of this paper:
“This survey provides a detailed overview of small-scale CAV/RS testbeds, with the aim of helping researchers in these fields to select or build the most suitable testbed for their experiments and to identify potential research focus areas. We structured the survey according to characteristics derived from potential use cases and research topics within the sense-plan-act paradigm.
Through an extensive investigation of 17 testbeds, we have evaluated 56 characteristics and have made the results of this analysis available on our webpage. We invited the testbed creators to assist in the initial process of gathering information and updating the content of this webpage. This collaborative approach ensures that the survey maintains its relevance and remains up to date with the latest developments.
The ongoing maintenance will allow researchers to access the most recent information. In addition, this paper can serve as a guide for those interested in creating a new testbed. The characteristics and overview of the testbeds presented in this survey can help identify potential gaps and areas for improvement.
One ongoing challenge that we identified with small-scale testbeds is the enhancement of their ability to accurately map to realworld conditions, ensuring that experiments conducted are as realistic and applicable as possible.
Overall, this paper provides a resource for researchers and developers in the fields of connected and automated vehicles and robot swarms, enabling them to make informed decisions when selecting or replicating a testbed and supporting the advancement of testbed technologies by identifying research gaps.”
Project Authors
Armin Mokhtarian is currently working as a Research Associate & PhD Candidate at RWTH Aachen University, Germany.
Patrick Scheffe is a Research Associate at Lehrstuhl Informatik 11 – Embedded Software, Germany.
Maximilian Kloock is working as a Team Manager Advanced Battery Management System Technologies at FEV Europe, Germany.
Simon Schäfer is a Visiting Researcher at Faculty of Engineering, University of Alberta, Canada.
Heeseung Bang is currently a Postdoctoral Associate at Cornell University, USA.
Viet-Anh Le is a Visiting Graduate Student at Cornell University, USA.
Sangeet Ulhas is a PhD candidate at Ira A. Fulton Schools of Engineering at Arizona State University, USA.
Johannes Betz is a Assistant Professor at Technische Universität München, Germany.
Sean Wilson is a Senior Research Engineer at Georgia Institute of Technology, USA.
Spring Berman is an Associate Professor at Arizona State University, USA.
Liam Paull is an Associate Professor at Université de Montréal, Canada and he is also the Chief Education Officer at Duckietown, USA.
Amanda Prorok is an associate professor at University of Cambridge, UK.
Bassam Alrifaee is a Professor at Bundeswehr University Munich, Germany.
Learn more
Duckietown is a platform for creating and disseminating robotics and AI learning experiences.
It is modular, customizable and state-of-the-art, and designed to teach, learn, and do research. From exploring the fundamentals of computer science and automation to pushing the boundaries of knowledge, Duckietown evolves with the skills of the user.
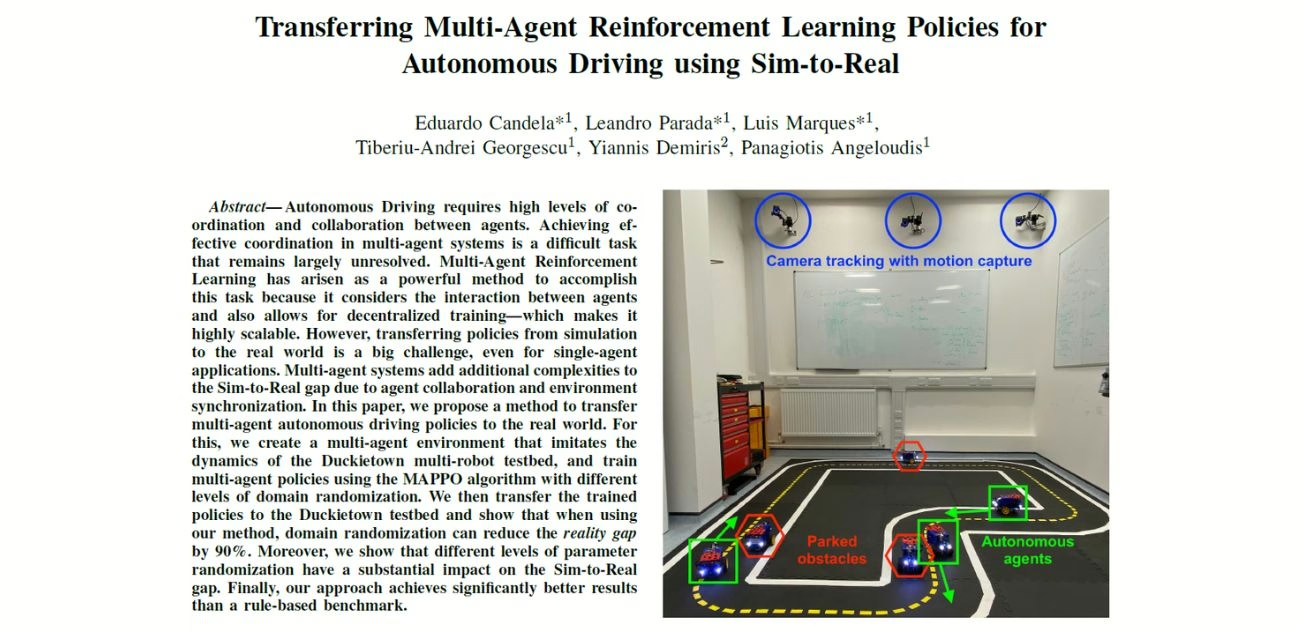
Sim2Real Transfer of Multi-Agent Policies for Self-Driving
General Information
- Transferring Multi-Agent Reinforcement Learning Policies for Autonomous Driving using Sim-to-Real
- Eduardo Candela, Leandro Parada, Luis Marques, Tiberiu-Andrei Georgescu, Yiannis Demiris, Panagiotis Angeloudis
- Imperial College London, United Kingdom
- E. Candela, L. Parada, L. Marques, T. -A. Georgescu, Y. Demiris and P. Angeloudis, "Transferring Multi-Agent Reinforcement Learning Policies for Autonomous Driving using Sim-to-Real," 2022 IEEE/RSJ International Conference on Intelligent Robots and Systems (IROS), Kyoto, Japan, 2022, pp. 8814-8820, doi: 10.1109/IROS47612.2022.9981319.
Sim2Real Transfer of Multi-Agent Policies for Self-Driving
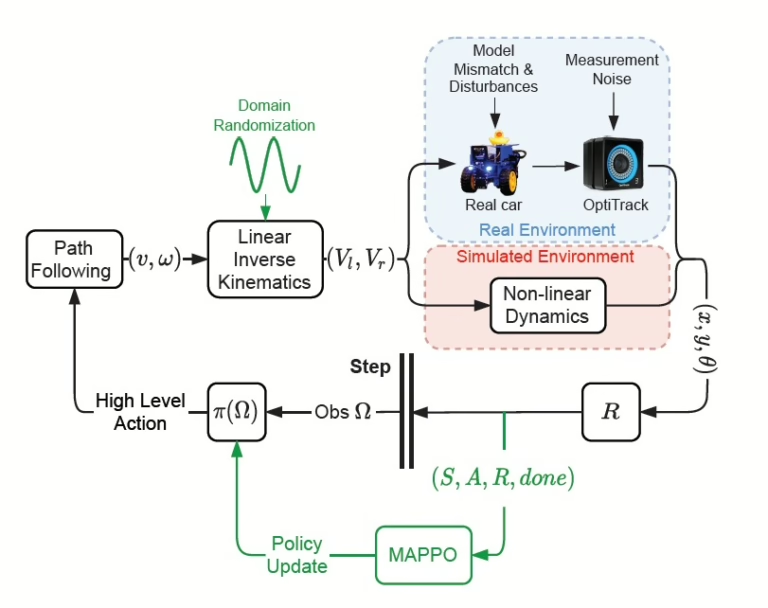
In the field of autonomous driving, transferring policies from simulation to the real world (Sim-to-real transfer, or Sim2Real) is theoretically desirable, as it is much faster and more cost-effective to train agents in simulation rather than in the real world.
Given simulations are just that – representations of the real world – the question of whether the trained policies will actually perform well enough in the real world is always open. This challenge is known as “Sim-to-Real gap”.
This gap is especially pronounced in Multi-Agent Reinforcement Learning (MARL), where agent collaboration and environmental synchronization significantly complicate policy transfer.
The authors of this work propose employing “Multi-Agent Proximal Policy Optimization” (MAPPO) in conjunction with domain randomization techniques, to create a robust pipeline for training MARL policies that is not only effective in simulation but also adaptable to real-world conditions.
Through varying levels of parameter randomization—such as altering lighting conditions, lane markings, and agent behaviors— the authors enhance the robustness of trained policies, ensuring they generalize effectively across a wide range of real-world scenarios.
Learn about training, sim2real, navigation, and other robot autonomy topics with Duckietown starting from the link below!
Abstract
Autonomous Driving requires high levels of coordination and collaboration between agents. Achieving effective coordination in multi-agent systems is a difficult task that remains largely unresolved. Multi-Agent Reinforcement Learning has arisen as a powerful method to accomplish this task because it considers the interaction between agents and also allows for decentralized training—which makes it highly scalable.
However, transferring policies from simulation to the real world is a big challenge, even for single-agent applications. Multi-agent systems add additional complexities to the Sim-to-Real gap due to agent collaboration and environment synchronization.
In this paper, we propose a method to transfer multi-agent autonomous driving policies to the real world. For this, we create a multi-agent environment that imitates the dynamics of the Duckietown multi-robot testbed, and train multi-agent policies using the MAPPO algorithm with different levels of domain randomization. We then transfer the trained policies to the Duckietown testbed and show that when using our method, domain randomization can reduce the reality gap by 90%.
Moreover, we show that different levels of parameter randomization have a substantial impact on the Sim-to-Real gap. Finally, our approach achieves significantly better results than a rule-based benchmark.
Highlights - Sim2Real Transfer of Multi-Agent Policies for Self-Driving
Here is a visual tour of the work of the authors. For more details, check out the full paper.
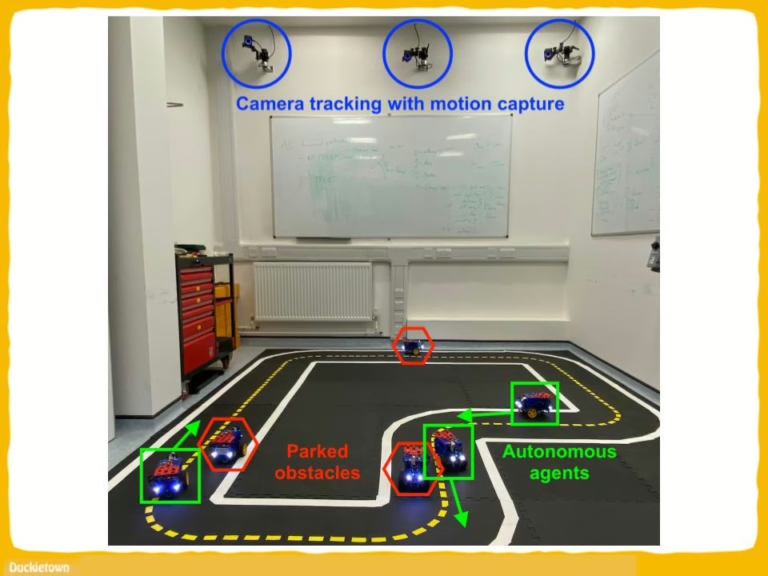
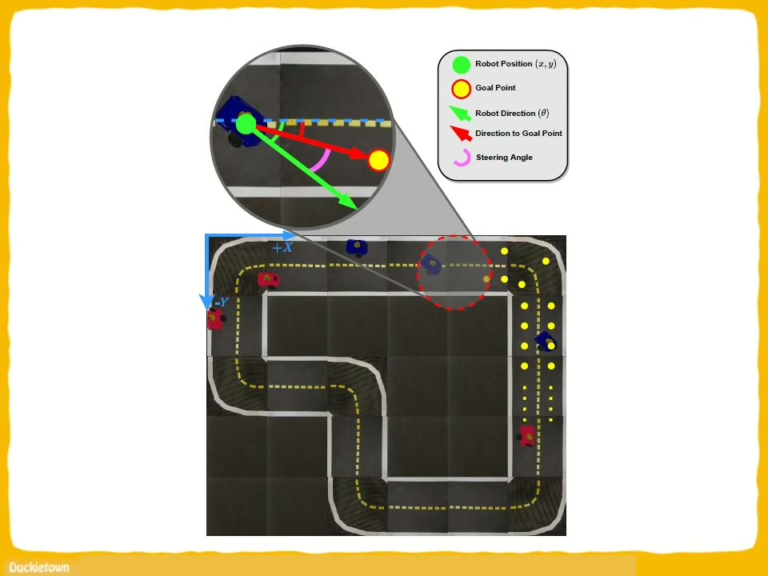
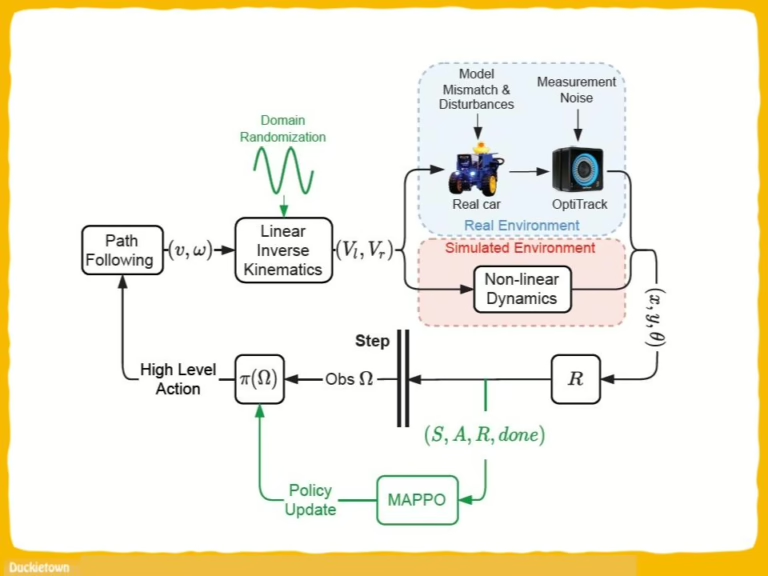
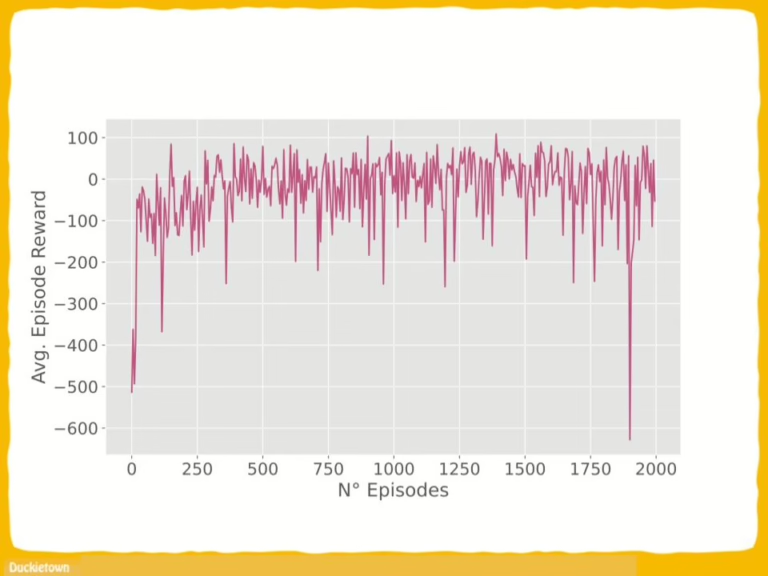
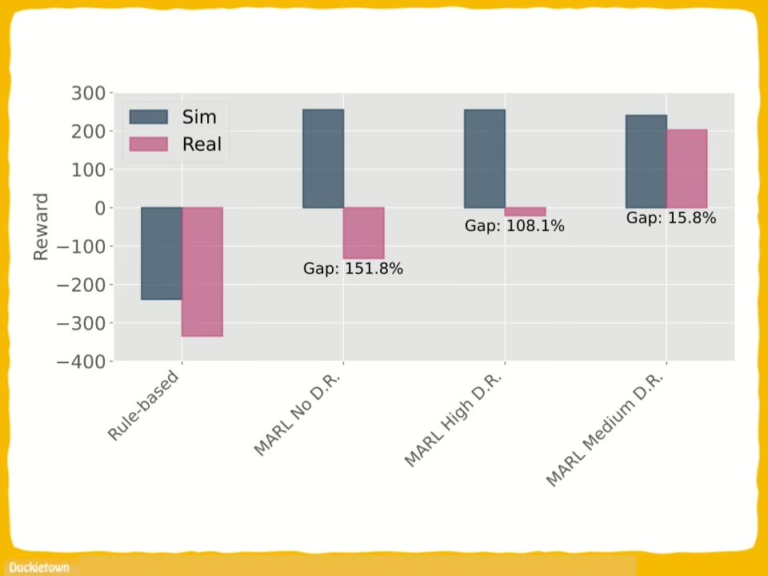
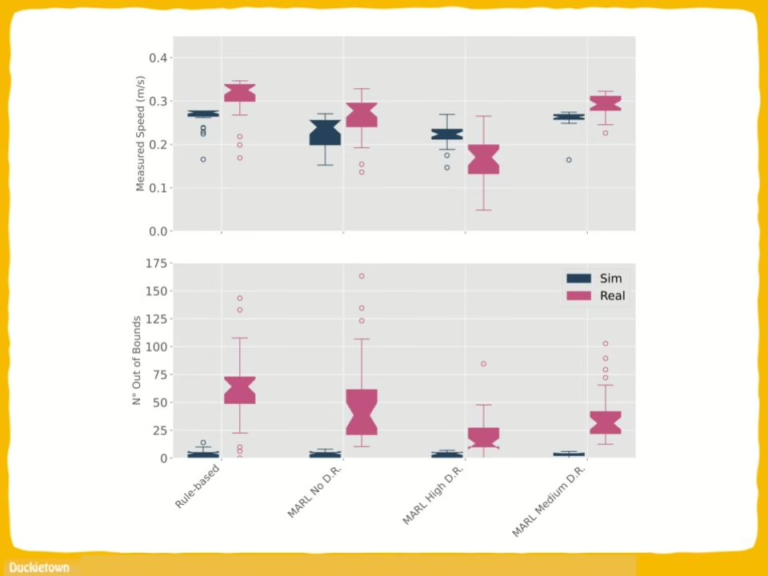
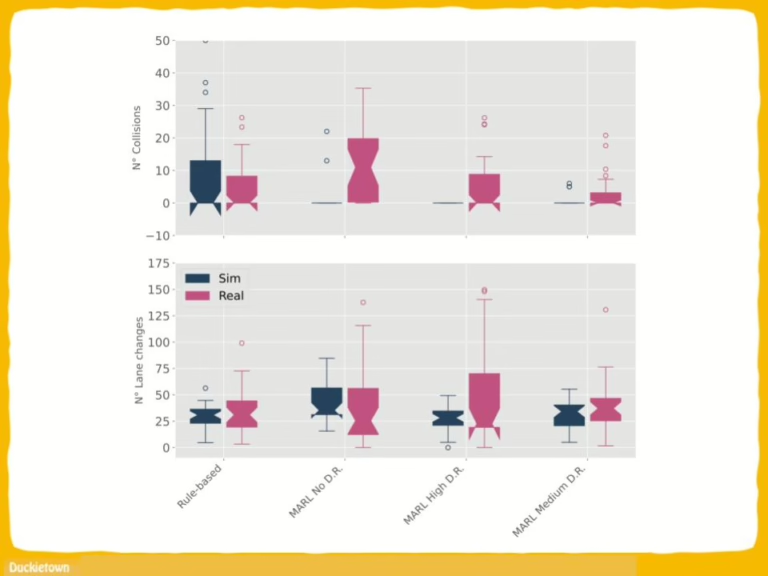
Conclusion - Sim2Real Transfer of Multi-Agent Policies for Self-Driving
Here are the conclusions from the authors of this paper:
“AVs will lead to enormous safety and efficiency benefits across multiple fields, once the complex problem of multiagent coordination and collaboration is solved. MARL can help towards this, as it enables agents to learn to collaborate by sharing observations and rewards.
