In case you missed it AI-DO 3 has come and gone. Interested in reliving the competition? Here’s the video.
We had a great time at NeurIPS hosting the Third Edition of the AI Driving Olympics. As usual the sound of Duckies attracted an engaging and supportive crowd.
Racing Event
The competition began with the Racing Event, hosted by AWS DeepRacer. They ran their top 10 submissions and selected the winner by who could complete the fastest lap.
Racing Event Winner
Ayrat Baykov at 8:08 seconds
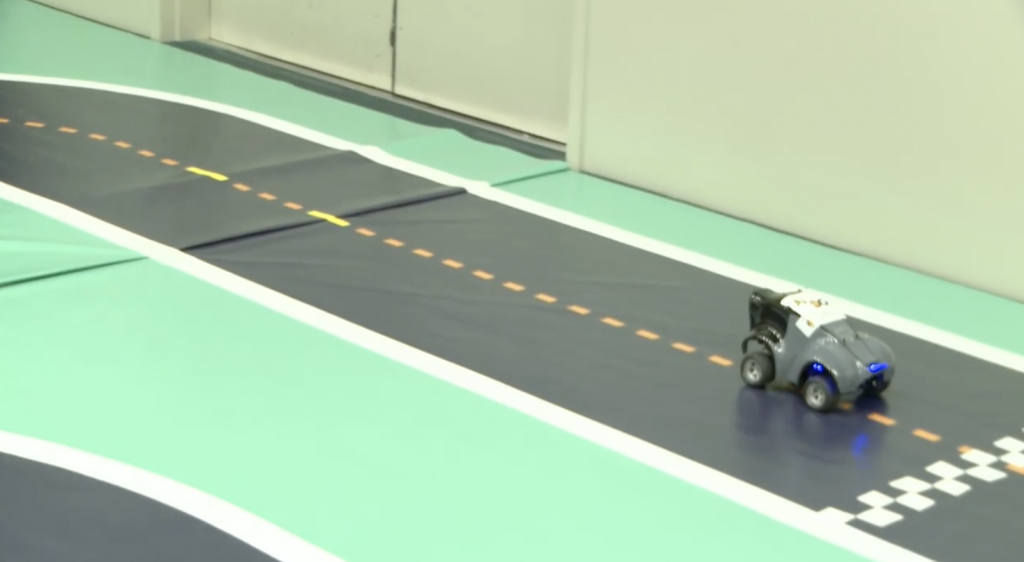
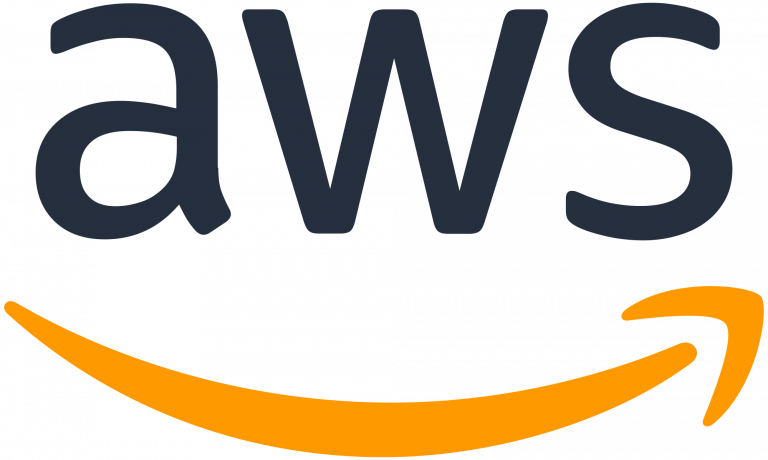
Advanced Perception Event
The winners of the Advanced Perception Event hosted by APTIV and the nuScenes dataset were announced. Luckily a member of the winning team was present to accept the award.
Rank 3
CenterTrack – Open and Vision
Rank 2
VV_Team
Rank 1
StanfordlPRL-TRI
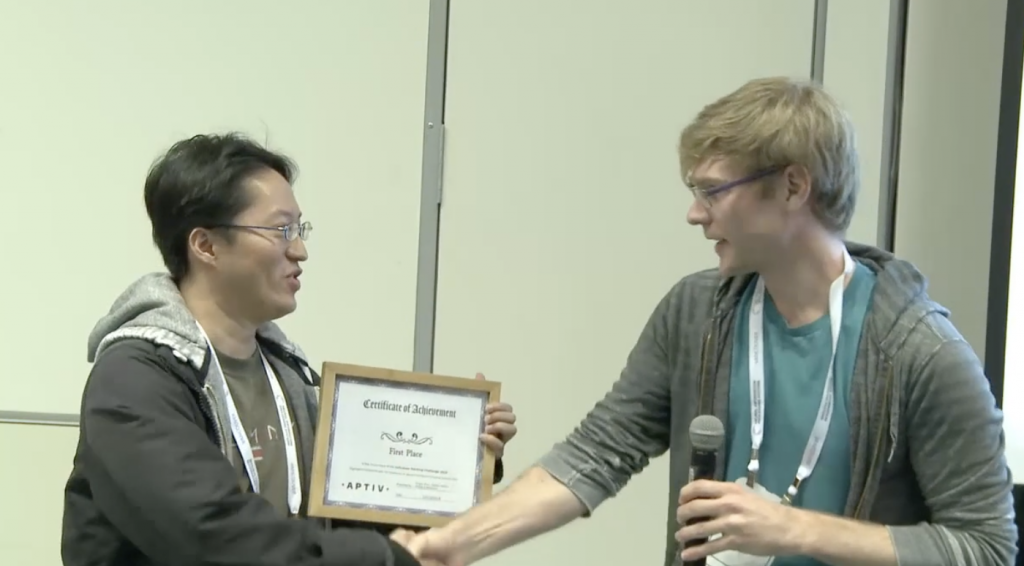
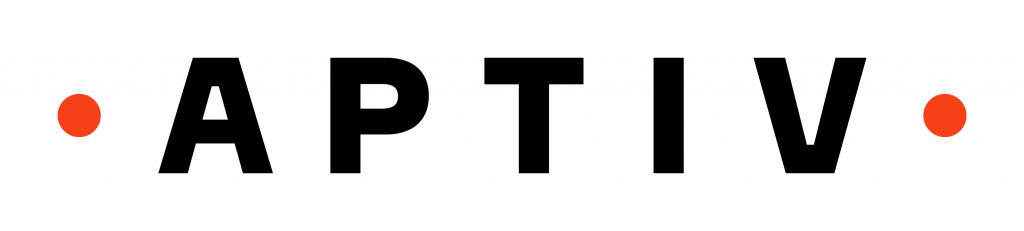
Urban Event
The competition culminated with Duckietown’s own Urban Driving Event, where we ran the top submissions for each of the three challenges on our competition tracks.
