AI-DO 5 Update
AI-DO 5 is in full swing and we want to bring you some updates: better graphics, more maps, faster and more reliable backend and an improved GUI to submit to challenges!
Challenges visualization
We updated the visualization. Now the evaluation produces videos with your name and evaluation number (as below).
Challenges updates
We fixed some of the bugs in the simulator regarding the visualization (“phantom robots” popping in and out).
We updated the maps in the challenges to have more variety in the road network; we put more grass and trees to make the maps more joyful!
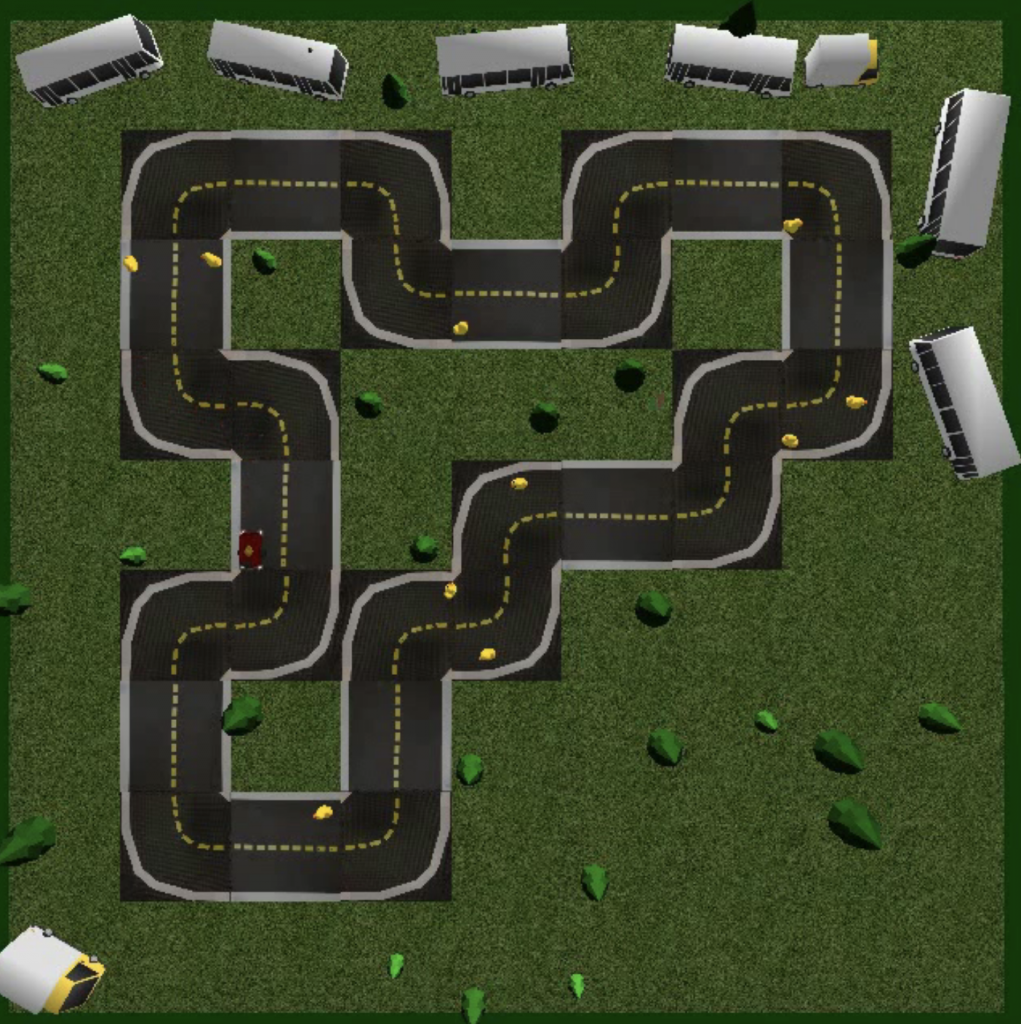
Faster and more reliable backend
The server was getting slow given the number of submissions, and sometime the service was unavailable. We have revamped the server code and added some backend capacity to be more fault-tolerant. It is now much faster!
Thanks so much to the participants that helped us debug this problem!