However, the successful application of MARL, is heavily dependent on the fidelity of the simulation environment they were trained in. We present a method to train policies using MARL and to reduce the reality gap when transferring them to the real world via adding domain randomization during training, which we show has a significant and positive impact in real performance compared to rule-based methods or policies trained without different levels of domain randomization.
It is important to mention that despite the performance improvements observed when using domain randomization, its use presents diminishing returns as seen with the overly conservative policy, for it cannot completely close the reality gap without increasing the fidelity of the simulator. Additionally, the amount of domain randomization to be used is case-specific and a theory for the selection of domain randomization remains an open question. The quantification and description of reality gaps presents another opportunity for future research.”
Project Authors
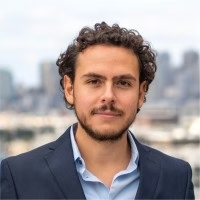
Eduardo Candela is currently working as the Co-Founder & CTO of MAIHEM (YC W24), California.
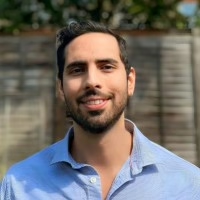
Leandro Parada is a Research Associate at Imperial College London, United Kingdom.
Luís Marques is a Doctoral Researcher in the Department of Robotics at the University of Michigan, USA.
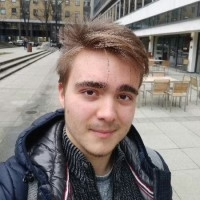
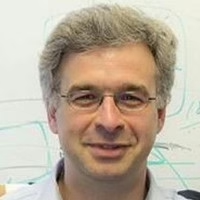
Yiannis Demiris is a Professor of Human-Centred Robotics and Royal Academy of Engineering Chair in Emerging Technologies at Imperial College London, United Kingdom.
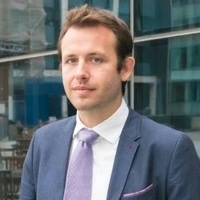
Panagiotis Angeloudis is a Reader in Transport Systems and Logistics at Imperial College London, United Kingdom.
Learn more
Duckietown is a platform for creating and disseminating robotics and AI learning experiences.
It is modular, customizable and state-of-the-art, and designed to teach, learn, and do research. From exploring the fundamentals of computer science and automation to pushing the boundaries of knowledge, Duckietown evolves with the skills of the user.
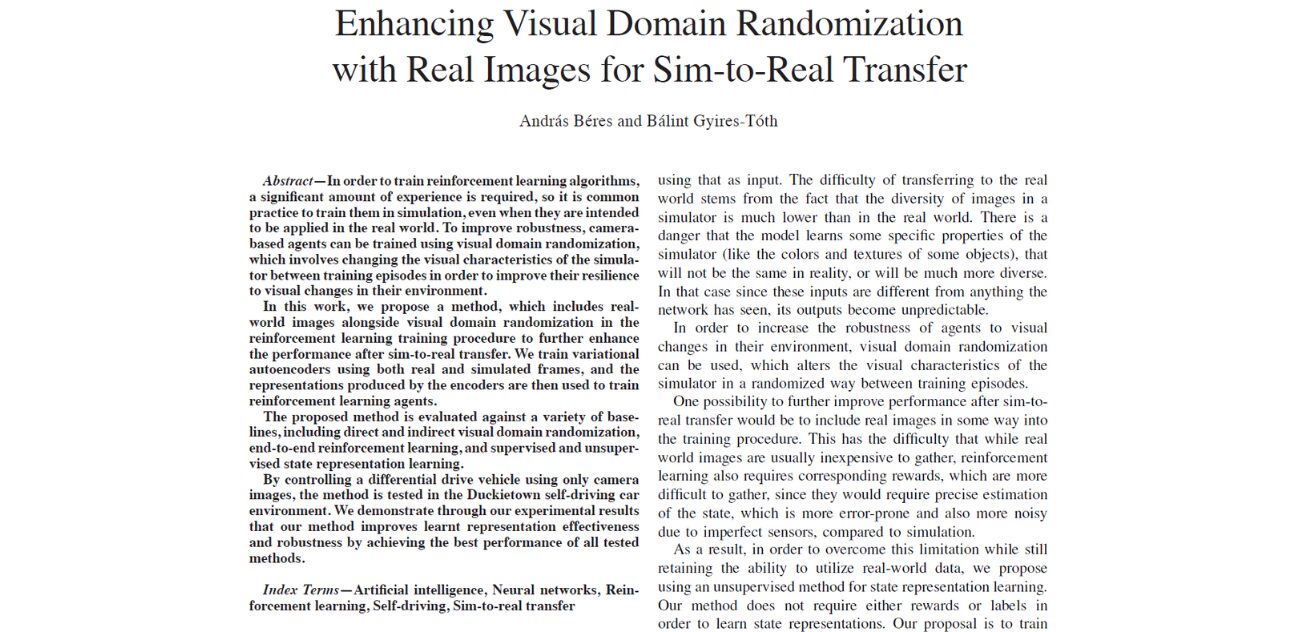
Enhancing Visual Domain Randomization for Sim2Real Transfer
General Information
- Enhancing Visual Domain Randomization with Real Images for Sim-to-Real Transfer
- András Béres and Bálint Gyires-Tóth
- Budapest University of Technology and Economics, Hungary
- Béres, András and Gyires-Tóth, Bálint (2023) Enhancing Visual Domain Randomization with Real Images for Sim-to-Real Transfer. INFOCOMMUNICATIONS JOURNAL, 15 (1). pp. 15-25. ISSN 2061-2079
Enhancing Visual Domain Randomization with Real Images for Sim-to-Real Transfer
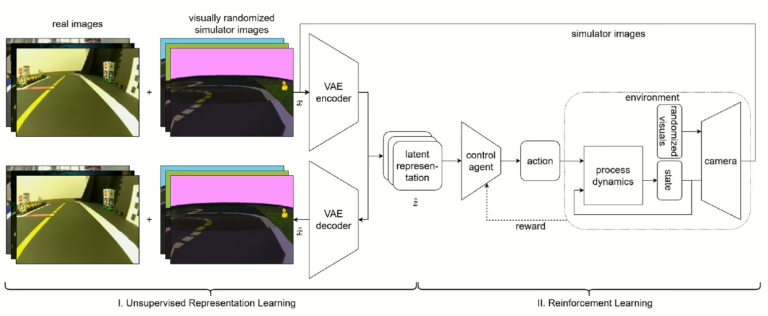
One of the classical objections made to machine learning approaches to embeddded autonomy (i.e., to create agents that are deployed on real, physical, robots) is that training requires data, data requires experiement, and experiment are “expensive” (time, money, etc.).
The natural counter argument to this is to use simulation to create the training data, because simulations are much less expensive than real world experiment; they can be ran continuously, with accellerated time, don’t require supervision, nobody gets tired, etc.
But, as the experienced roboticist knows, “simulations are doomed to succeed”. This phrase encapsulates the notion that simulations do not contain the same wealth if information as the real world, because they are programmed to be what the programmer wants them to be useful for – they do not capture the complexity of the real world. Eventually things will “work” in simulation, but does that mean they will “work” in the real-world, too?
As Carl Sagan once said: “If you wish to make an applie pie from scratch, you must first reinvent the universe”.
Domain randomization is an approach to mitigate the limitations of simulations. Instead of training an agent on one set of parameters defining the simulation, many simulations are instead ran, with different values of this parameters. E.g., in the context of a driving simulator like Duckietown, one set of parameters could make the sky purple instead of blue, or the lane markings have slightly different geometric properties, etc. The idea behind this approach is that the agent will be trained on a distribution of datasets that are all slightly different, hopefully making the agent more robust to real world nuisances once deployed in a physical body.
In this paper, the authors investigate specifically visual domain randomization.
Learn about RL, navigation, and other robot autonomy topics at the link below!
Abstract
In order to train reinforcement learning algorithms, a significant amount of experience is required, so it is common practice to train them in simulation, even when they are intended to be applied in the real world. To improve robustness, camerabased agents can be trained using visual domain randomization, which involves changing the visual characteristics of the simulator between training episodes in order to improve their resilience to visual changes in their environment.
In this work, we propose a method, which includes realworld images alongside visual domain randomization in the reinforcement learning training procedure to further enhance the performance after sim-to-real transfer. We train variational autoencoders using both real and simulated frames, and the representations produced by the encoders are then used to train reinforcement learning agents.
The proposed method is evaluated against a variety of baselines, including direct and indirect visual domain randomization, end-to-end reinforcement learning, and supervised and unsupervised state representation learning.
By controlling a differential drive vehicle using only camera images, the method is tested in the Duckietown self-driving car environment. We demonstrate through our experimental results that our method improves learnt representation effectiveness and robustness by achieving the best performance of all tested methods.
Highlights - Enhancing Visual Domain Randomization with Real Images for Sim-to-Real Transfer
