UdM, Montréal, May 5, 2022: Liam Paull, professor at the University of Montreal and one of Duckietown’s founders, talks about his role and experiences with Duckietown.
When rubber duckies meet the road: an interview with Prof. Liam Paull
Liam Paull, professor at the University of Montreal in Quebec, and one of the very founders of Duckietown, shares below his unique perspective about Duckietown’s journey and its origin.
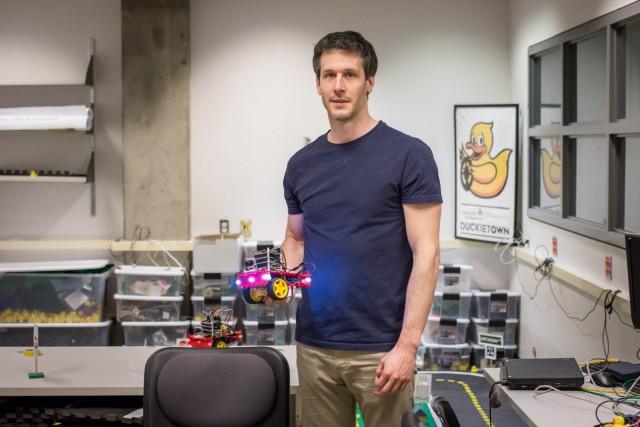
Good morning, Liam.
Hello.
Thank you very much for accepting to have this little chat.
Could you tell us something about you?
Sure. So my name is Liam Paull. I’m a professor at the University of Montreal in Quebec, Canada. I teach in the computer science Department, And I do research on robotics.
Ok and when was the first time you “came across” Duckietown?
Well, I’m actually one of the creators of Duckietown, So I didn’t come across it as much! The origin story of Duckietown is kind of interesting, But I probably forgot some of the details. It must have been about 2015. And myself, Andrea Censi, and a few others were interested to get more teaching experience. We were all postdocs or research scientists at MIT at the time. I guess we started brainstorming ideas, and then roughly around that time, I switched positions at MIT. I was previously a postdoc in John Leonard’s group working on marine robotics, and then I switched to become part of Danielle Lerous lab and lead an autonomous driving project. And so somehow the stars just aligned. That the right topic for this class that we would teach would be autonomous driving. Yeah, the Ducky thing is kind of a separate thing. Actually, Andrea had started this other thing that was making videos for people to publicize their work at a top robotics conference Called the international conference robotics automation, and somehow had the idea that every single video that was submitted should have a rubber Ducky in it. And this was for scale or something.
There was some kind of reason behind it I sort of forget. But anyway, so the branding kind of caught fire.
When we were building the class, we agreed the one constraint was that there should be duckies involved somehow, and the rest is kind of history!
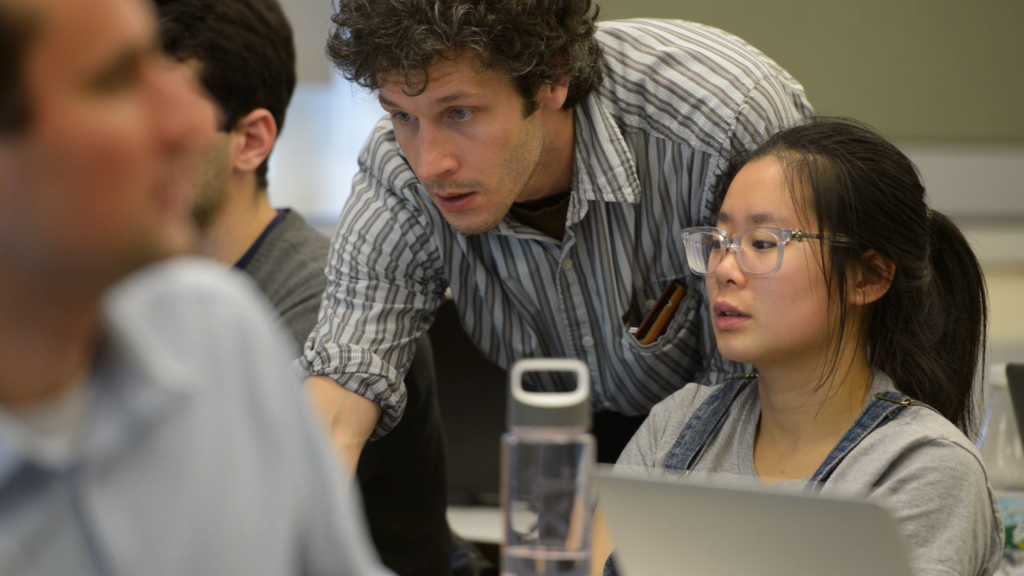
"I believe that there's a lot of interesting research directions that come from a standardized, small scale, accessible autonomous driving platform like Duckietown."