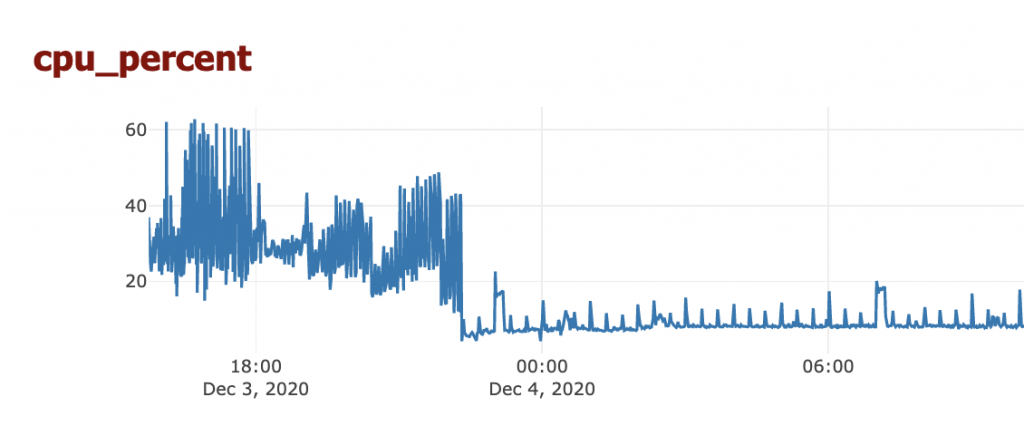
More evaluators
We brought online many more CPU and GPU evaluators. We now encourage you to submit more often as we have a lot more capacity.
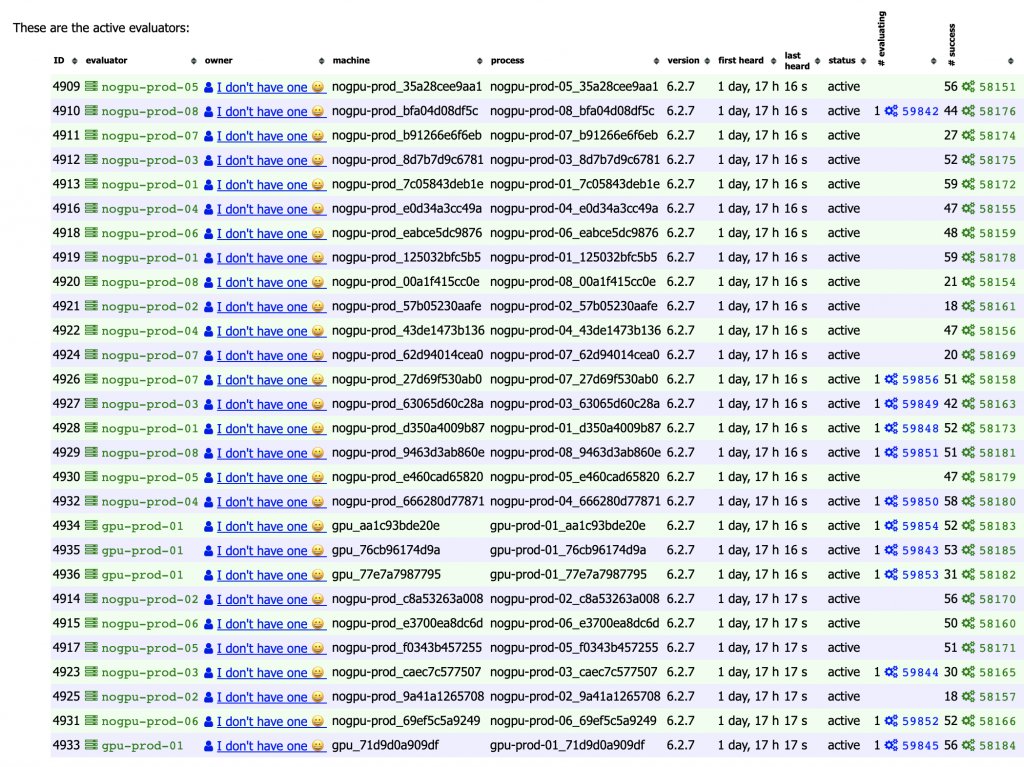
Submit to testing challenges
We also remind you that the challenges on the front page are the validation challenges, in which everybody can see the output. However what counts for winning are the testing challenges!