Winners
Lane Following
JBRRussia1: Konstantin Chaika, Nikita Sazanovich, Kirill Krinkin, Max Kuzmin
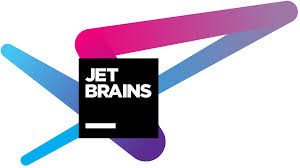
Lane Following with Vehicles
phmarm
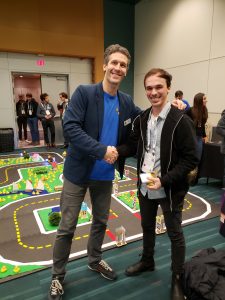
Lane Following with Vehicles and Intersections
frank_qcd_qk
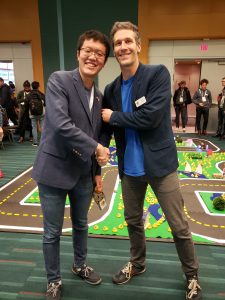
Final Scoreboard
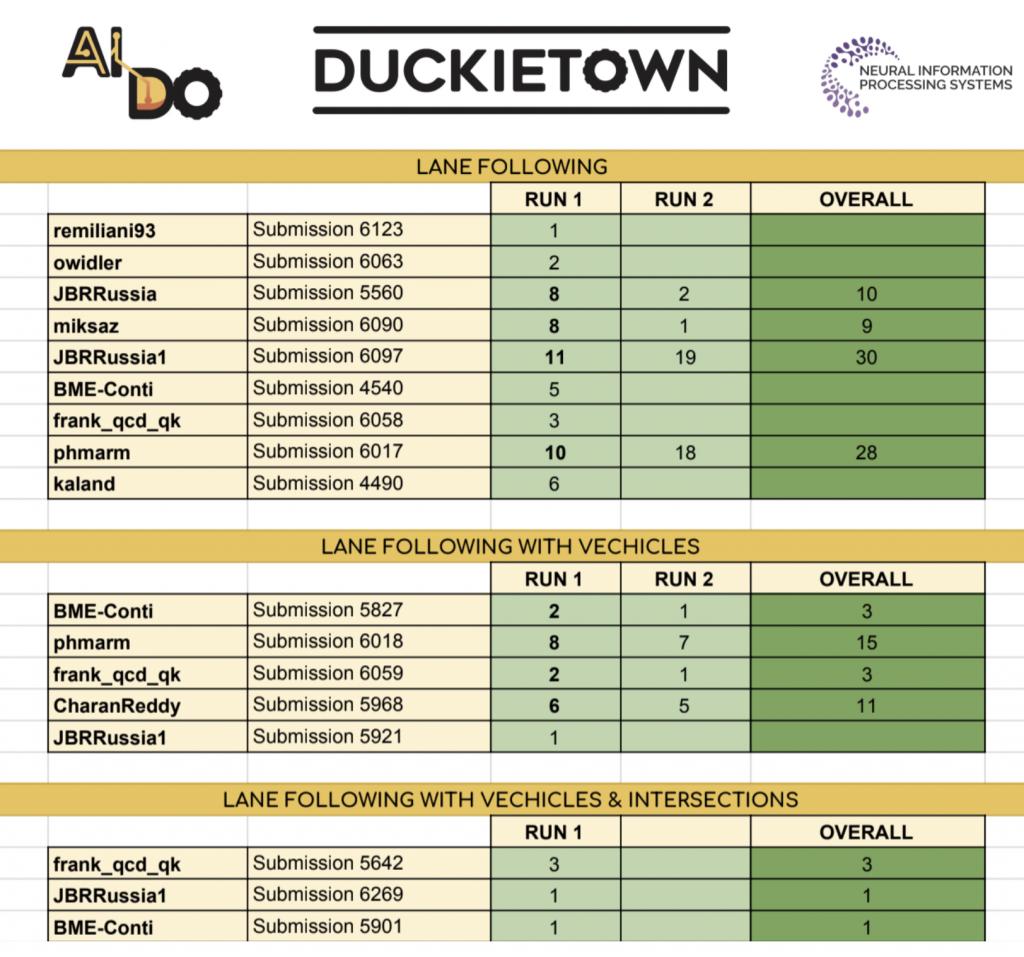
A few pictures from the event
Congratulations to all the winners and thanks for participating in the competition. We look forward to seeing you for AI-DO 4!