Here is a visual tour of the work of the authors. For more details, check out the full paper.
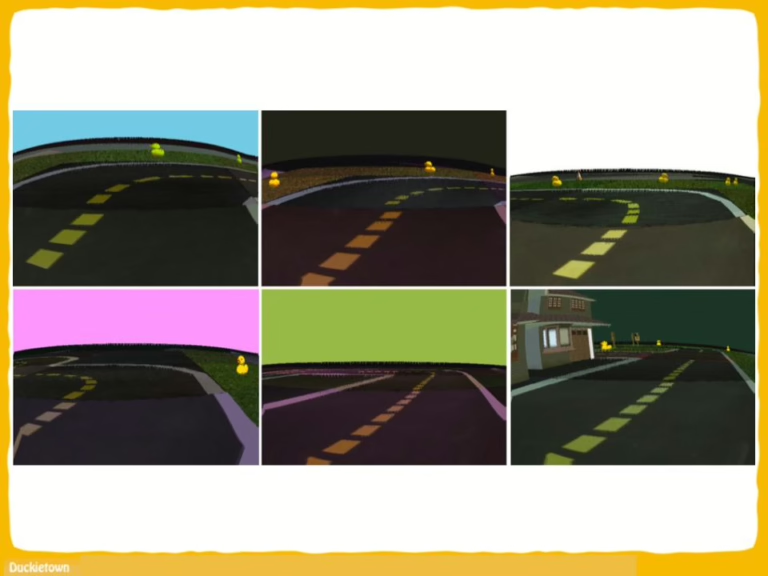
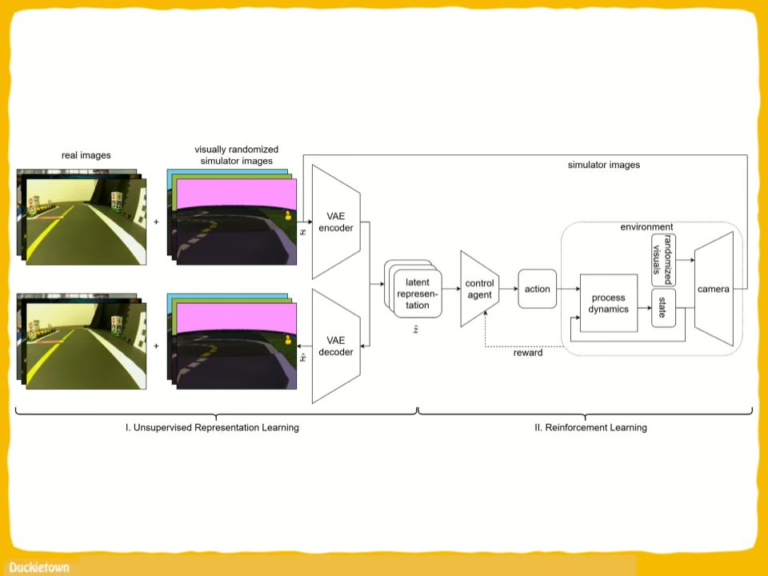
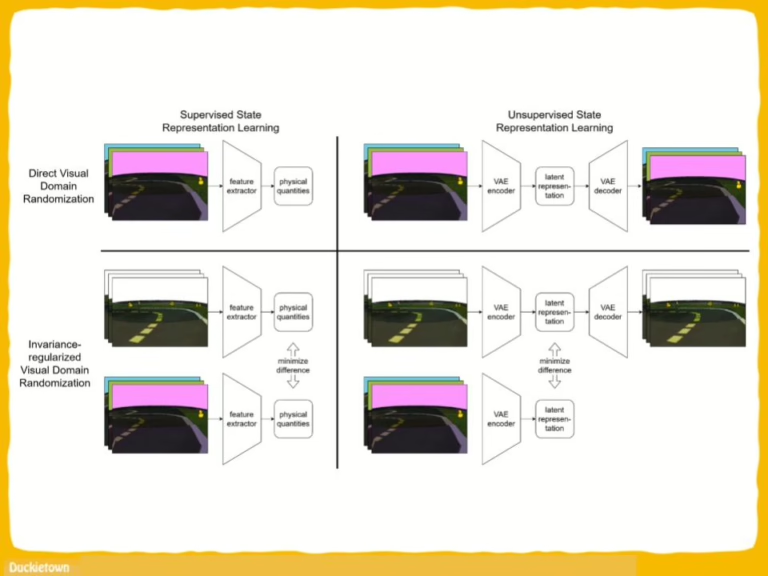
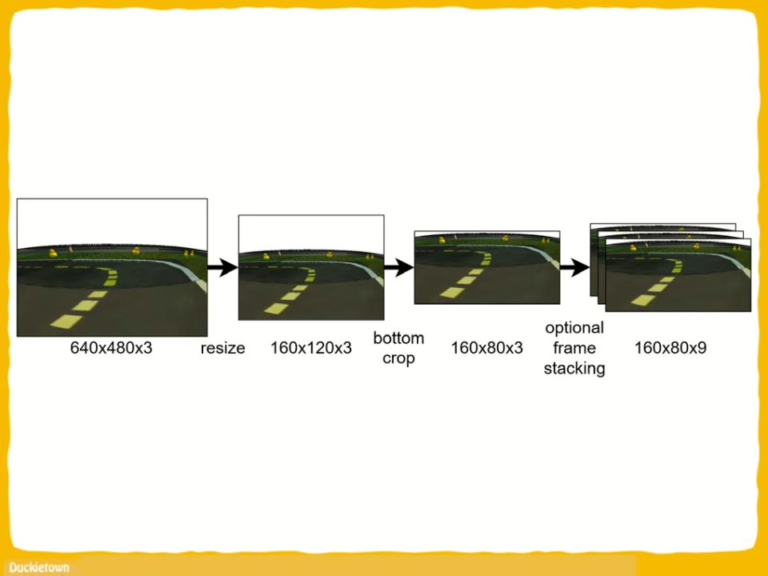
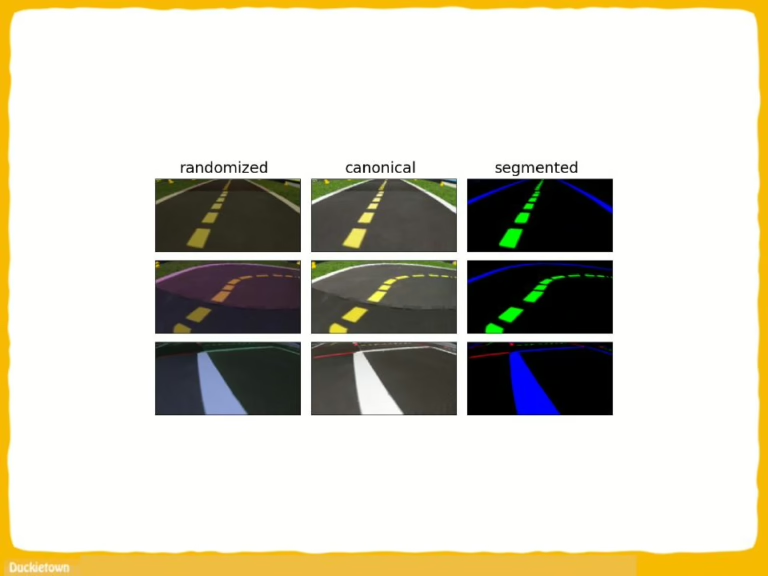
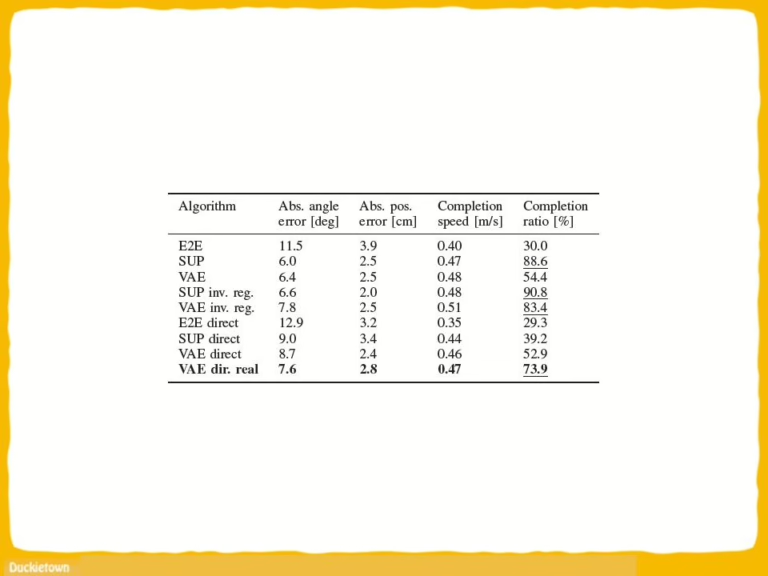
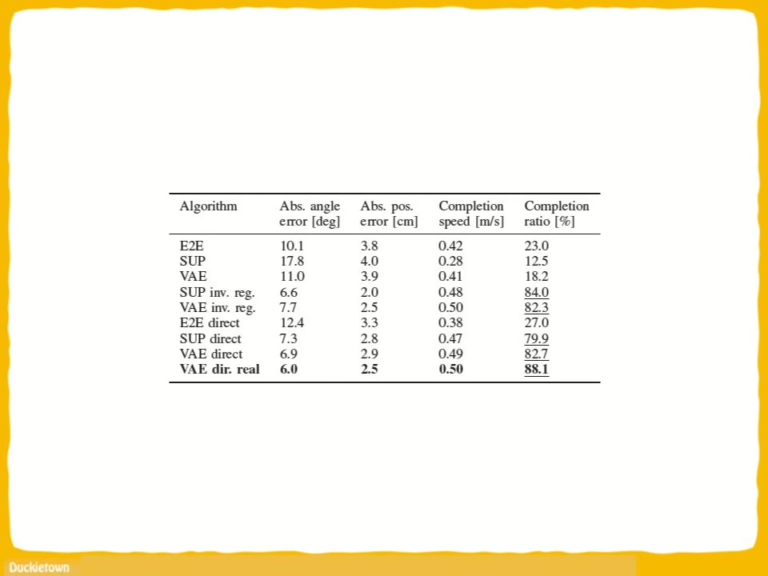
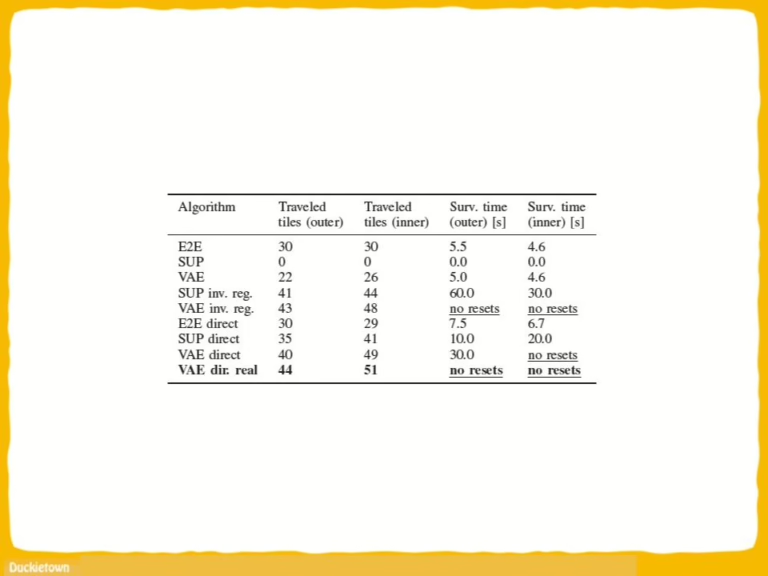
Conclusion - Enhancing Visual Domain Randomization with Real Images for Sim-to-Real Transfer
Here are the conclusions from the authors of this paper:
“In this work we proposed a novel method for learning effective image representations for reinforcement learning, whose core idea is to train a variational autoencoder using visually randomized images from the simulator, but include images from the real world as well, as if it was just another visually different version of the simulator.
We evaluated the method in the Duckietown self-driving environment on the lane-following task, and our experimental results showed that the image representations of our proposed method improved the performance of the tested reinforcement learning agents both in simulation and reality. This demonstrates the effectiveness and robustness of the representations learned by the proposed method. We benchmarked our method against a wide range of baselines, and the proposed method performed among the best in all cases.
Our experiments showed that using some type of visual domain randomization is necessary for a successful simto- real transfer. Variational autoencoder-based representations tended to outperform supervised representations, and both outperformed representations learned during end-to-end reinforcement learning. Also, for visual domain randomization, when using no real images, invariance regularization-based methods seemed to outperform direct methods. Based on our results, we conclude that including real images in simulation-based reinforcement learning trainings is able to enhance the real world performance of the agent – when using the two-stage approach, proposed in this paper.”
Project Authors
András Béres is currently working as a Junior Deep Learning Engineer at Continental, Hungary.
Bálint Gyires-Tóth is an associate professor at
Budapest University of Technology and Economics, Hungary.
Learn more
Duckietown is a platform for creating and disseminating robotics and AI learning experiences.
It is modular, customizable and state-of-the-art, and designed to teach, learn, and do research. From exploring the fundamentals of computer science and automation to pushing the boundaries of knowledge, Duckietown evolves with the skills of the user.
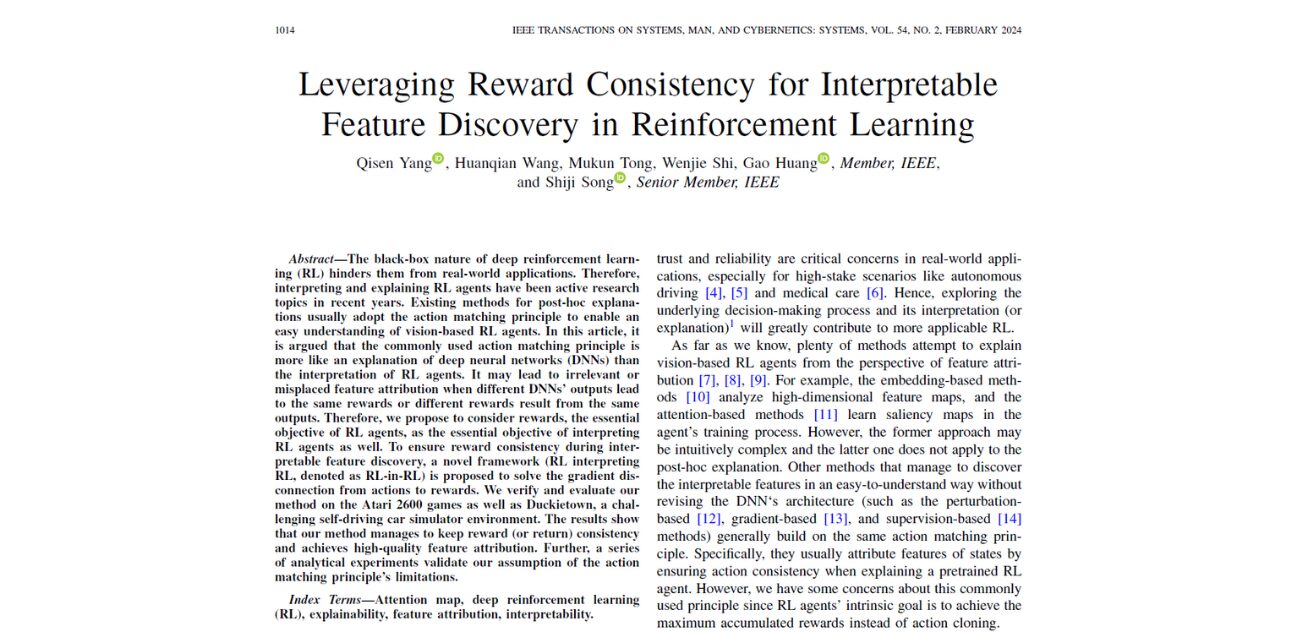
Reward Consistency for Interpretable Feature Discovery in RL
General Information
- Leveraging Reward Consistency for Interpretable Feature Discovery in Reinforcement Learning
- Qisen Yang, Huanqian Wang, Mukun Tong, Wenjie Shi, Gao Huang, and Shiji Song
- Tsinghua University, China
- Q. Yang, H. Wang, M. Tong, W. Shi, G. Huang and S. Song, "Leveraging Reward Consistency for Interpretable Feature Discovery in Reinforcement Learning," in IEEE Transactions on Systems, Man, and Cybernetics: Systems, vol. 54, no. 2, pp. 1014-1025, Feb. 2024, doi: 10.1109/TSMC.2023.3312411.
Leveraging Reward Consistency for Interpretable Feature Discovery in Reinforcement Learning
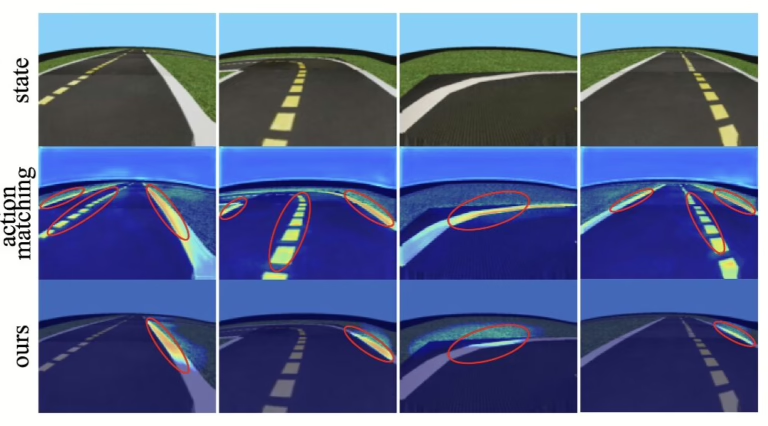
What is interpretable feature discovery in reinforcement learning?
To understand this, let’s introduce a few important topics:
Reinforcement Learning (RL): A machine learning approach where an agent gains the ability to make decisions by engaging with an environment to accomplish a specific objective. Interpretable Feature Discovery in RL is an approach that aims to make the decision-making process of RL agents more understandable to humans.
The need for interpretability: In real-world applications, especially in safety-critical domains like self-driving cars, it is crucial to understand why an RL agent makes a certain decision. Interpretability helps:
- Build trust in the system
- Debug and improve the model
- Ensure compliance with regulations and ethical standards
- Understand fault if accidents arise
Feature discovery: Feature discovery in this context refers to identifying the key artifacts (features) of the environment that the RL agent is focusing on while making decisions. For example, in a self-driving car simulation, relevant features might include the position of other cars, road signs, or lane markings.
Learn about RL, navigation, and other robot autonomy topics at the link below!
Abstract
The black-box nature of deep reinforcement learning (RL) hinders them from real-world applications. Therefore, interpreting and explaining RL agents have been active research topics in recent years. Existing methods for post-hoc explanations usually adopt the action matching principle to enable an easy understanding of vision-based RL agents. In this article, it is argued that the commonly used action matching principle is more like an explanation of deep neural networks (DNNs) than the interpretation of RL agents.
It may lead to irrelevant or misplaced feature attribution when different DNNs’ outputs lead to the same rewards or different rewards result from the same outputs. Therefore, we propose to consider rewards, the essential objective of RL agents, as the essential objective of interpreting RL agents as well. To ensure reward consistency during interpretable feature discovery, a novel framework (RL interpreting RL, denoted as RL-in-RL) is proposed to solve the gradient disconnection from actions to rewards.
We verify and evaluate our method on the Atari 2600 games as well as Duckietown, a challenging self-driving car simulator environment. The results show that our method manages to keep reward (or return) consistency and achieves high-quality feature attribution. Further, a series of analytical experiments validate our assumption of the action matching principle’s limitations.
Highlights - Leveraging Reward Consistency for Interpretable Feature Discovery in Reinforcement Learning
Here is a visual tour of the work of the authors. For more details, check out the full paper.