Liam Paull
Anything else you would like to add about Duckietown and it’s uses?
I didn’t mention specifically about the MOOC. One of the core missions of this project from the onset has been that it’s accessible. Both in terms of hardware but also in terms of software. And part of what that means to us Is that no matter where you are, no matter who you are, you should be able to get the hardware and you should be able to use the educational resources to learn. And part of the motivation for that Was that we saw that while we were at MIT. When you’re at a place like MIT you are extremely privileged and if you come from a background of less privilege, you see the discrepancy. In some sense, it’s palpable. Part of that, I guess, was that we don’t even necessarily want it to be a prerequisite that students should be enrolled in universities in order to be able to address the platform. So we built this massive online open source course through edx, which is also an open source provider Where people can, regardless of their background or regardless of their situation, they can sign up for this thing, and it’s a creative set of materials that also have exercises to interact with the robot that anybody can do, Regardless of whether they’re at a University or not.
I think this is the next step for us in making the platform accessible to all, and we’re going to continue to run iterations of this thing. But I also think that this is an exciting objective that very much fits in the mission of what we’re trying to do with this project.
This was great thank you for your time!
Awesome. Great. Thank you for your time. Bye.
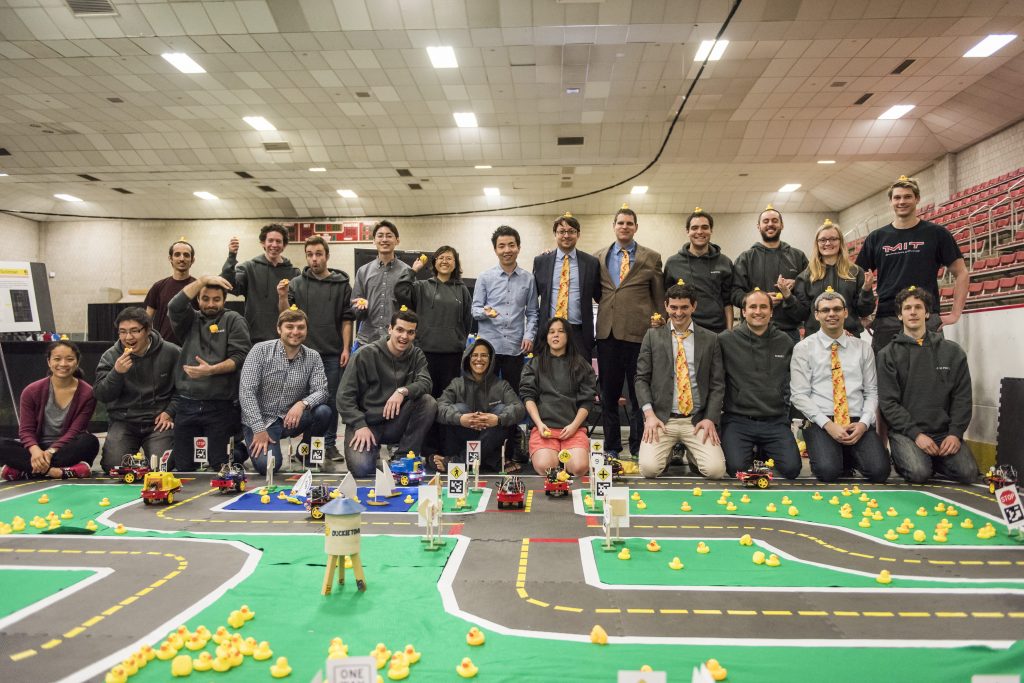
Learn more about Duckietown
The Duckietown platform offers robotics and AI learning experiences.
Duckietown is modular, customizable and state-of-the-art. It is designed to teach, learn, and do research: from exploring the fundamentals of computer science and automation to pushing the boundaries of knowledge.
Tell us your story
Are you an instructor, learner, researcher or professional with a Duckietown story to tell? Reach out to us!