The second edition of the Duckietown Summer School will take place August 6-8 in Taiwan.
Category: Post categories
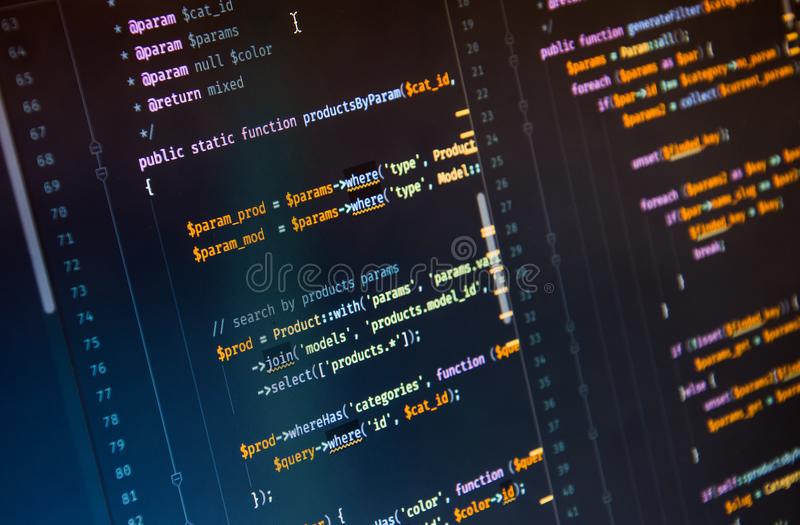
Front-end developer (PHP)
We are looking for a PHP website developer to improve the appearance and functionality of the Duckietown website.
We would like to implement new functionality such as advanced user group management.
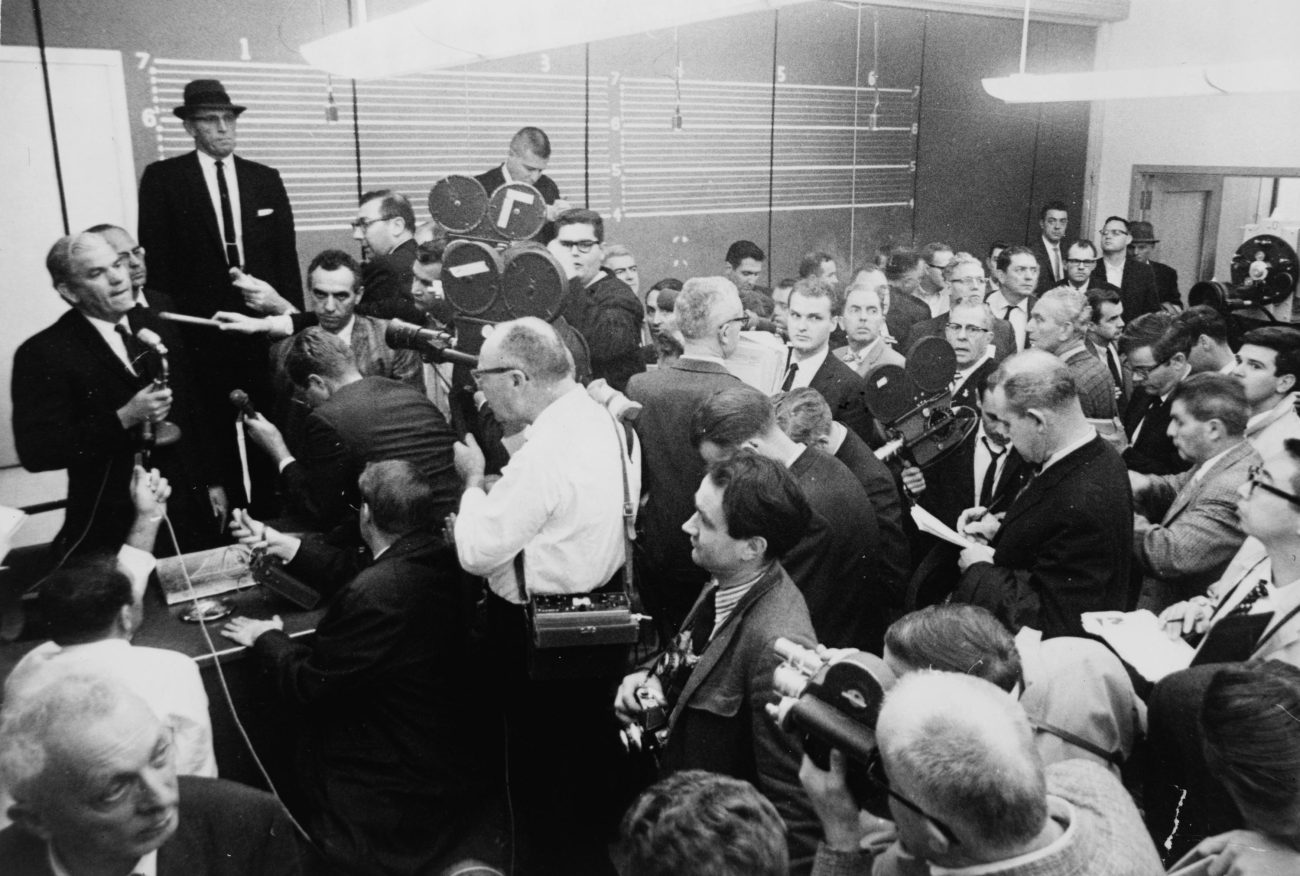
Media Relations Manager
We are looking for a media relations manager to interact with press/media.