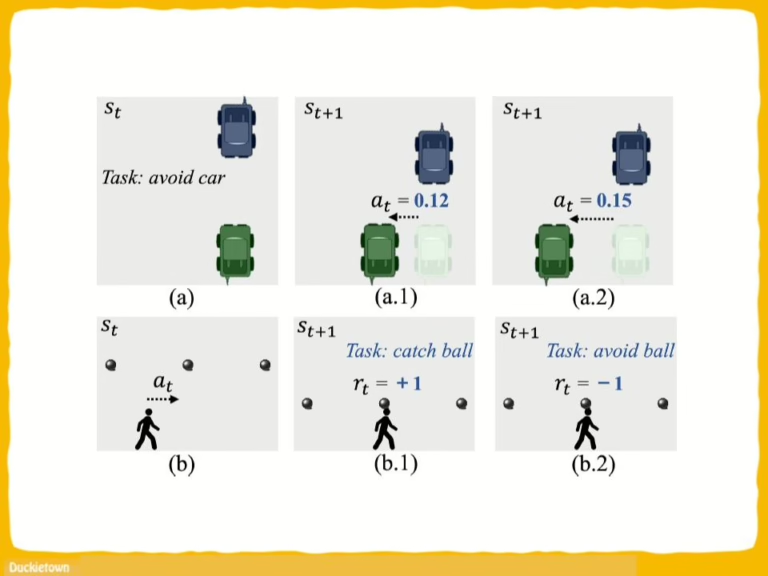
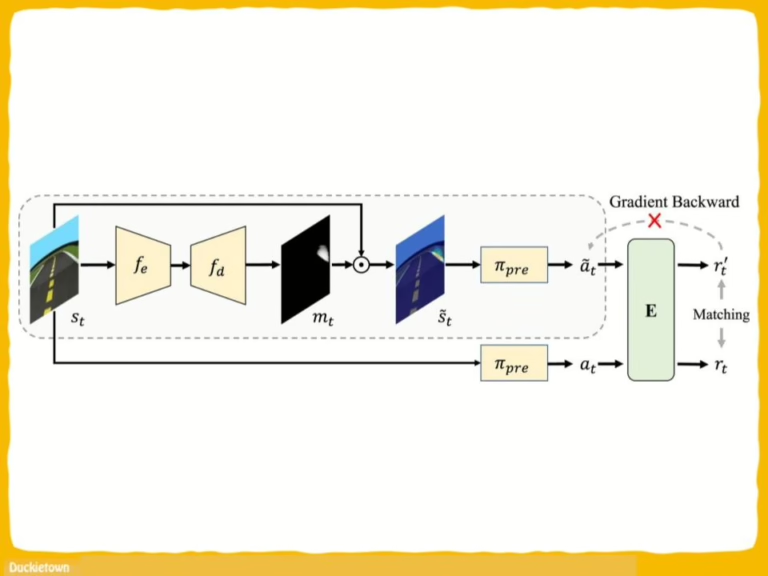
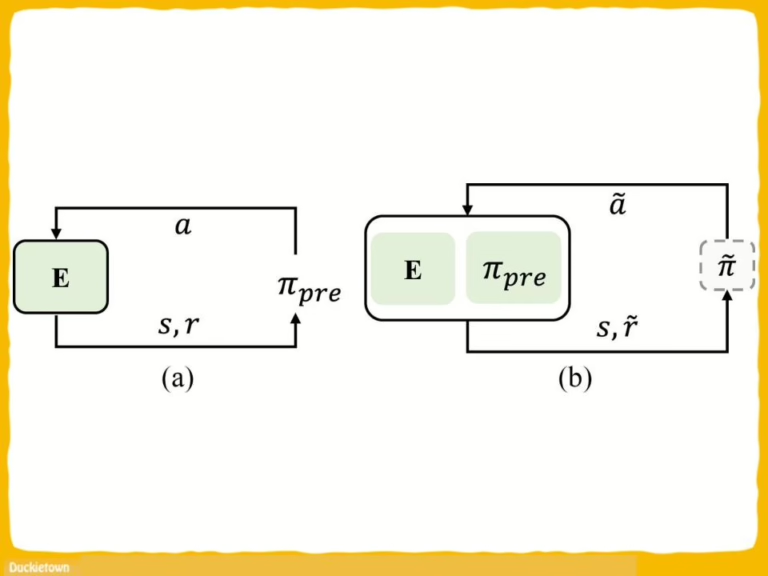
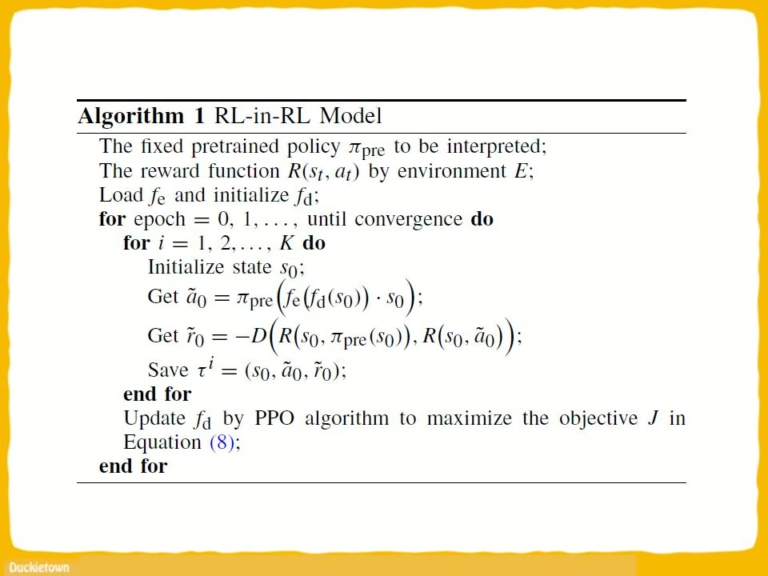
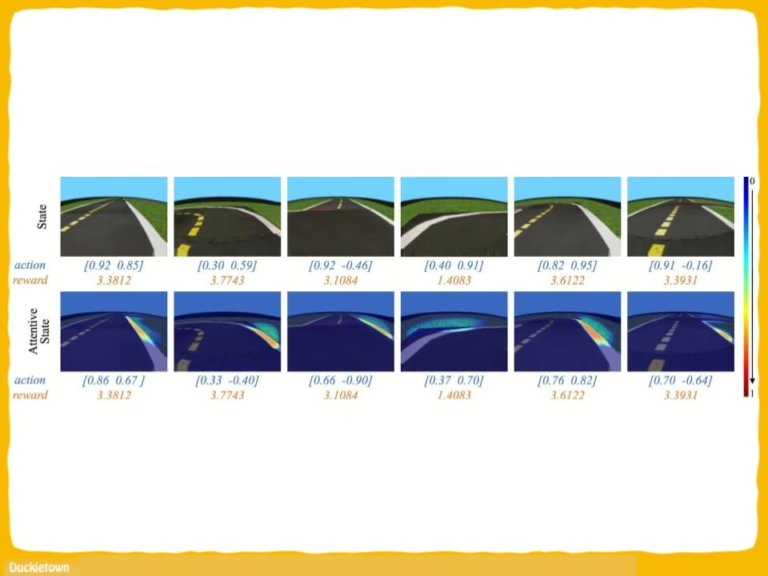
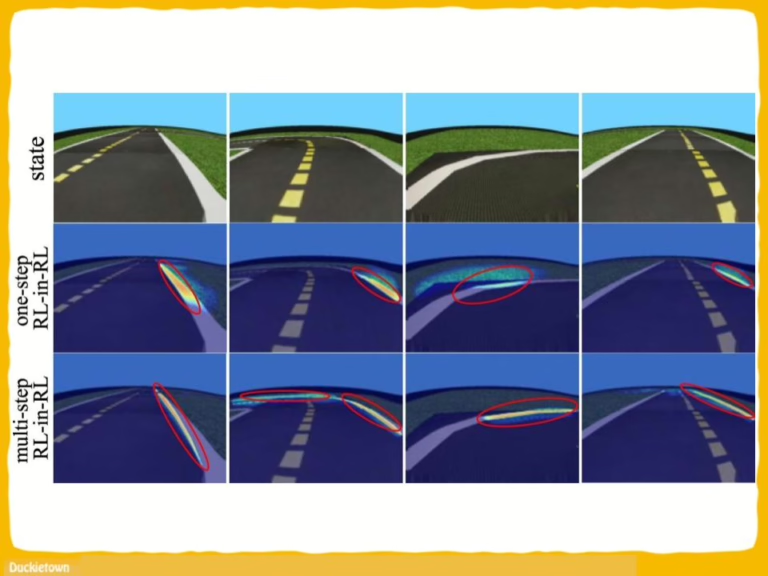
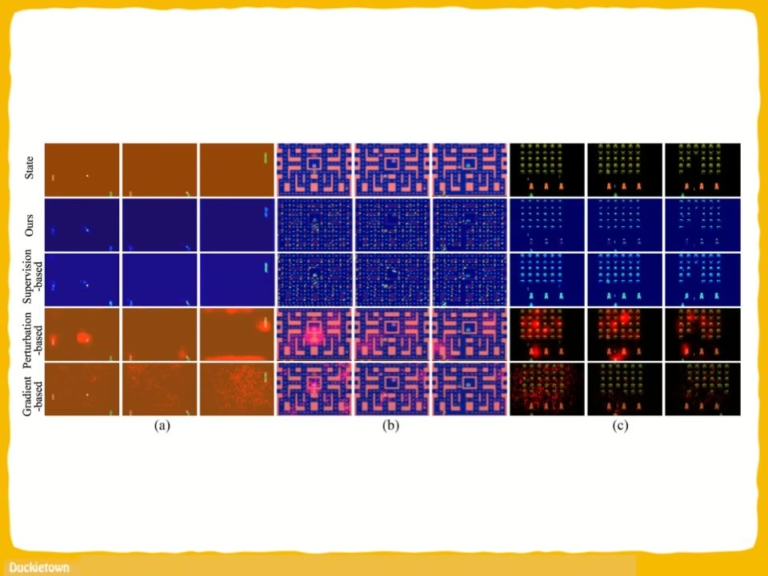
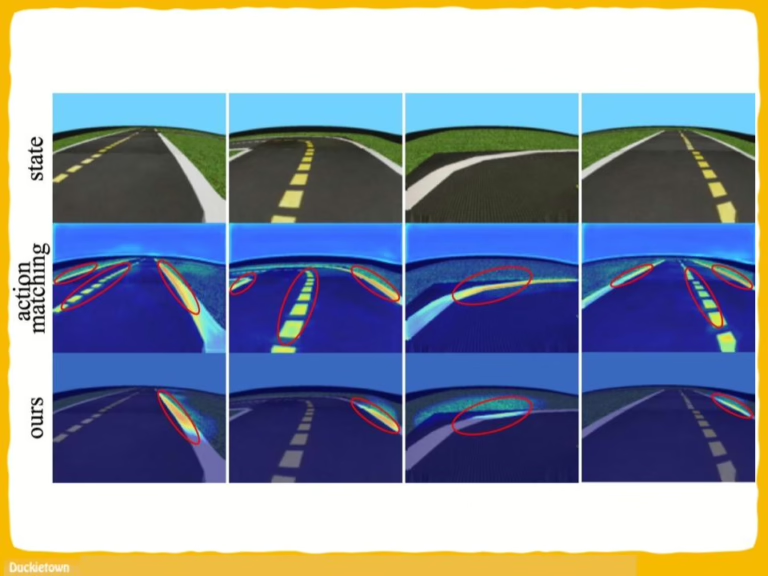
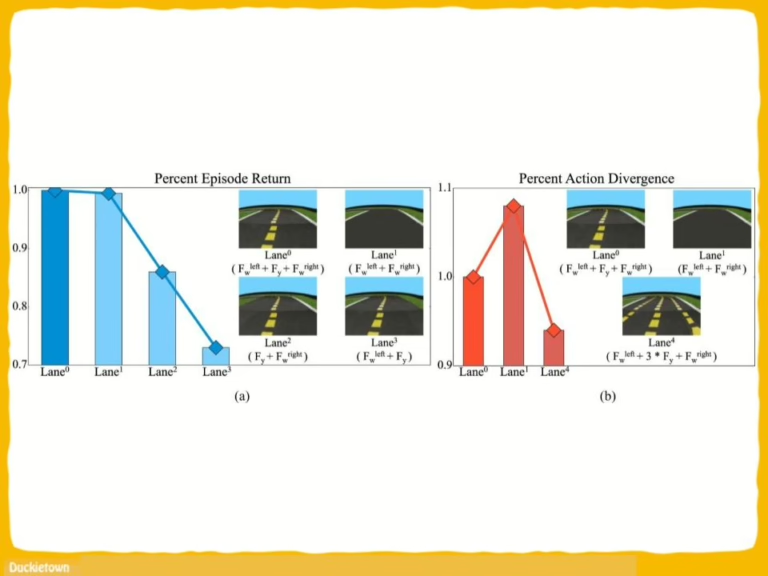
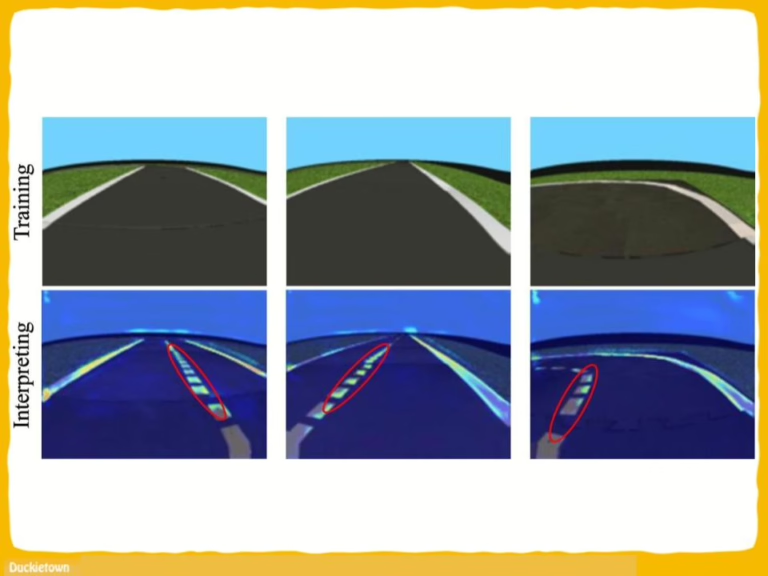
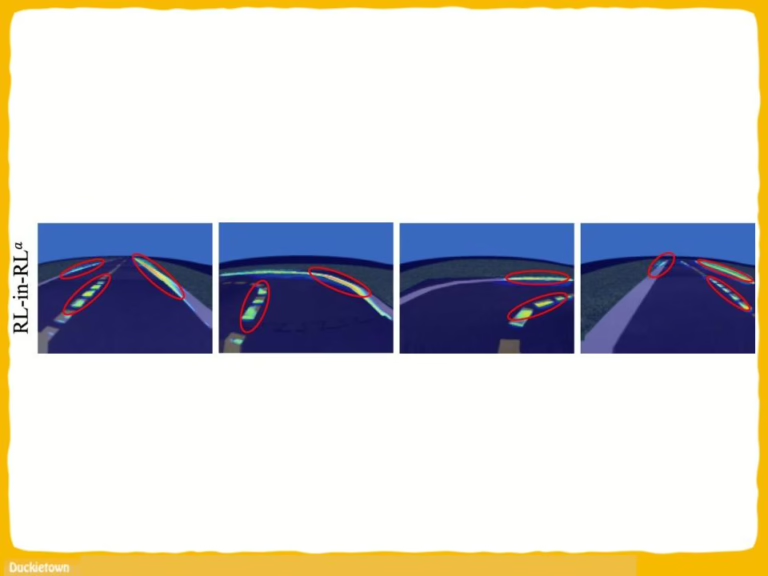
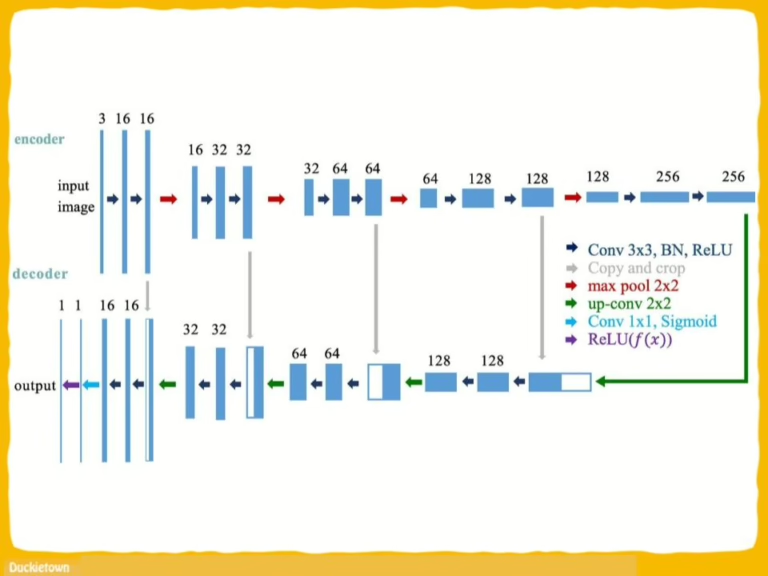
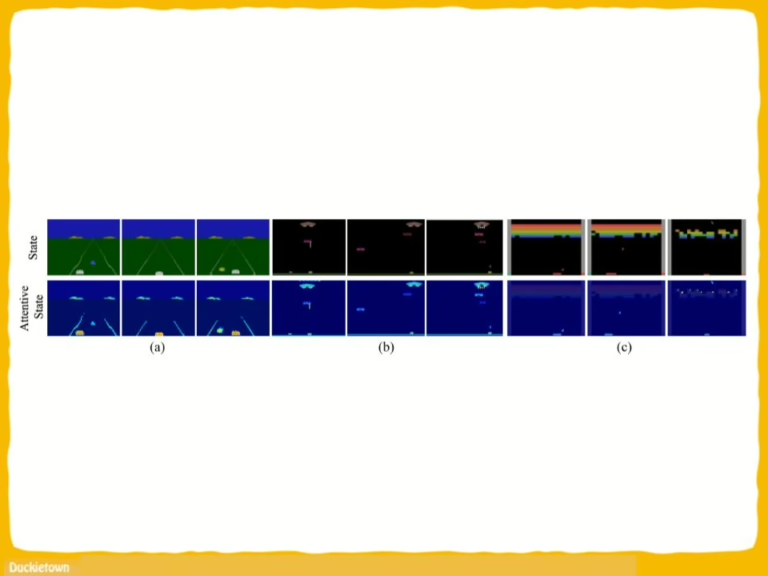
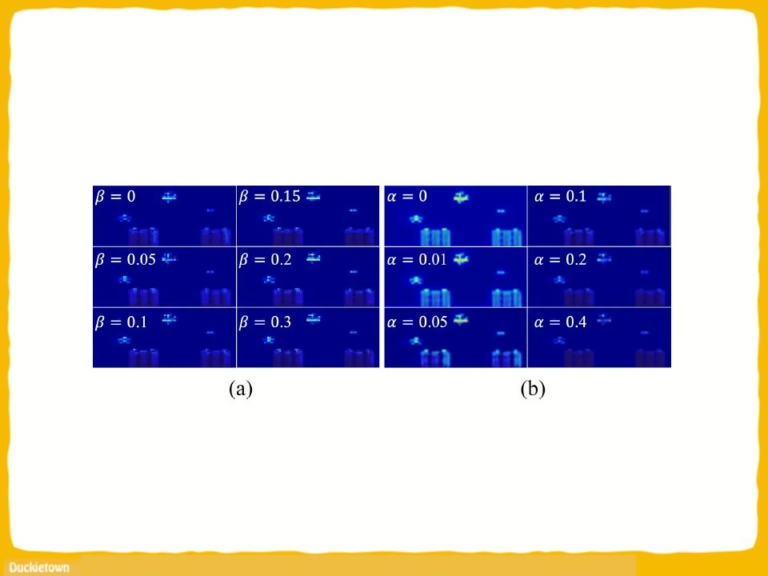
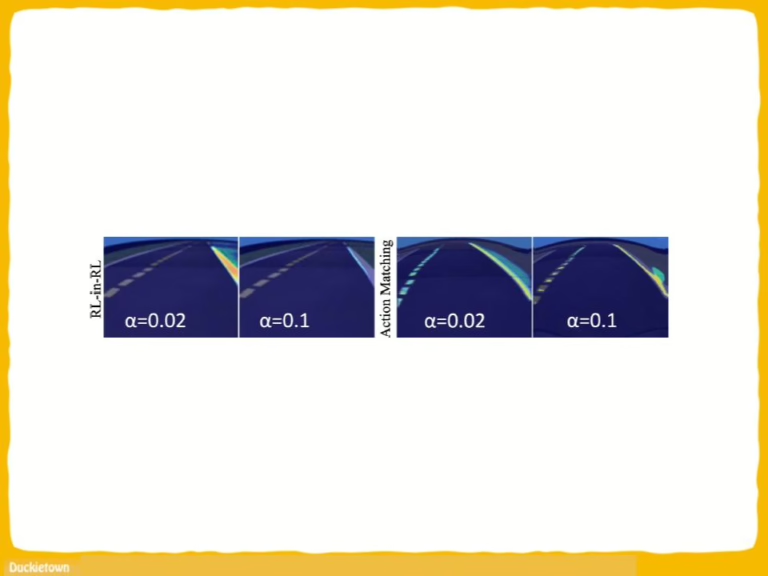
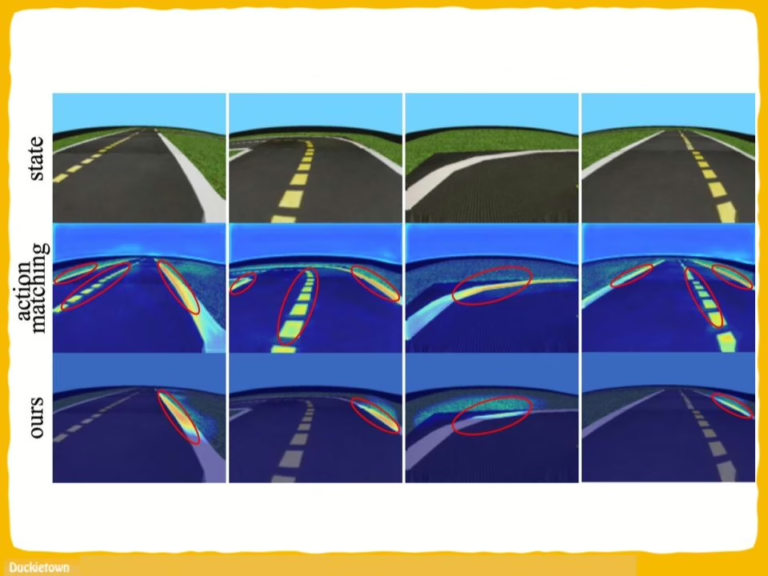
Conclusion
Here are the conclusions from the authors of this paper:
“In this article, we discussed the limitations of the commonly used assumption, the action matching principle, in RL interpretation methods. It is suggested that action matching cannot truly interpret the agent since it differs from the reward-oriented goal of RL. Hence, the proposed method first leverages reward consistency during feature attribution and models the interpretation problem as a new RL problem, denoted as RL-in-RL.