To do that you can use dts challenges submit with the –challenges option
Or, you can use a new way using the website that we just implemented, described below.
Submitting to other challenges
Step 1: Go to your user page, by clicking “login” and then going to “My Submissions”.

Step 2: In this page you will find your submissions grouped by “component”.
Click the component icon as in the figure.
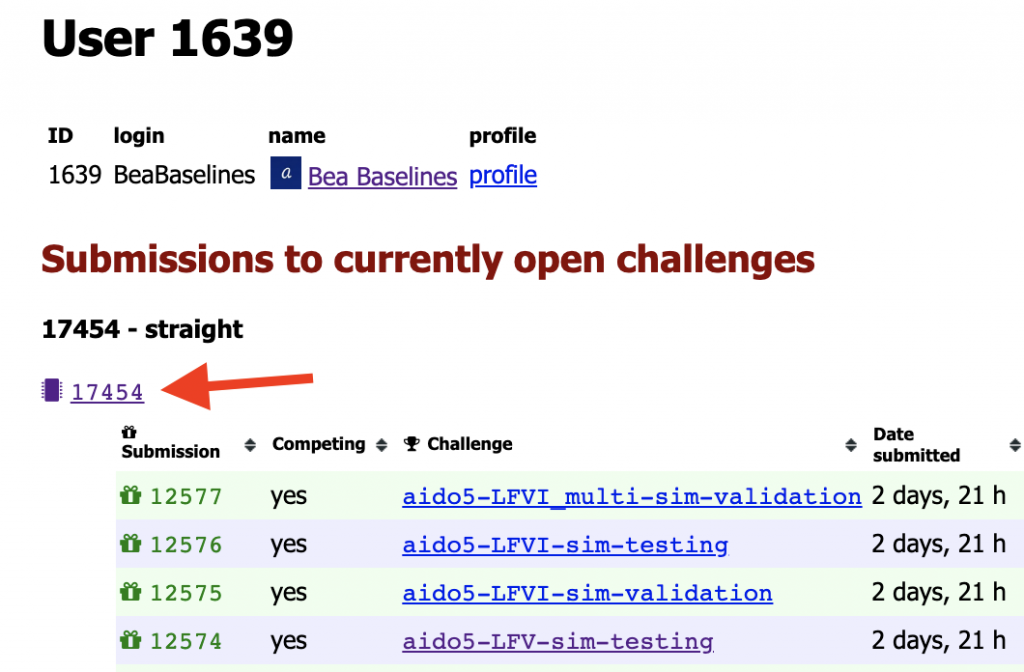
Step 3: The page will contain some buttons that allow you to submit to other challenges that you didn’t submit to yet.
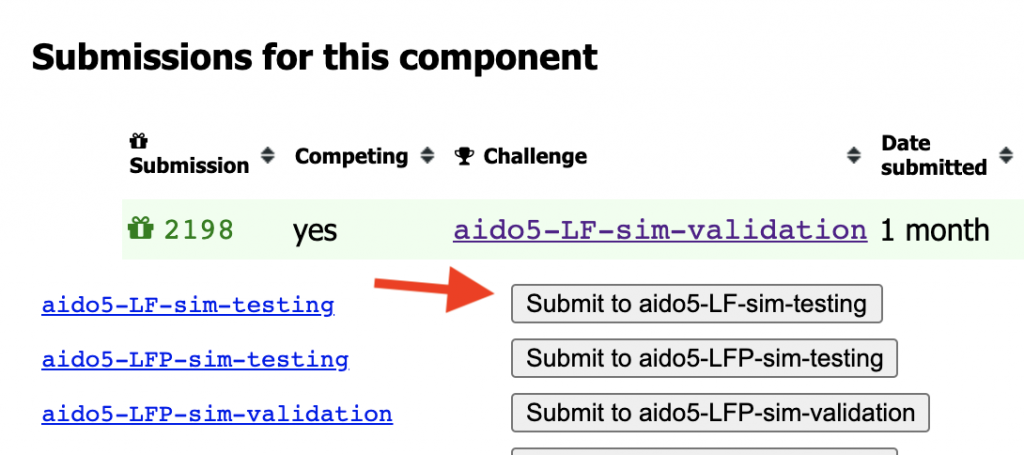