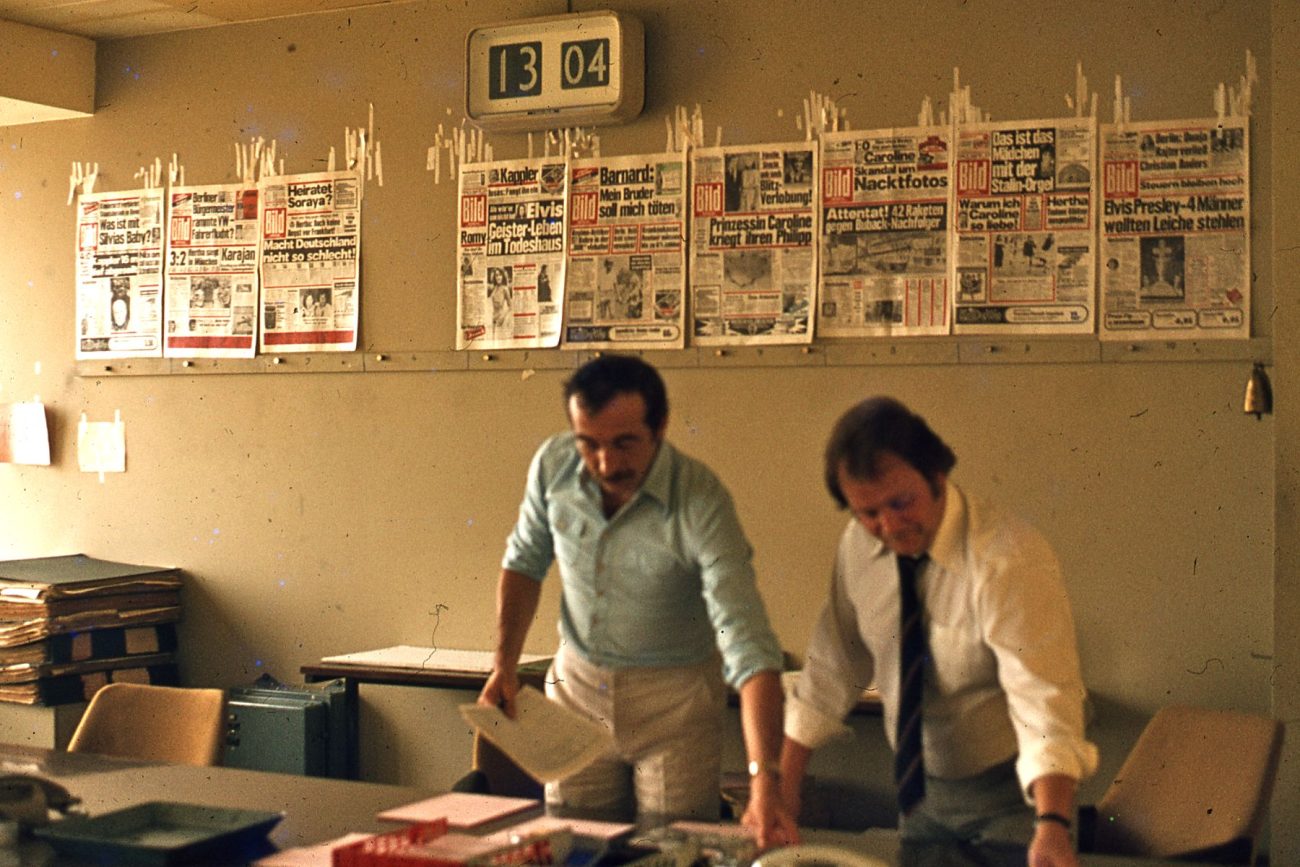
Website editor
We are looking for an editor for our website to coordinate writing and polishing blog posts and web pages.