Moreover, it provides an adjustable observation length for one-step reward or multistep reward (or return) consistency, depending on the requirements of behavior analyses. Extensive experiments validate the proposed model and support our concerns that action matching would lead to redundant and noncausal attention during interpretation since it is dedicated to exactly identical actions and thus results in a sort of “overfitting.”
Nevertheless, although RL-in-RL shows superior interpretability and dispenses with redundant attention, further exploration of interpreting RL tasks with explicit causality is left for future work.”
Project Authors
Qisen Yang is an Artificial Intelligence PhD Student at Tsinghua University, China.
Huanqian Wang is currently pursuing the B.E. degree in control science and engineering with the Department of Automation, Tsinghua University, Beijing, China.
Mukun Tong is currently pursuing the B.E. degree in control science and engineering with the Department of Automation, Tsinghua University,
Beijing, China.
Wenjie Shi received his Ph.D. degree in control science and engineering from the Department of Automation, Institute of Industrial Intelligence and System, Tsinghua University, Beijing, China, in 2022.
Guang-Bin Huang is in the School of Electrical and Electronic Engineering, Nanyang Technological University, Singapore.
Shiji Song is currently a Professor with the Department of Automation, Tsinghua University, Beijing, China.
Learn more
Duckietown is a platform for creating and disseminating robotics and AI learning experiences.
It is modular, customizable and state-of-the-art, and designed to teach, learn, and do research. From exploring the fundamentals of computer science and automation to pushing the boundaries of knowledge, Duckietown evolves with the skills of the user.
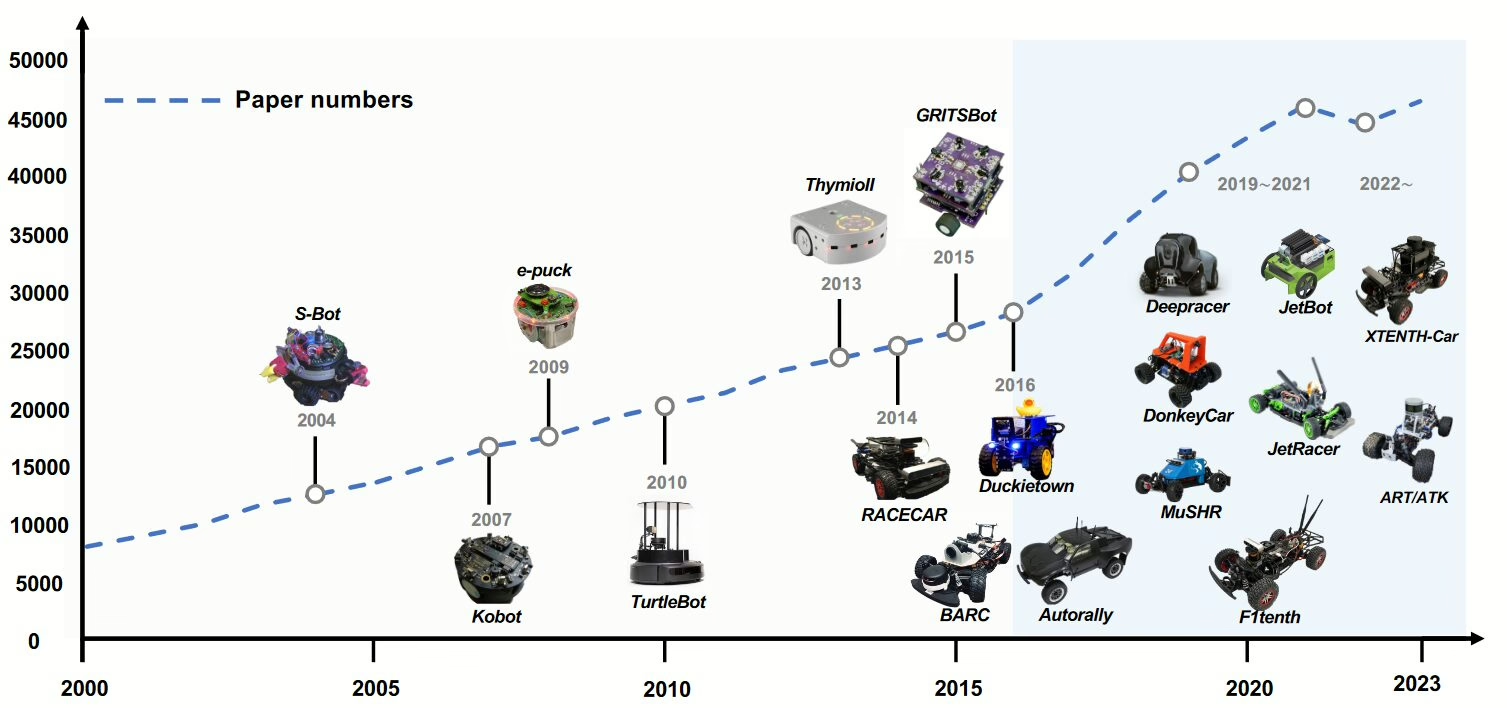
Towards Autonomous Driving with Small-Scale Cars: A Survey of Recent Development
General Information
- Towards Autonomous Driving with Small-Scale Cars: A Survey of Recent Development
- Dianzhao Li, Paul Auerbach, Ostap Okhrin
- Technische Universität Dresden, Germany
Towards Autonomous Driving with Small-Scale Cars: A Survey of Recent Development
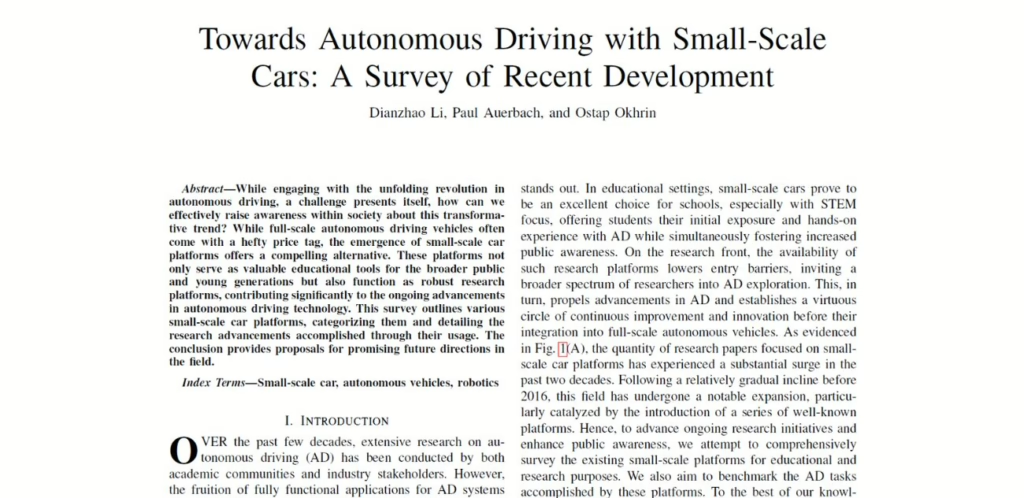
“Towards Autonomous Driving with Small-Scale Cars: A Survey of Recent Development by Dianzhao Li, Paul Auerbach, and Ostap Okhrin is a review that highlights the rapid development of the industry and the important contributions of small-scale car platforms to robot autonomy research.
This survey is a valuable resource for anyone looking to get their bearings in the landscape of autonomous driving research.
We are glad see Duckietown – not only included on the list – but identified as one of the platforms that started a marked increase in the trend of yearly published papers.
The mission of Duckietown, since we started at as a class at MIT, is to democratize access to the science and technology of robot autonomy. Part of how we intended to achieve this mission was to streamline the way autonomous behaviors for non-trivial robots were developed, tested and deployed in the real world.
From 2018-2021 we ran several editions of the AI Driving Olympics (AI-DO): an international competition to benchmark the state of the art of embodied AI for safety-critical applications. It was a great experience – not only because it led to the development of the Challenges infrastructure, the Autolab infrastructure, and many agent baselines that catalyze further developments that are now available to the broader community, but even because it was the first time physical robots were brought the world’s leading scientific conference in Machine Learning (NeurIPS: the Neural Information Processing Systems conference – known as NIPS the first time AI-DO was launched).
All this infrastructure development and testing might have been instrumental in making R&D in autonomous mobile robotics more efficient. Practitioners in the field know-how doing R&D is particularly difficult because final outcomes are the result of the tuple (robot) x (environment) x (task) – so not standardizing everything other than the specific feature under development (i.e., not following the ceteris paribus principle) often leads to apples and pair comparisons, i.e., bad science, which hampers the overall progress of the field.
We are happy to see Duckietown recognized as a contributor to facilitating the making of good science in the field. We beleive that even better and more science will come in the next years, as the students being educated with the Duckietown system start their professional journeys in academia or the workforce.
We are excited to see what the future of robot autonomy will look like, and we will continue doing our best by providing tools, workflows, and comprehensive resources to facilitate the professional development of the next generations of scientists, engineers, and practicioners in the field!
To learn more about Duckietown teaching resources follow the link below.
Starting around 2016, with the introduction of Duckietown, BARC, and Autorally, there was a significant increase in research papers.
Dianzhao Li, Paul Auerbach, Ostap Okhrin
Abstract
We report the abstract of the authors’ work:
“While engaging with the unfolding revolution in autonomous driving, a challenge presents itself, how can we effectively raise awareness within society about this transformative trend? While full-scale autonomous driving vehicles often come with a hefty price tag, the emergence of small-scale car platforms offers a compelling alternative.