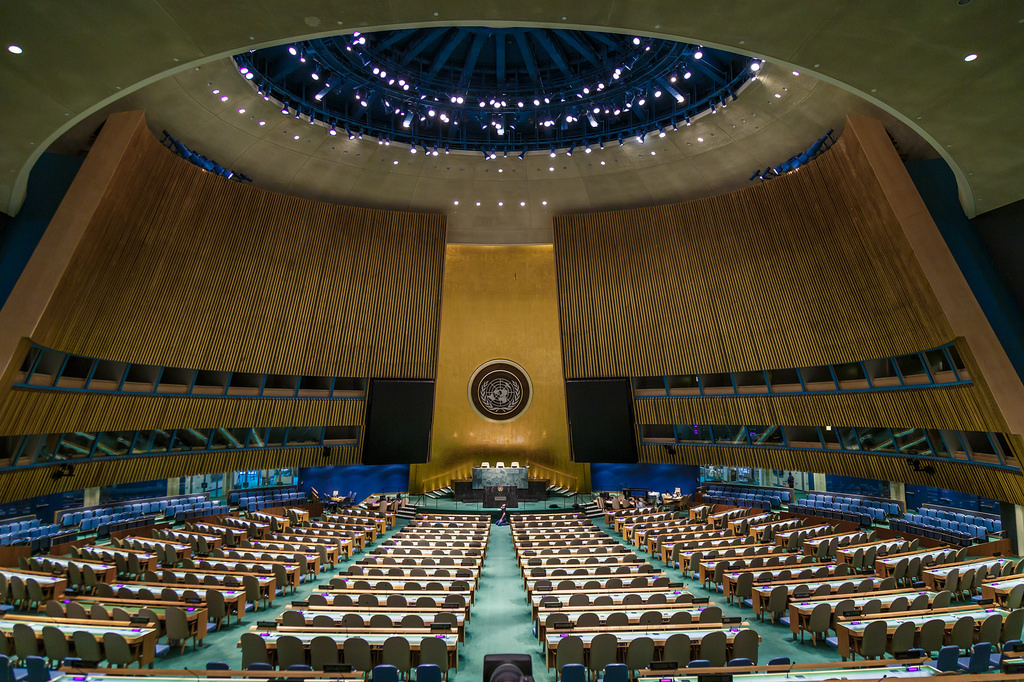
Translation manager
We are looking for a volunteer to coordinate the effort to get the project materials translated into as many languages as possible.