These platforms not only serve as valuable educational tools for the broader public and young generations but also function as robust research platforms, contributing significantly to the ongoing advancements in autonomous driving technology.
This survey outlines various small-scale car platforms, categorizing them and detailing the research advancements accomplished through their usage. The conclusion provides proposals for promising future directions in the field.”
Towards Autonomous Driving with Small-Scale Cars: A Survey of Recent Development
Here is a visual tour of the work. For more details, check out the full paper.
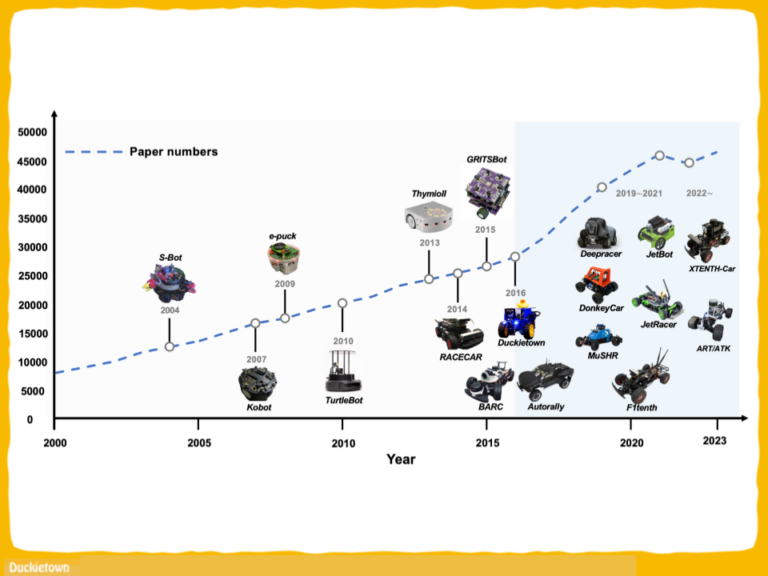
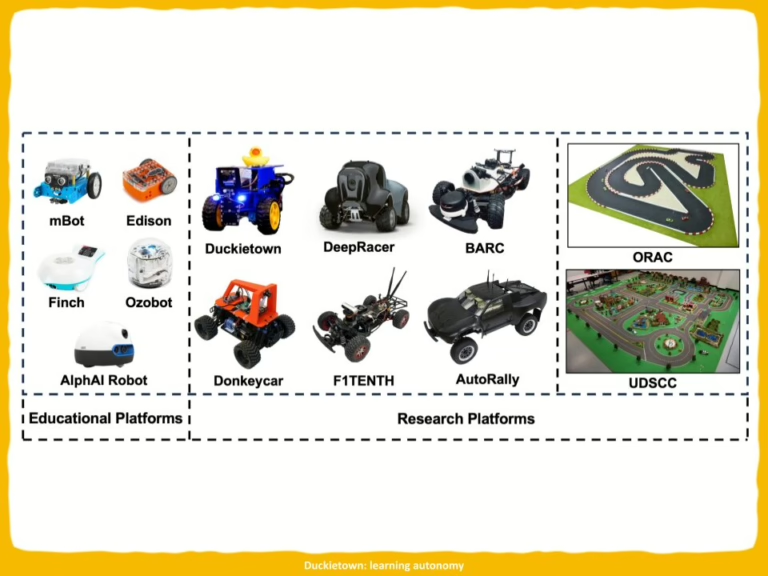
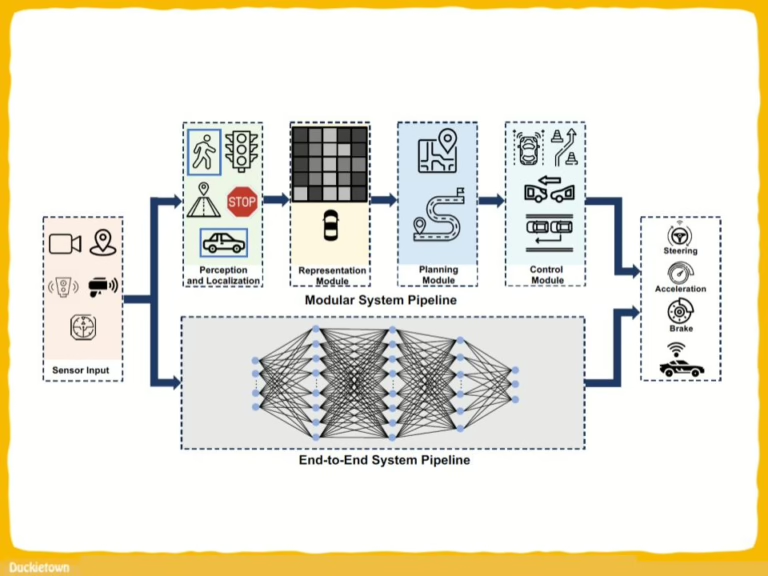
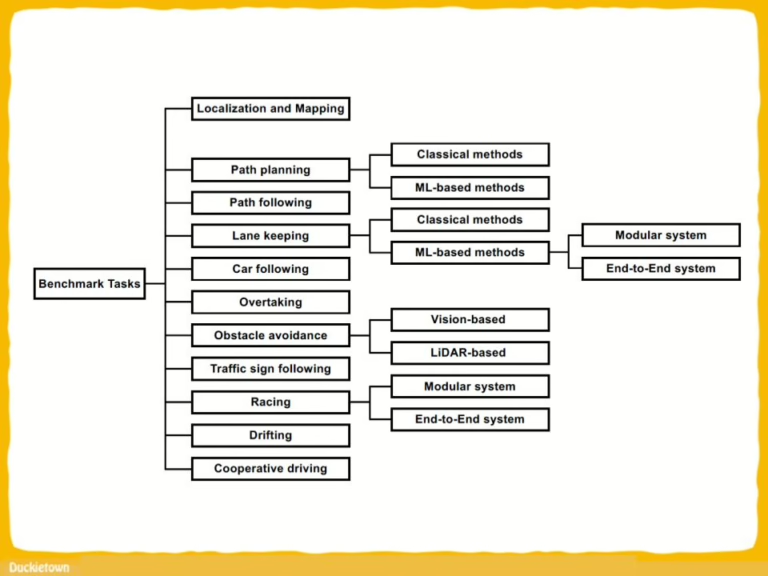
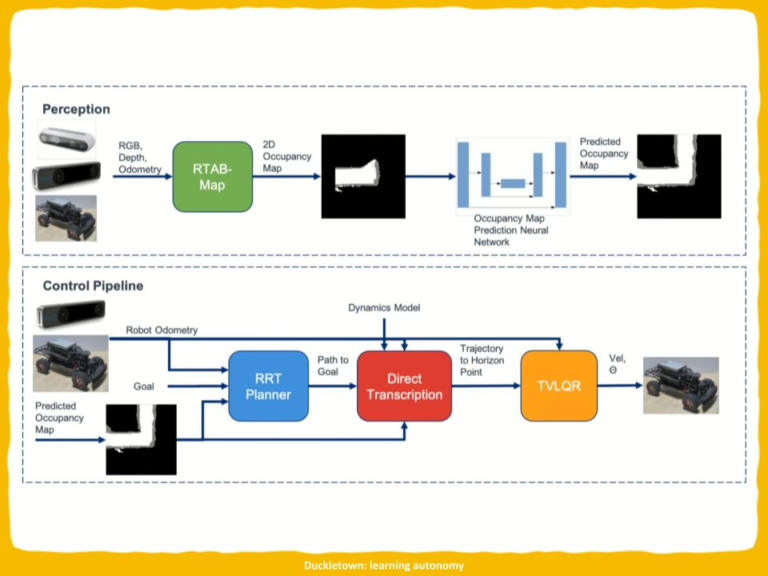
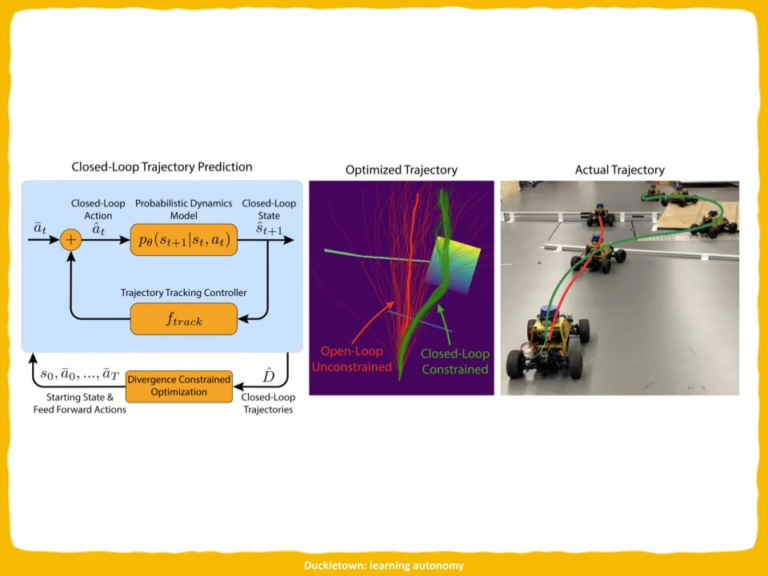
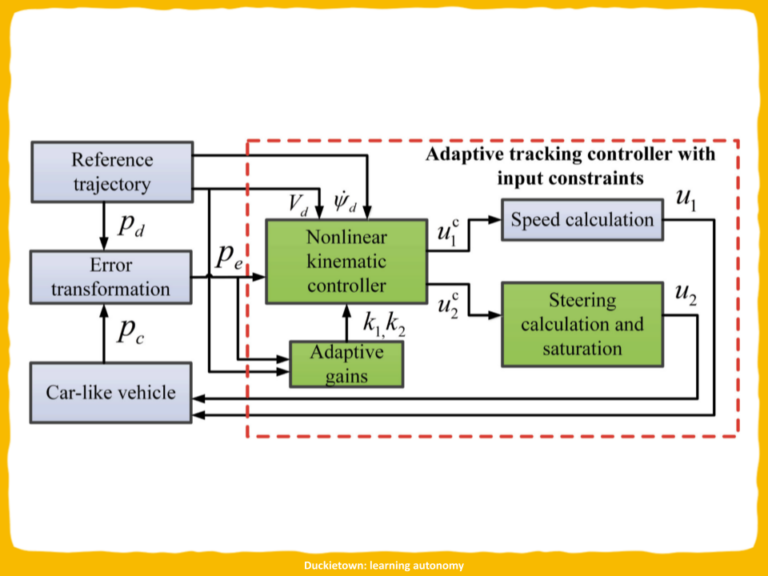
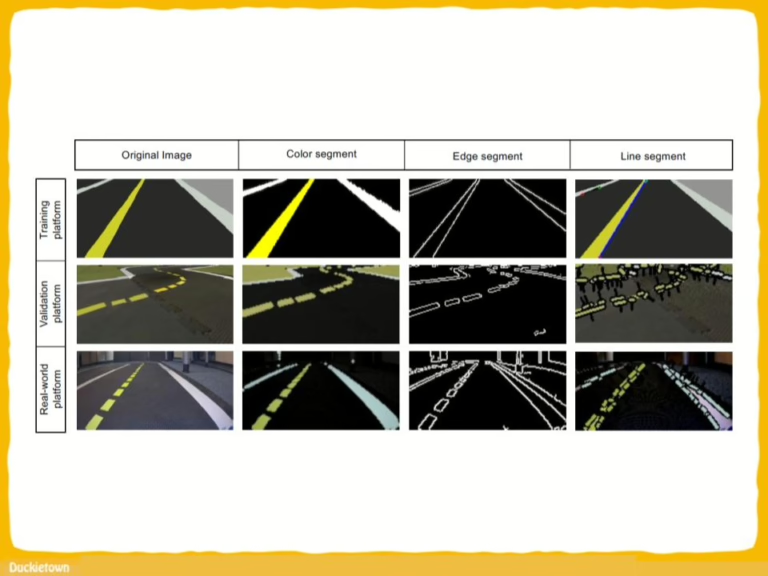
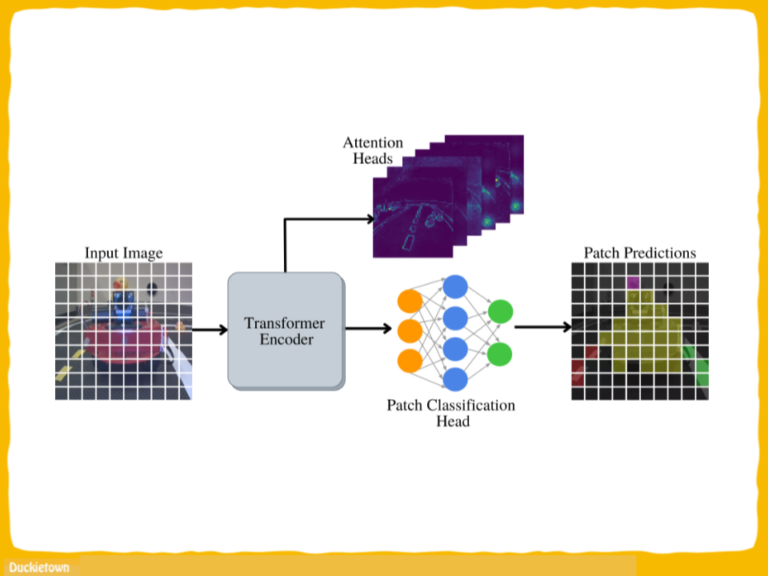
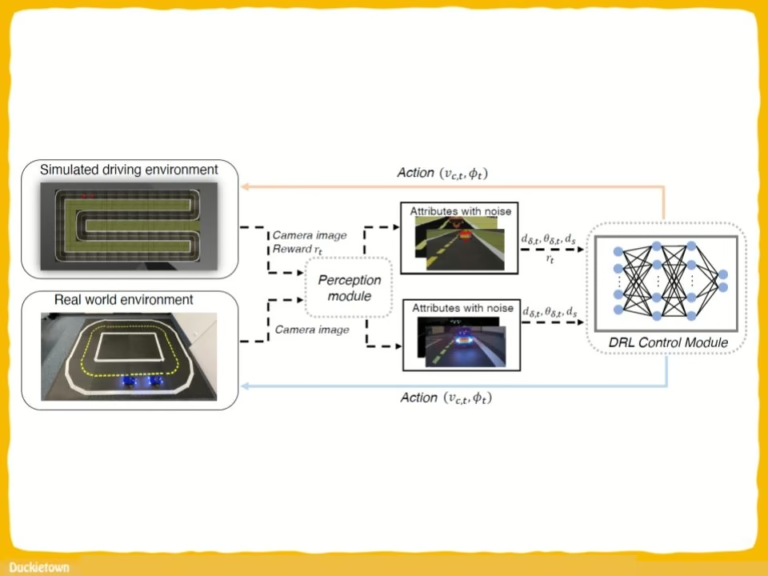
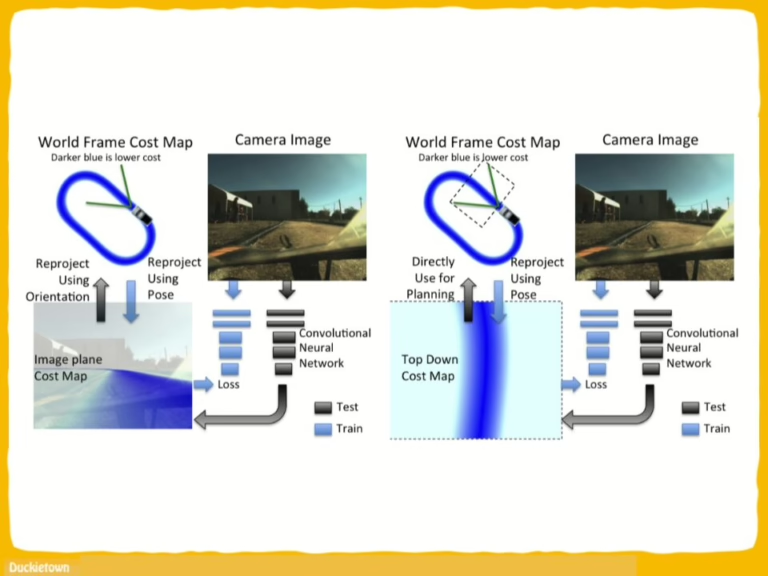
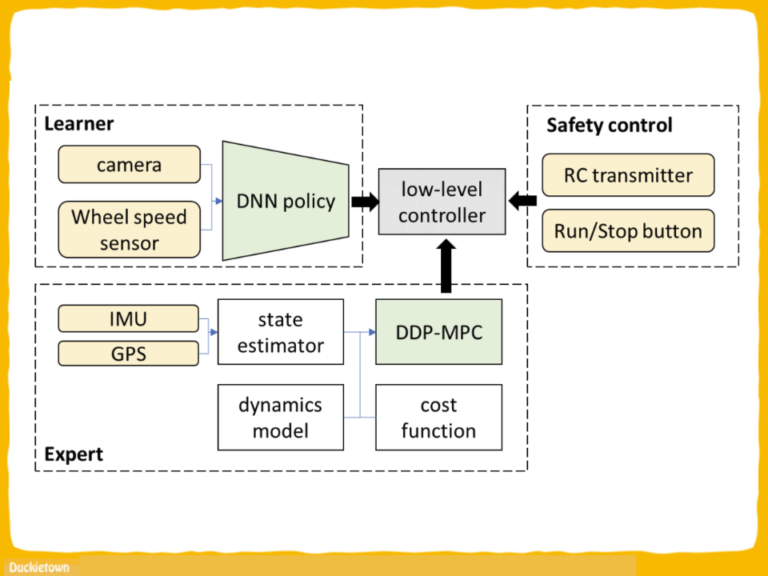
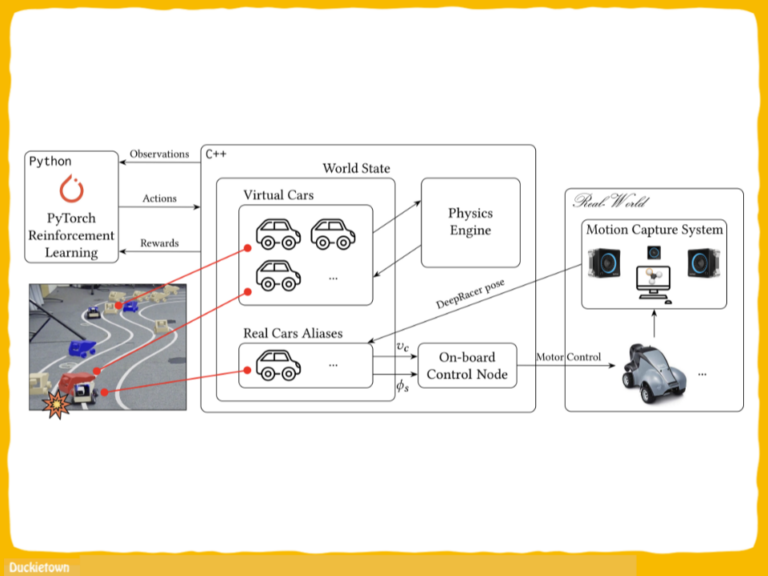
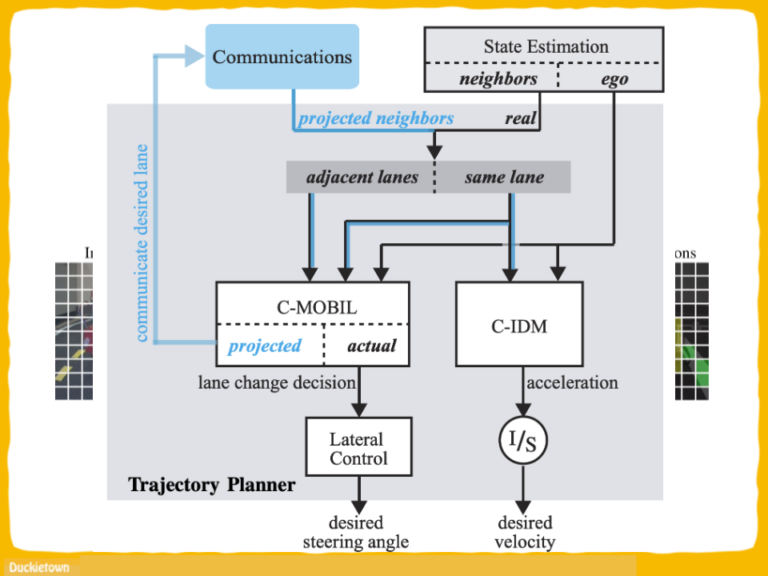
Summary and conclusion
Here is what the authors learned from this survey:
“In this paper, we offer an overview of the current state-of-the- art developments in small-scale autonomous cars. Through a detailed exploration of both past and ongoing research in this domain, we illuminate the promising trajectory for the advancement of autonomous driving technology with small-scale cars. We initially enumerate the presently predominant small-scale car platforms widely employed in academic and educational domains and present the configuration specifics of each platform. Similar to their full-size counterparts, the deployment of hyper-realistic simulation environments is imperative for training, validating, and testing autonomous systems before real-world implementation. To this end, we show the commonly employed universal simulators and platform-specific simulators.
Furthermore, we provide a detailed summary and categorization of tasks accomplished by small-scale cars, encompassing localization and mapping, path planning and following, lane-keeping, car following, overtaking, racing, obstacle avoidance, and more. Within each benchmarked task, we classify the literature into distinct categories: end-toend systems versus modular systems and traditional methods 20 versus ML-based methods. This classification facilitates a nuanced understanding of the diverse approaches adopted in the field. The collective achievements of small-scale cars are thus showcased through this systematic categorization. Since this paper aims to provide a holistic review and guide, we also outline the commonly utilized in various well-known platforms. This information serves as a valuable resource, enabling readers to leverage our survey as a guide for constructing their own platforms or making informed decisions when considering commercial options within the community.
We additionally present future trends concerning small-scale car platforms, focusing on different primary aspects. Firstly, enhancing accessibility across a broad spectrum of enthusiasts: from elementary students and colleagues to researchers, demands the implementation of a comprehensive learning pipeline with diverse entry levels for the platform. Next, to complete the whole ecosystem of the platform, a powerful car body, varying weather conditions, and communications issues should be addressed in a smart city setup. These trends are anticipated to shape the trajectory of the field, contributing significantly to advancements in real-world autonomous driving research.
While we have aimed to achieve maximum comprehensiveness, the expansive nature of this topic makes it challenging to encompass all noteworthy works. Nonetheless, by illustrating the current state of small-scale cars, we hope to offer a distinctive perspective to the community, which would generate more discussions and ideas leading to a brighter future of autonomous driving with small-scale cars.”
Project Authors
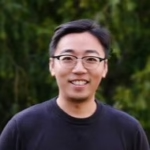
Dianzhao Li is a research assistant at the Technische Universität Dresden, Dresden, Germany.
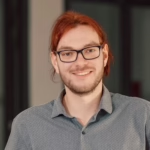
Paul Auerbach is with Barkhausen Institut gGmbH, Dresden, Germany
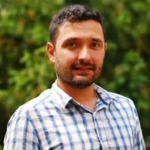
Ostap Okhrin is Chair of Statistics and Econometrics at the Institute of Economics and Transport, School of Transportation, Technische Universitat Dresden in Germany.
Learn more
Duckietown is a platform for creating and disseminating robotics and AI learning experiences.
It is modular, customizable and state-of-the-art, and designed to teach, learn, and do research. From exploring the fundamentals of computer science and automation to pushing the boundaries of knowledge, Duckietown evolves with the skills of the user.
End-to-end Deep RL (DRL) systems: in autonomous driving environments that rely on visual input for vehicle control face potential security risks, including:
- State Adversarial Perturbations: Subtle alterations to visual input that mislead the DRL agent, causing incorrect decision-making.
- Reward Tampering: Manipulation of the reward signal to misguide the learning process, leading the agent to adopt unsafe or inefficient policies.
These vulnerabilities can compromise the safety and reliability of self-driving vehicles.