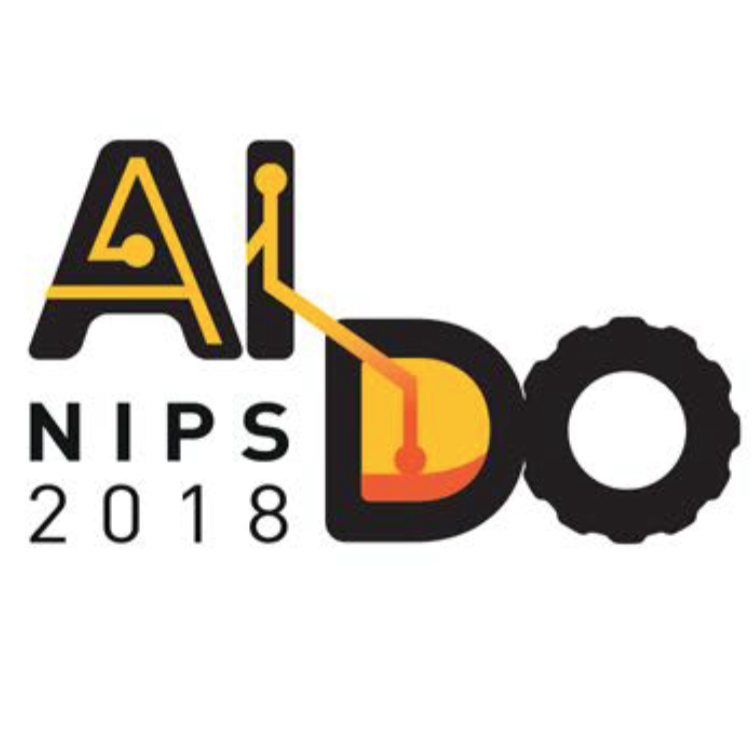
Announcing the AI Driving Olympics (AI-DO)
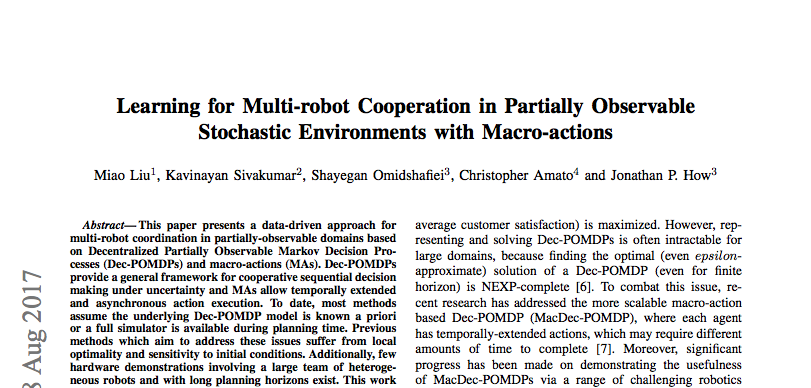
Learning for Multi-robot Cooperation in Partially Observable Stochastic Environments with Macro-actions
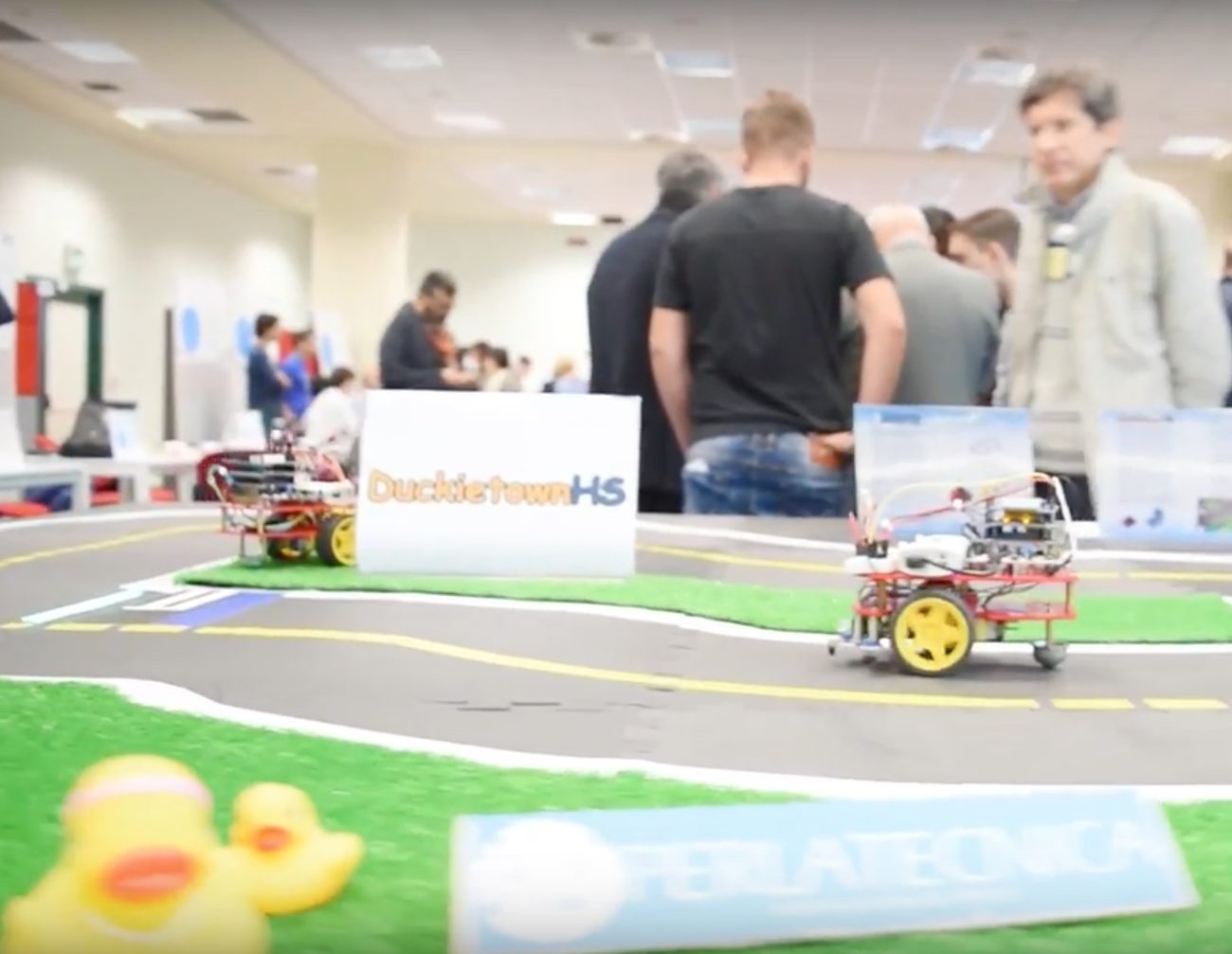
Student Day at ST Napoli
High school students from around Naples enjoyed Duckietown at STMicroelectronics Research Centre this past May 9th.
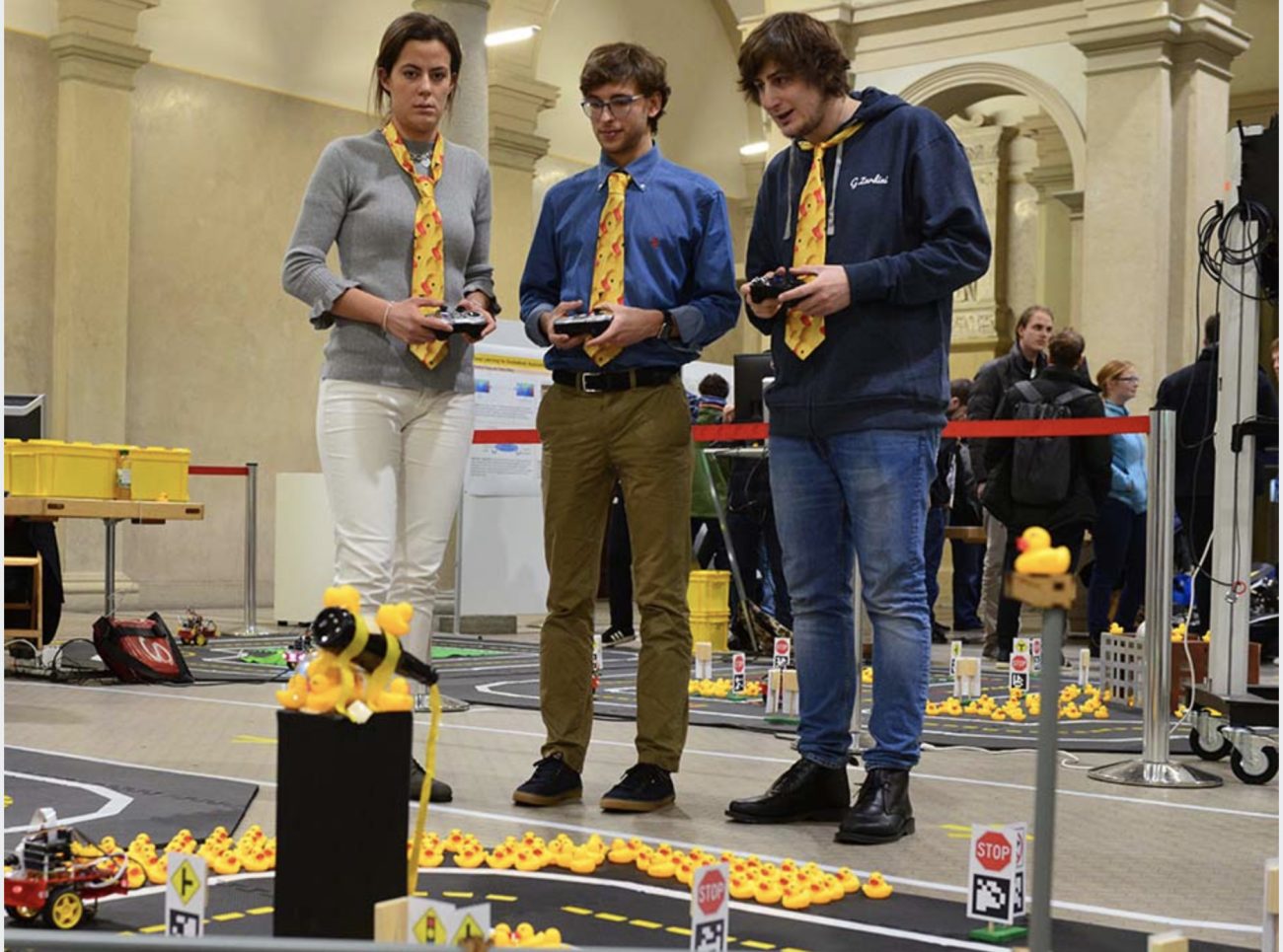
A lab for self-driving vehicles
A lab for self-driving vehicles: profile of the Fall 2017 class.