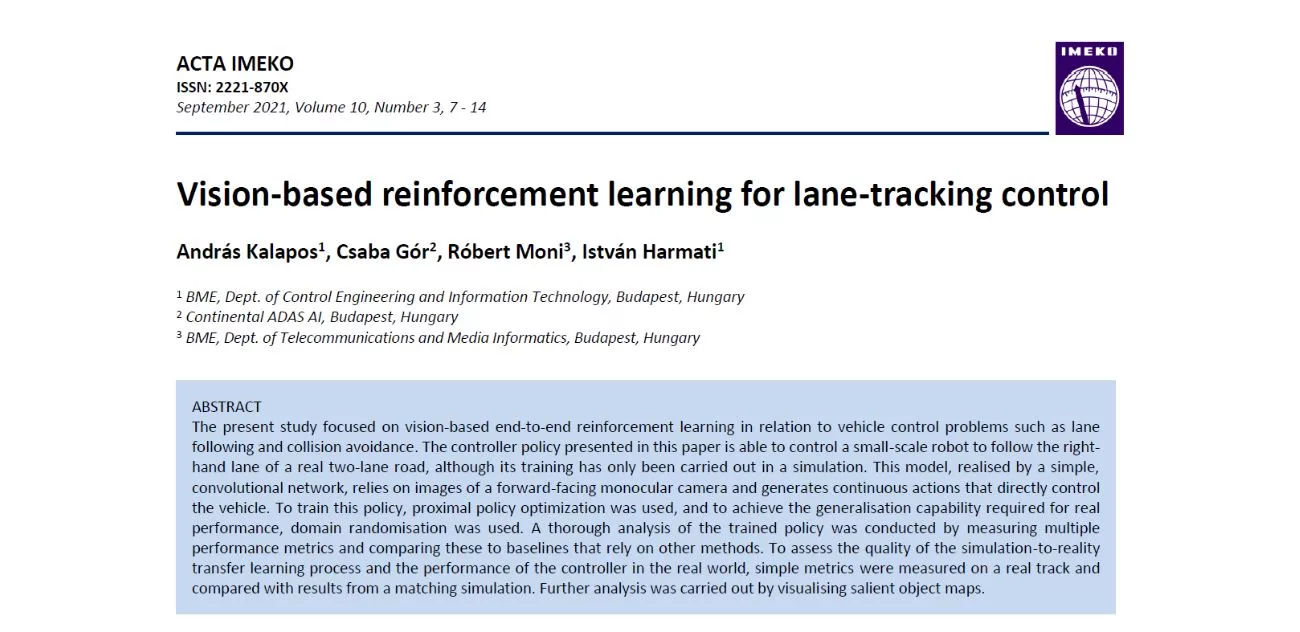
Vision-based Reinforcement Learning for Lane-Tracking Control
General Information
- Vision-based Reinforcement Learning for Lane-Tracking Control
- András Kalapos, Csaba Gór, Róbert Moni, István Harmati
- Budapest University of Technology and Economics
- Kalapos, A., Gór, C., Moni, R. and Harmati, I., 2021. Vision-based reinforcement learning for lane-tracking control. Acta IMEKO, 10(3), pp.7-14.
Vision-based reinforcement learning for lane-tracking control
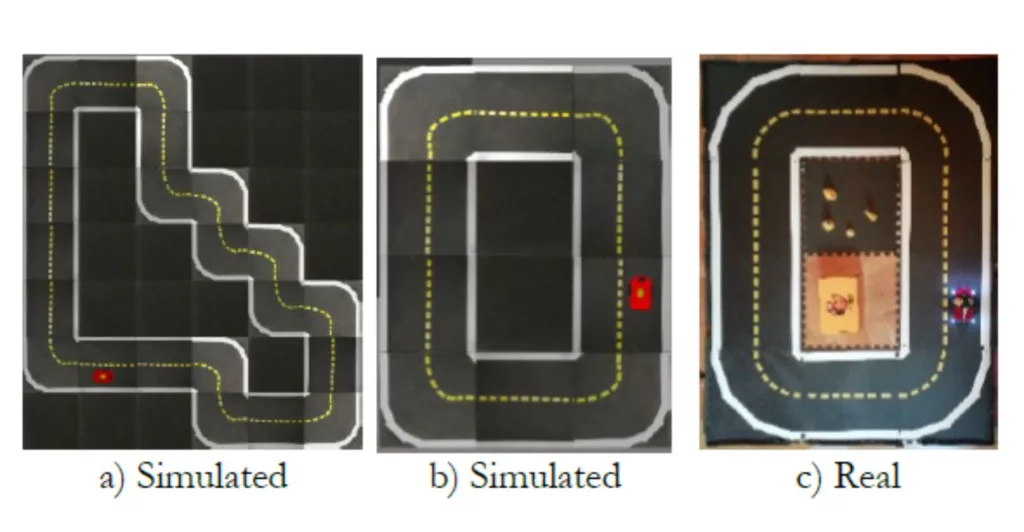
What is Vision-based Reinforcement Learning? A few important topics:
Reinforcement Learning: a machine learning paradigm where an agent learns to make decisions by interacting with an environment to achieve a goal. In this context, reinforcement learning is used to teach a vehicle how to drive within Duckietown lanes by providing rewards or penalties based on its actions.
Vision-based Control: The control of the vehicle is based on visual inputs, specifically images captured by a forward-facing camera. These images are processed by a neural network to determine appropriate steering actions, allowing the vehicle to track lanes and avoid collisions.
Simulation-to-Reality (sim2real) Transfer Learning: The trained policy, which learns to control the vehicle in a simulated environment, is transferred to real-world scenarios. The effectiveness of the trained model in real-world driving situations is evaluated, demonstrating the ability to generalize learning from simulation to reality.
Domain Randomization: This technique involves introducing variations or randomizations into the simulation environment during training. By exposing the agent to a wide range of simulated scenarios with different lighting conditions, road surfaces, and other environmental factors, domain randomization helps improve the model’s ability to generalize to unseen real-world conditions.
Learn about RL, navigation and other robot autonomy topics at the link below!
Abstract
The present study focused on vision-based end-to-end reinforcement learning in relation to vehicle control problems such as lane following and collision avoidance. The controller policy presented in this paper is able to control a small-scale robot to follow the right-hand lane of a real two-lane road, although its training has only been carried out in a simulation.
This model, realised by a simple, convolutional network, relies on images of a forward-facing monocular camera and generates continuous actions that directly control the vehicle. To train this policy, proximal policy optimization was used, and to achieve the generalisation capability required for real performance, domain randomisation was used. A thorough analysis of the trained policy was conducted by measuring multiple performance metrics and comparing these to baselines that rely on other methods.
To assess the quality of the simulation-to-reality transfer learning process and the performance of the controller in the real world, simple metrics were measured on a real track and compared with results from a matching simulation. Further analysis was carried out by visualising salient object maps.
Highlights - Vision-based reinforcement learning for lane-tracking control
Here is a visual tour of the work of the authors. For more details, check out the full paper.
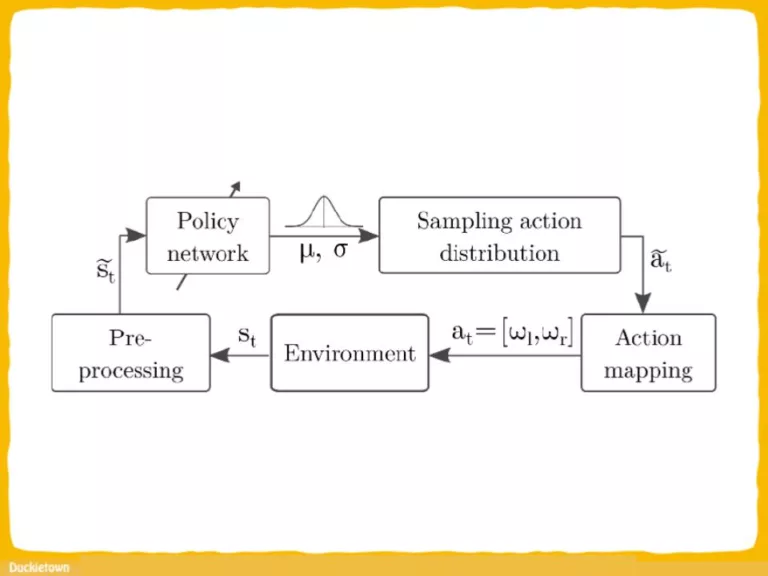
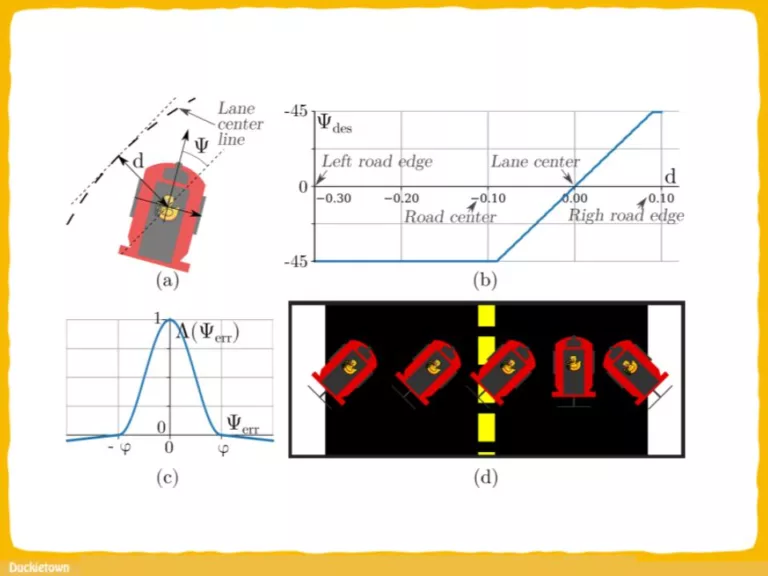
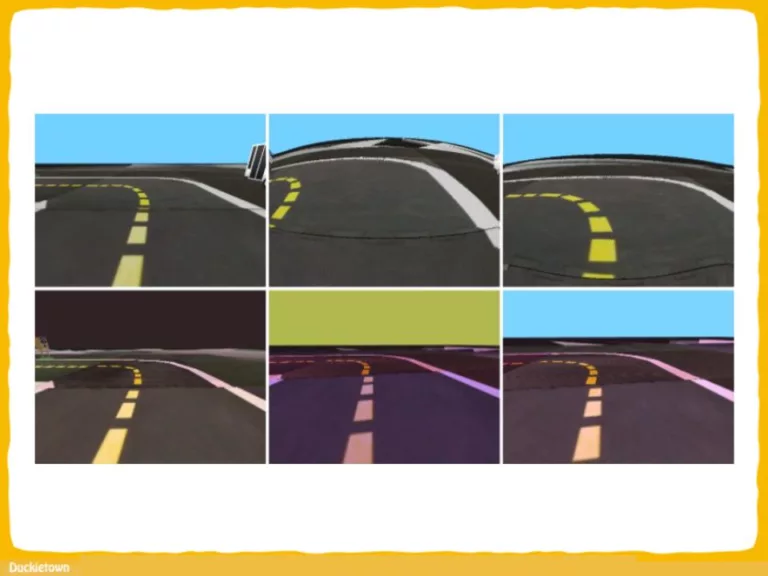
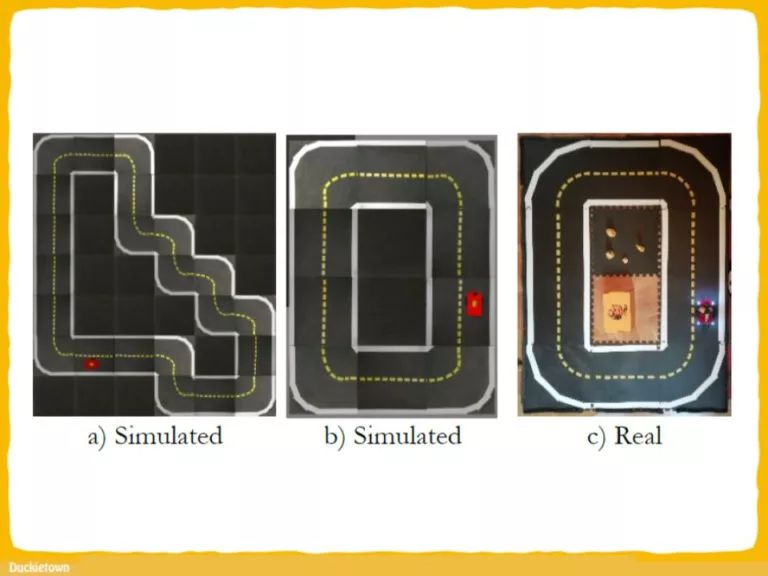
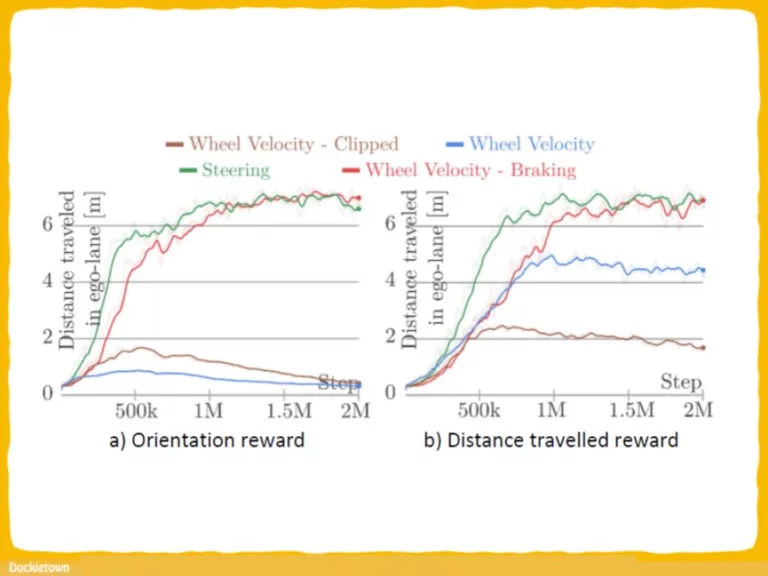
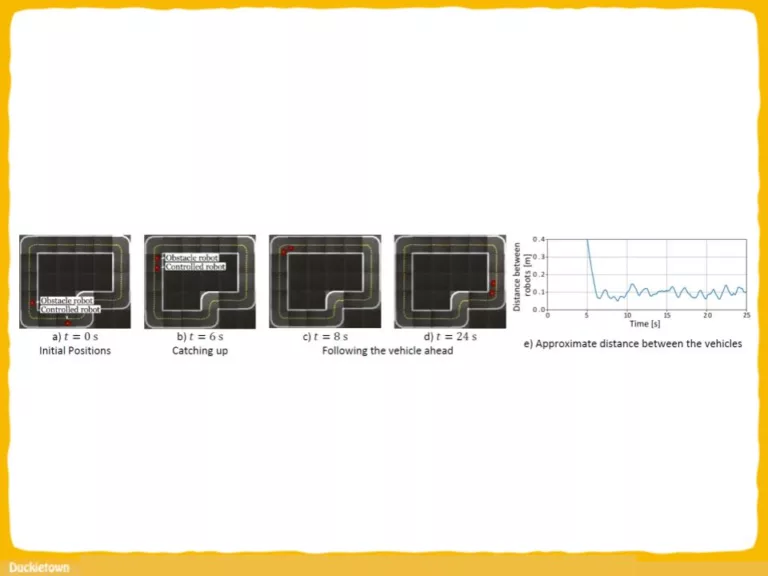
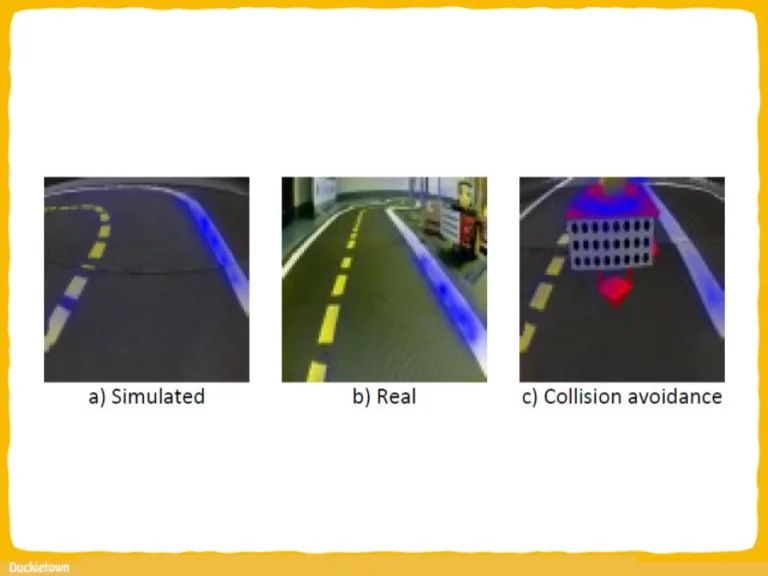
Conclusion
Here are the conclusions from the authors of this paper:
“This work presented a solution to the problem of complex, vision-based lane following in the Duckietown environment using reinforcement learning to train an end-to-end steering policy capable of simulation-to-real transfer learning. It was found that the training is sensitive to problem formulation, such as the representation of actions.
This study has demonstrated that by using domain randomisation, a moderately detailed and accurate simulation is sufficient for training end-to-end lane-following agents that operate in a real environment. The performance of these agents was evaluated by comparing some basic metrics to match real and simulated scenarios.
Agents were also successfully trained to perform collision avoidance in addition to lane following. Finally, salient object visualisation was used to give an illustrative explanation of the inner workings of the policies in both the real and simulated domains.”.