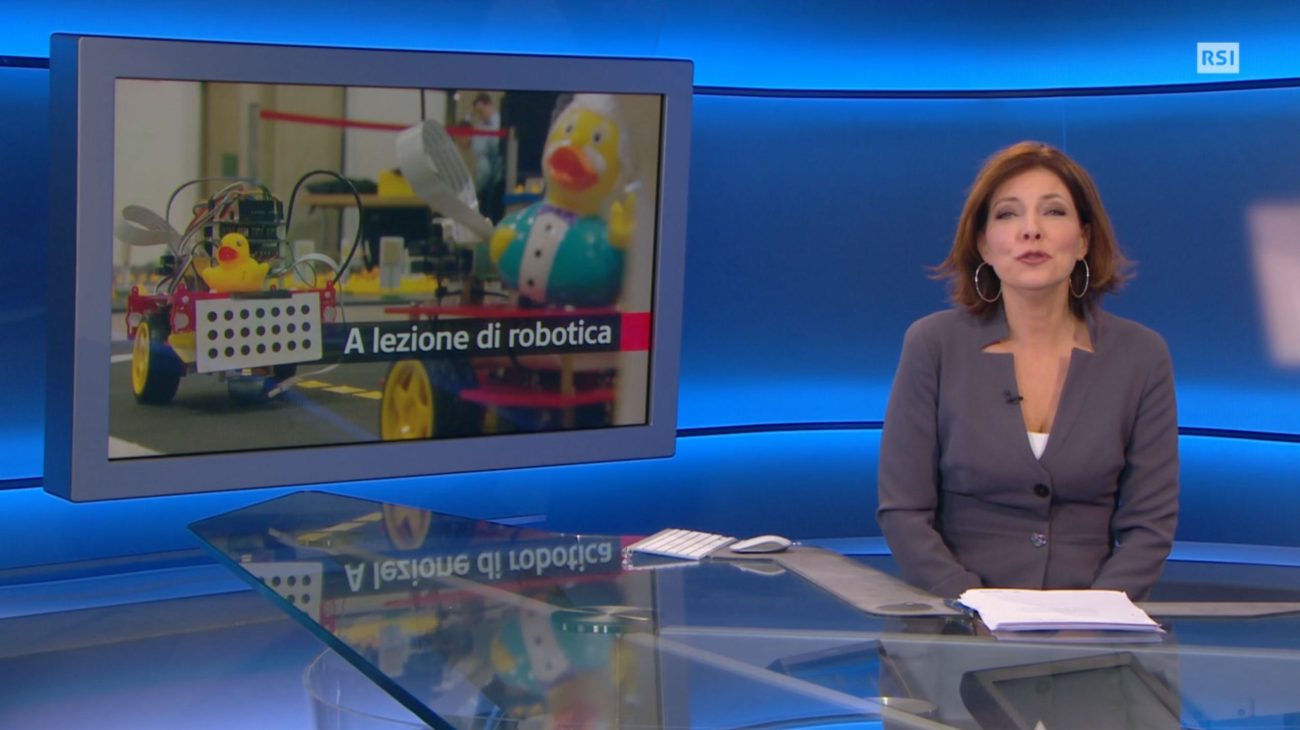