Project Authors
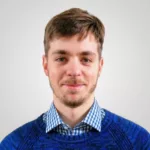
András Kalapos is a Machine Learning PhD Student at Budapest University of Technology and Economics, Hungary.
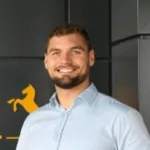
Róbert Moni is a Senior Machine Learning Engineer at Continental.
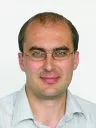
István Harmati is an Associate Professor at Budapest University of Technology and Economics.
Learn more
Duckietown is a platform for creating and disseminating robotics and AI learning experiences.
It is modular, customizable and state-of-the-art, and designed to teach, learn, and do research. From exploring the fundamentals of computer science and automation to pushing the boundaries of knowledge, Duckietown evolves with the skills of the user.
End-to-end Deep RL (DRL) systems: in autonomous driving environments that rely on visual input for vehicle control face potential security risks, including:
- State Adversarial Perturbations: Subtle alterations to visual input that mislead the DRL agent, causing incorrect decision-making.
- Reward Tampering: Manipulation of the reward signal to misguide the learning process, leading the agent to adopt unsafe or inefficient policies.
These vulnerabilities can compromise the safety and reliability of self-driving vehicles.
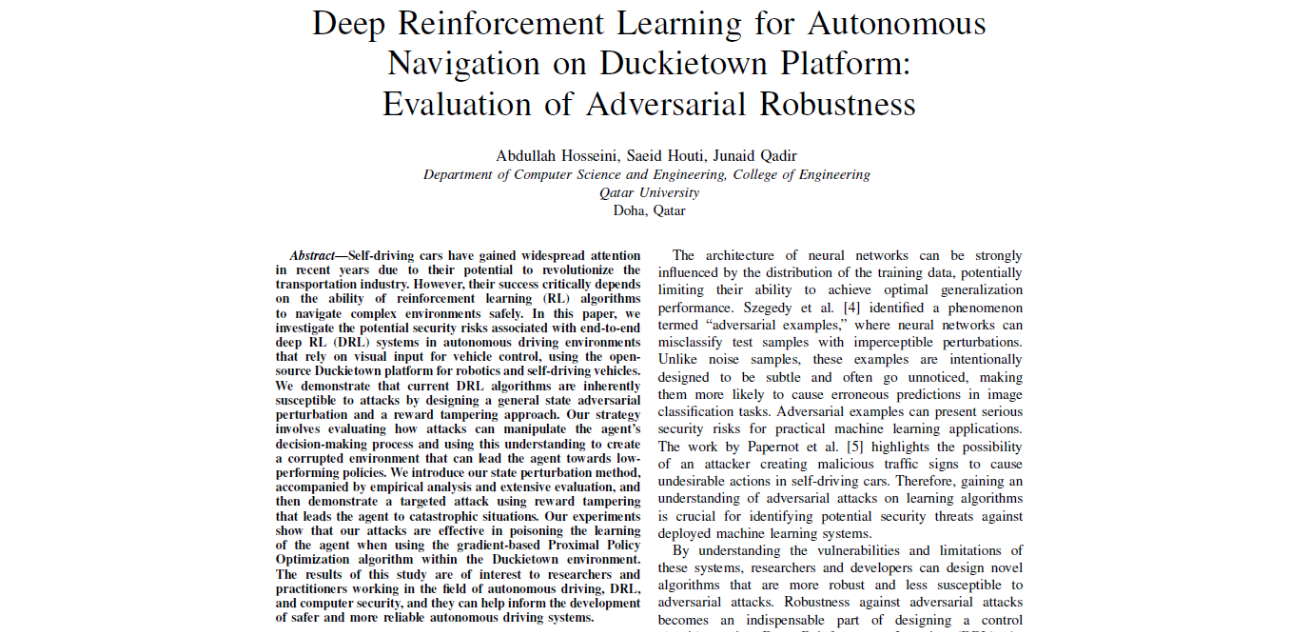
Evaluating Adversarial Robustness in Duckietown Navigation
General Information
- Deep Reinforcement Learning for Autonomous Navigation on Duckietown Platform: Evaluation of Adversarial Robustness
- Abdullah Hosseini, Saeid Houti, Junaid Qadir
- Qatar University
- A. Hosseini, S. Houti and J. Qadir, "Deep Reinforcement Learning for Autonomous Navigation on Duckietown Platform: Evaluation of Adversarial Robustness," 2023 International Symposium on Networks, Computers and Communications (ISNCC), Doha, Qatar, 2023, pp. 1-6, doi: 10.1109/ISNCC58260.2023.10323905.
Deep RL for Autonomous Navigation on Duckietown Platform: Evaluation of Adversarial Robustness
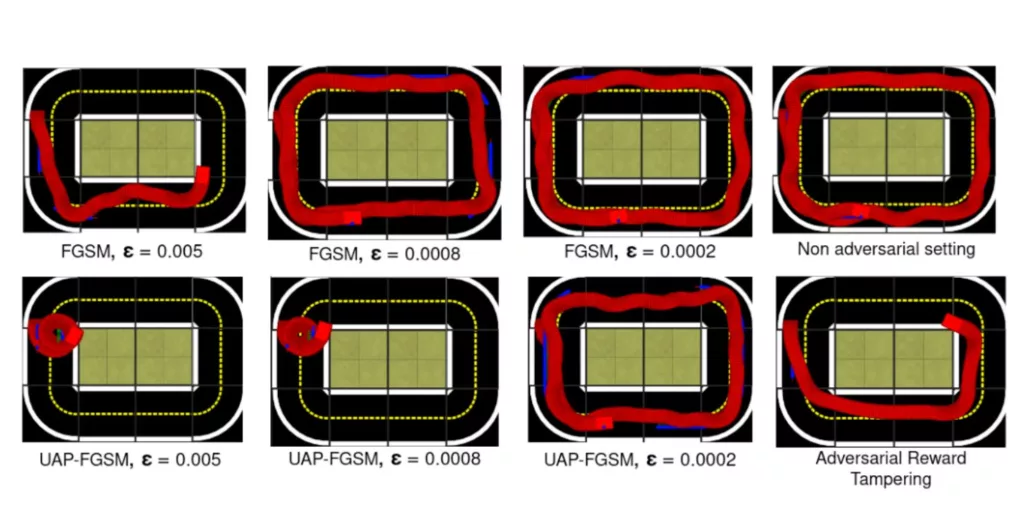
What is adversarial robustness in navigation tasks all about? A few important topics:
Reinforcement Learning (RL) is a type of machine learning where agents learn to make decisions by receiving rewards or penalties based on their actions in an environment. This is great because it removed the need for curated training datasets.
Deep Reinforcement Learning (DRL) enhances RL by using deep neural networks to process complex inputs and make decisions. Deep networks are neural networks with multiple layers.
Adversarial Robustness refers to a system’s ability to resist and maintain performance despite deliberate attacks or input perturbations.
Navigation is the task of finding feasible paths between points in the environment like Google Maps or similar systems provide us in everyday life.
Learn about RL, navigation and other robot autonomy topics at the link below.
Abstract
Self-driving cars have gained widespread attention in recent years due to their potential to revolutionize the transportation industry. However, their success critically depends on the ability of reinforcement learning (RL) algorithms to navigate complex environments safely. In this paper, we investigate the potential security risks associated with end-to-end deep RL (DRL) systems in autonomous driving environments that rely on visual input for vehicle control, using the open-source Duckietown platform for robotics and self-driving vehicles.
We demonstrate that current DRL algorithms are inherently susceptible to attacks by designing a general state adversarial perturbation and a reward tampering approach. Our strategy involves evaluating how attacks can manipulate the agent’s decision-making process and using this understanding to create a corrupted environment that can lead the agent towards low-performing policies. We introduce our state perturbation method, accompanied by empirical analysis and extensive evaluation, and then demonstrate a targeted attack using reward tampering that leads the agent to catastrophic situations.
Our experiments show that our attacks are effective in poisoning the learning of the agent when using the gradient-based Proximal Policy Optimization algorithm within the Duckietown environment. The results of this study are of interest to researchers and practitioners working in the field of autonomous driving, DRL, and computer security, and they can help inform the development of safer and more reliable autonomous driving systems.
Highlights - Evaluation of Adversarial Robustness Results
Here is a visual tour of the work of the authors. For more details, check out the paper link.
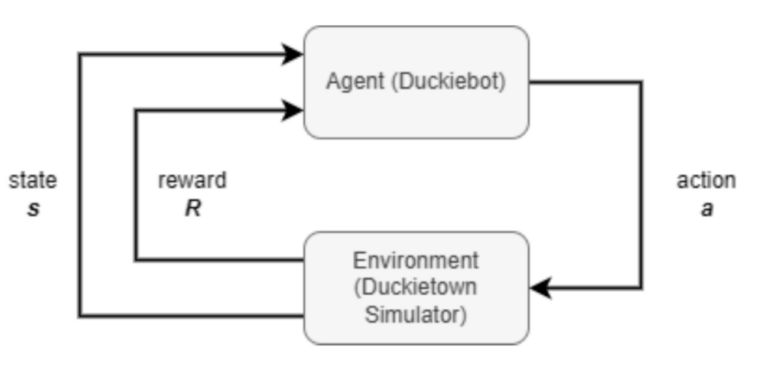
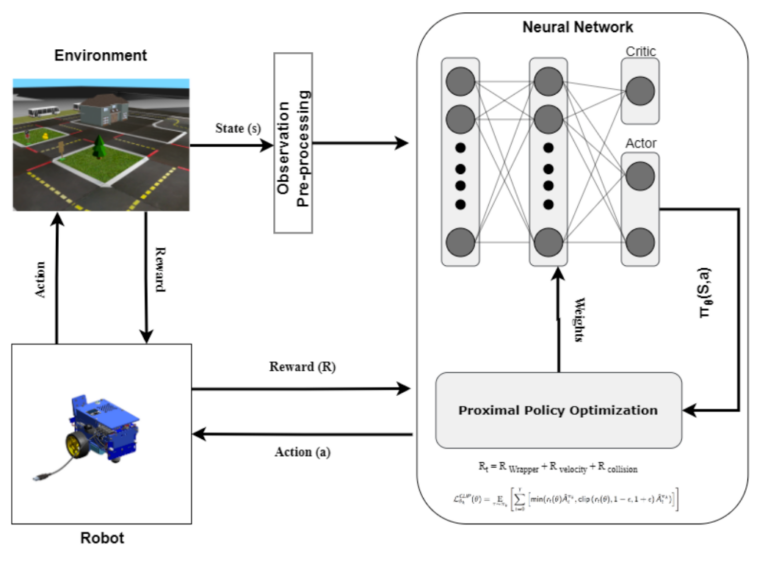
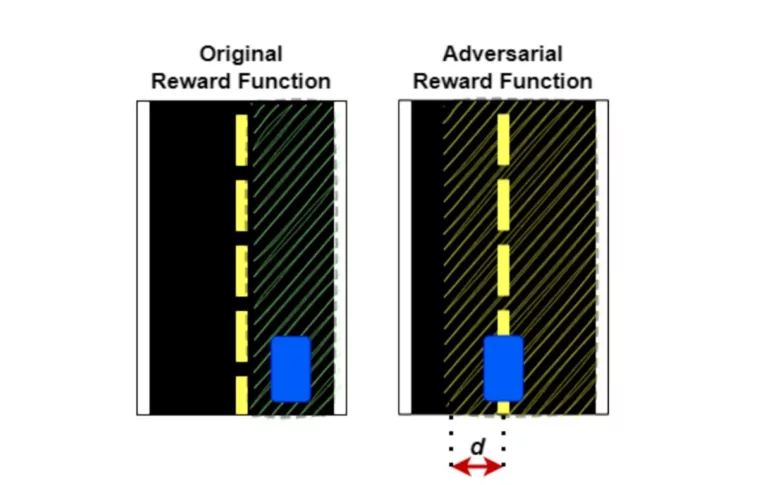
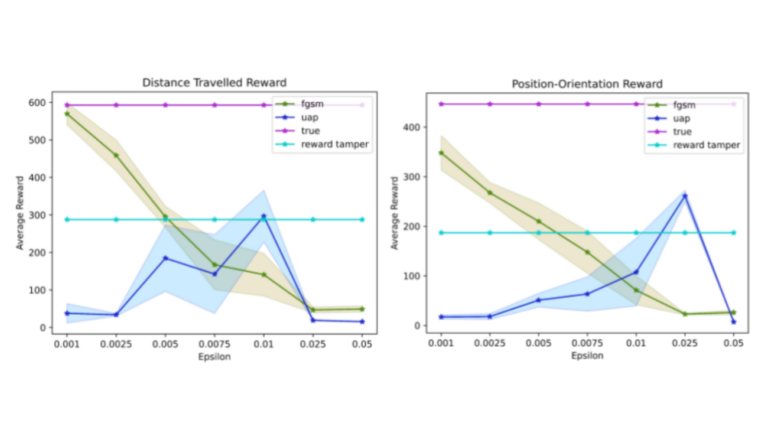
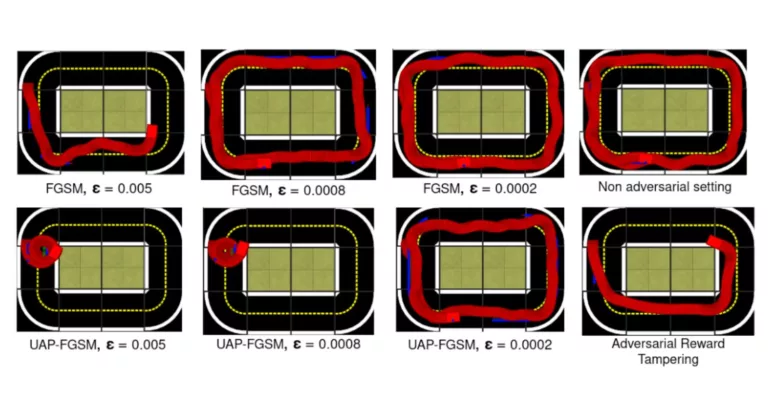
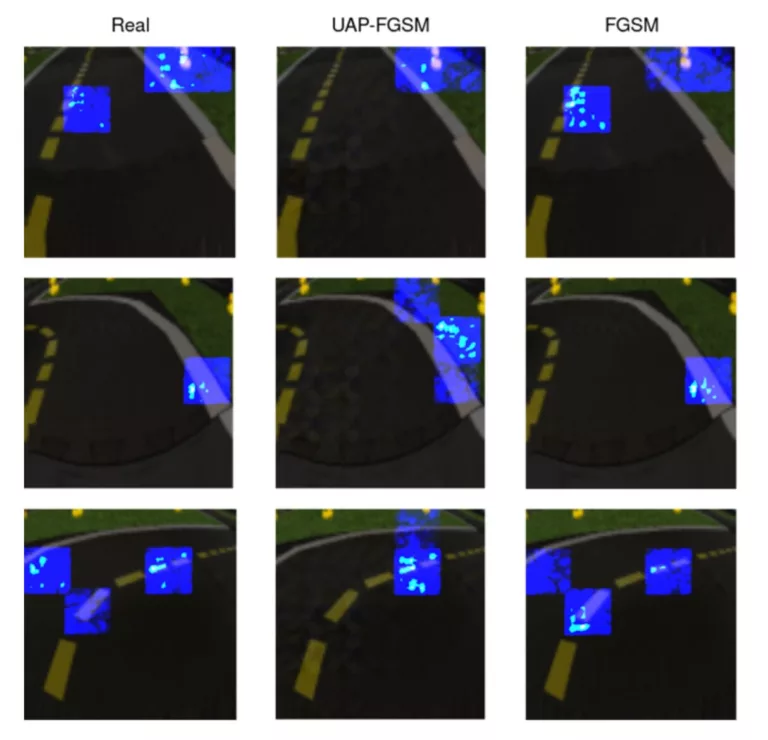
Conclusion
Here are the conclusions from the authors of this paper:
“The focus of our study was to address adversarial attacks on deep reinforcement learning (DRL) agents, specifically examining state adversarial attacks and reward-tampering attacks.
We developed a parametric framework for state adversarial attacks and a non-parametric framework for reward tampering attacks, which enabled us to create effective attacks. We found that the performance of a DRL agent declined rapidly after the attack, and the deviation from the road was worse than that of standard DRL.
We used salient maps to provide a clear explanation of the policies’ internal operations in both the adversarial and non-adversarial aspects. Our research provides insight into the potential vulnerabilities of DRL agents and highlights the need for more robust and secure agents to mitigate the risk of adversarial attacks.
Moving forward, future work will focus on incorporating real-world analysis to test the performance of the DuckieBot under both adversarial and non-adversarial settings”.
Project Authors
Abdullah Hosseini is a Research and Development Specialist at Weill Cornell Medicine in Qatar.
Saeid Houti is a Software Developer at Ministry of Education and Higher Education Qatar.
Junaid Qadir is a Professor of Computer Engineering at Qatar University.
Learn more
Duckietown is a platform for creating and disseminating robotics and AI learning experiences.
It is modular, customizable and state-of-the-art, and designed to teach, learn, and do research. From exploring the fundamentals of computer science and automation to pushing the boundaries of knowledge, Duckietown evolves with the skills of the user.
End-to-end Deep RL (DRL) systems: in autonomous driving environments that rely on visual input for vehicle control face potential security risks, including:
- State Adversarial Perturbations: Subtle alterations to visual input that mislead the DRL agent, causing incorrect decision-making.
- Reward Tampering: Manipulation of the reward signal to misguide the learning process, leading the agent to adopt unsafe or inefficient policies.
These vulnerabilities can compromise the safety and reliability of self-driving vehicles.